Predicting the future of the economy is a complex yet crucial endeavor. Economic forecasting, the art and science of anticipating economic trends, plays a pivotal role in shaping governmental policies, informing business strategies, and guiding individual financial decisions. From the qualitative insights of expert panels to the quantitative precision of econometric models, a vast array of methods exists, each with its own strengths and limitations. This exploration delves into the core techniques, highlighting their applications and inherent challenges in navigating the unpredictable landscape of economic activity.
This guide systematically examines various economic forecasting methodologies, ranging from qualitative approaches that leverage expert judgment to quantitative methods relying on statistical analysis and econometric modeling. We will explore the historical context, discuss the strengths and weaknesses of different techniques, and analyze their applications in forecasting key economic variables like inflation, unemployment, and GDP growth. Furthermore, we will consider the impact of emerging technologies and the persistent challenges in achieving accurate predictions.
Introduction to Economic Forecasting
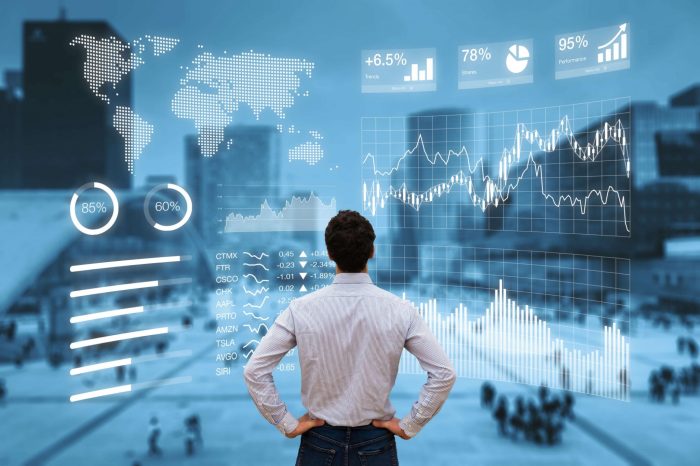
Economic forecasting involves predicting future economic conditions using various statistical and analytical methods. Its significance lies in enabling businesses, governments, and individuals to make informed decisions based on anticipated economic trends, mitigating risks, and capitalizing on opportunities. Accurate forecasting can significantly impact investment strategies, policy formulation, and resource allocation.
Economic forecasting plays a crucial role across diverse sectors. For example, businesses utilize forecasts to optimize production levels, manage inventory, and make strategic investment decisions. Governments rely on economic forecasts to design fiscal and monetary policies, aiming to achieve macroeconomic stability and sustainable growth. Financial institutions use forecasts to assess investment risks, manage portfolios, and develop appropriate financial strategies. Furthermore, individuals may use forecasts to inform major financial decisions such as purchasing a home or investing in the stock market.
The Historical Evolution of Economic Forecasting Methods
Early economic forecasting methods were largely qualitative, relying on expert judgment and anecdotal evidence. The development of econometrics in the 20th century revolutionized the field, introducing quantitative techniques such as time series analysis and regression modeling. The advent of high-speed computing and the availability of vast datasets further enhanced the sophistication of forecasting models. The development of more complex models like Vector Autoregression (VAR) and dynamic stochastic general equilibrium (DSGE) models reflect this ongoing evolution. The increasing use of artificial intelligence and machine learning techniques in recent years represents the latest frontier in economic forecasting. For example, the use of ARIMA models became popular in the 1970s for short-term forecasting, while the development of DSGE models in the 1990s provided a more structural approach to modeling macroeconomic phenomena. The increased availability of real-time data, coupled with advancements in computational power, continues to fuel innovation in this field.
Comparison of Qualitative and Quantitative Forecasting Approaches
The choice between qualitative and quantitative methods depends on the specific forecasting problem, the availability of data, and the desired level of accuracy. Qualitative methods are particularly useful when historical data is scarce or unreliable, while quantitative methods are better suited for situations with abundant and reliable data.
Feature | Qualitative Forecasting | Quantitative Forecasting |
---|---|---|
Data Requirements | Limited historical data; relies on expert judgment and intuition. | Requires substantial historical data; relies on statistical analysis. |
Method | Expert opinions, surveys, Delphi method, scenario planning. | Time series analysis, regression models, econometric models, machine learning algorithms. |
Strengths | Incorporates expert knowledge and insights; useful when historical data is limited or unreliable; can capture qualitative factors. | Objective and statistically rigorous; can identify patterns and trends; allows for quantification of uncertainty. |
Weaknesses | Subjective and prone to bias; difficult to quantify uncertainty; less precise than quantitative methods. | Requires substantial data; may not capture qualitative factors; can be complex and computationally intensive; assumptions underlying the model may not always hold. |
Qualitative Forecasting Methods
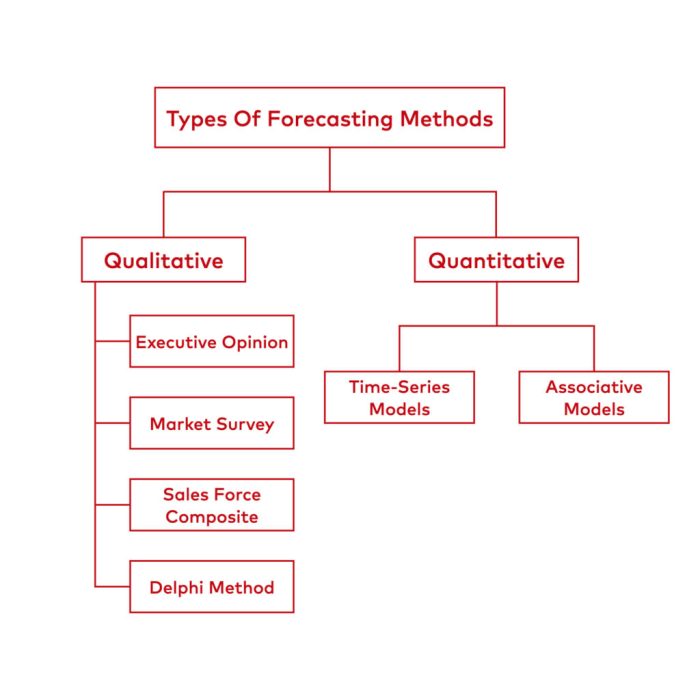
Qualitative forecasting methods rely on expert judgment and subjective assessments rather than purely quantitative data. These methods are particularly valuable when historical data is scarce, unreliable, or irrelevant to the future scenario being considered. They offer a valuable counterpoint to quantitative approaches, providing insights into potential future developments that may not be captured by statistical models.
The Delphi Method
The Delphi method is a structured communication technique used to gather and refine expert opinions on a particular topic. It involves a series of questionnaires distributed to a panel of experts, with feedback provided after each round. This iterative process aims to reach a consensus, or at least a better-informed understanding, of the issue at hand. In economic forecasting, the Delphi method can be used to assess the likelihood of major economic shifts, predict the impact of policy changes, or gauge future consumer behavior. For example, a Delphi panel could be assembled to forecast the long-term effects of a significant technological advancement on a specific industry, incorporating diverse perspectives to create a more robust prediction than any single expert could provide. The iterative nature of the method allows for the refinement of initial judgments based on the considered opinions of others, leading to a more nuanced and informed collective forecast.
Advantages and Limitations of Expert Opinions
Using expert opinions in economic forecasting offers several advantages. Experts possess specialized knowledge and experience that can be invaluable in interpreting complex economic situations. Their insights can identify potential risks and opportunities that may be overlooked by purely quantitative models. However, expert opinions are also subject to biases, and individual experts may hold strongly divergent views. The Delphi method helps mitigate some of these issues by incorporating feedback and encouraging reasoned discussion, but it cannot entirely eliminate the possibility of subjective biases influencing the final forecast. Over-reliance on a limited number of experts, or experts with similar backgrounds, can also limit the breadth and depth of the analysis.
Scenarios Where Qualitative Methods Excel
Qualitative forecasting methods are particularly useful in situations characterized by high uncertainty and a lack of historical data. For instance, predicting the economic impact of a completely novel technology, such as the initial development of the internet, would have been challenging using purely quantitative techniques. Similarly, forecasting the economic consequences of a major geopolitical event, such as a global pandemic or a significant international conflict, often necessitates incorporating expert judgment and qualitative assessments alongside quantitative data. In these scenarios, the ability of qualitative methods to capture the nuanced complexities of human behavior and political dynamics makes them an indispensable tool. Furthermore, when forecasting for emerging markets with limited data availability, qualitative methods can provide valuable insights that quantitative approaches might miss.
Qualitative Forecasting Techniques
The effectiveness of qualitative forecasting hinges on the selection and application of appropriate techniques. A well-chosen method enhances the accuracy and relevance of the forecast.
- Delphi Method: As previously described, this structured approach iteratively gathers and refines expert opinions.
- Scenario Planning: This technique involves developing multiple plausible future scenarios based on different assumptions and uncertainties.
- Market Research: Gathering data directly from consumers or businesses through surveys, focus groups, or interviews to understand their intentions and preferences.
- Expert Panels/Roundtables: Bringing together experts to discuss and debate potential future developments.
- Intuitive Forecasting: Relies on the judgment and experience of a single individual or a small group with deep understanding of the area.
Quantitative Forecasting Methods
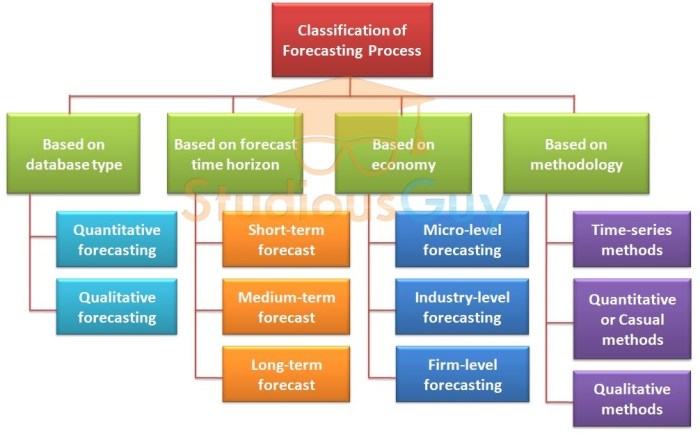
Quantitative forecasting methods rely on mathematical and statistical models to predict future economic trends. Unlike qualitative methods, which rely on expert opinion and judgment, quantitative approaches leverage historical data and established relationships to generate forecasts. This allows for a more objective and potentially more accurate prediction, though the accuracy is heavily dependent on the quality of the data and the appropriateness of the chosen model.
Time Series Analysis and its Components
Time series analysis is a crucial quantitative forecasting technique that examines data points collected over time. Understanding the components of a time series is fundamental to building accurate models. These components include trend, seasonality, and cyclical fluctuations. The trend represents the long-term direction of the data, either upward, downward, or stable. Seasonality refers to recurring patterns within a fixed period, such as yearly or monthly fluctuations. Cyclical fluctuations represent longer-term, less predictable ups and downs in the data, often lasting several years and not following a fixed pattern. For example, a time series of quarterly GDP might show an upward trend over several decades, seasonal peaks in the third quarter, and cyclical fluctuations related to business cycles. Successfully isolating and modeling these components is key to creating effective forecasts.
Comparison of Time Series Models: ARIMA and Exponential Smoothing
ARIMA (Autoregressive Integrated Moving Average) and Exponential Smoothing are two widely used time series models. ARIMA models use past values of the variable and past forecast errors to predict future values. The parameters (p, d, q) specify the order of the autoregressive (AR), integrated (I), and moving average (MA) components. Exponential smoothing assigns exponentially decreasing weights to older observations, giving more weight to recent data. Simple exponential smoothing is suitable for data with a stable trend, while more sophisticated variants like Holt-Winters handle trends and seasonality. The choice between ARIMA and exponential smoothing depends on the characteristics of the data and the forecasting horizon. ARIMA models can be more powerful for complex patterns, while exponential smoothing is often simpler to implement and understand. For instance, forecasting daily stock prices might benefit from ARIMA’s ability to capture complex short-term fluctuations, while forecasting yearly sales might be better suited to exponential smoothing’s simplicity and robustness to noise.
Assumptions Underlying Econometric Modeling
Econometric modeling employs statistical techniques to estimate relationships between economic variables. Several key assumptions underpin the reliability of econometric models. These include linearity (the relationship between variables is linear), independence (observations are independent of each other), homoscedasticity (the variance of the error term is constant), and no multicollinearity (independent variables are not highly correlated). Violation of these assumptions can lead to biased and inefficient estimates. For example, if the relationship between GDP growth and inflation is non-linear, a linear econometric model will not accurately capture the true relationship.
Leading Indicators in Economic Forecasting
Leading indicators are economic variables that tend to change before changes in the overall economy. They provide valuable insights into future economic activity. Examples include consumer confidence, building permits, and manufacturing orders. By monitoring these indicators, economists can anticipate turning points in the business cycle and adjust forecasts accordingly. For instance, a sharp decline in consumer confidence might signal an impending recession, allowing businesses to adjust their production plans and investment strategies.
Regression Analysis in Economic Forecasting: A Hypothetical Example
Regression analysis is a statistical method used to model the relationship between a dependent variable and one or more independent variables. In economic forecasting, it can be used to predict a variable of interest based on other related variables. For example, let’s say we want to predict housing prices (dependent variable) based on factors like average household income, interest rates, and number of new construction permits (independent variables). We could collect historical data on these variables and use regression analysis to estimate the coefficients of a model of the form:
Housing Price = β0 + β1(Household Income) + β2(Interest Rates) + β3(New Construction Permits) + ε
where β0 is the intercept, β1, β2, and β3 are the coefficients representing the effect of each independent variable, and ε is the error term. The estimated coefficients would allow us to predict future housing prices based on projected values of the independent variables. A positive coefficient for household income, for example, would suggest that higher incomes are associated with higher housing prices. This model could be refined further by incorporating other relevant factors and using more advanced regression techniques.
Econometric Models
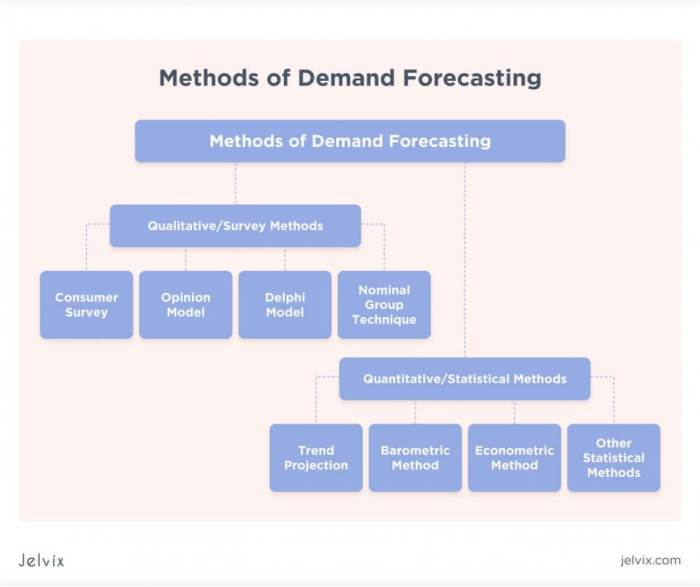
Econometric models are statistical models used to analyze economic data and forecast future economic trends. They provide a framework for testing economic theories and quantifying the relationships between economic variables. Unlike purely qualitative methods, econometric models offer a rigorous, data-driven approach to understanding economic phenomena. These models are crucial for policymakers, businesses, and researchers seeking to make informed decisions based on evidence.
Structural Econometric Models
Structural econometric models represent the underlying economic relationships between variables. These models are built based on established economic theories, specifying the causal links between variables. For example, a structural model of the labor market might include equations representing wage determination, labor supply, and labor demand. The parameters of these equations are then estimated using statistical techniques, allowing for the analysis of how changes in one variable (e.g., minimum wage) affect others (e.g., employment and wages). The strength of structural models lies in their ability to provide insights into the mechanisms driving economic behavior. However, they often require strong assumptions about the data generating process and can be challenging to estimate due to the complexity of economic systems.
Econometric Model Building, Estimation, and Validation
The process of building, estimating, and validating an econometric model involves several key steps. First, the model is specified based on economic theory and available data. This includes defining the variables, their relationships, and the functional form of the model. Next, the model parameters are estimated using statistical methods, such as ordinary least squares (OLS) or maximum likelihood estimation (MLE). The choice of estimation method depends on the model’s characteristics and the properties of the data. Finally, the estimated model is validated to assess its goodness of fit, predictive accuracy, and robustness. Diagnostic tests are performed to check for violations of model assumptions, such as heteroskedasticity or autocorrelation. If the model fails to meet these criteria, it may need to be revised or respecified.
Challenges in Econometric Modeling
Econometric modeling is not without its challenges. Two significant issues are endogeneity and multicollinearity. Endogeneity arises when an variable is correlated with the error term, leading to biased and inconsistent parameter estimates. This often occurs when omitted variables are correlated with included variables or there is simultaneity (where variables mutually influence each other). Multicollinearity occurs when two or more variables are highly correlated, making it difficult to isolate their individual effects on the dependent variable. This can lead to unstable and imprecise parameter estimates. Addressing these issues requires careful model specification, the use of instrumental variables, or other advanced econometric techniques.
Examples of Econometric Models in Macroeconomic Forecasting
Various econometric models are employed in macroeconomic forecasting. These include:
- Autoregressive (AR) models: These models predict a variable based on its past values. For example, an AR(1) model for GDP growth would predict next quarter’s growth based on this quarter’s growth.
- Vector Autoregression (VAR) models: VAR models extend AR models to multiple variables, allowing for the analysis of their interdependencies. A VAR model could be used to forecast inflation and unemployment simultaneously, capturing their dynamic interactions.
- Dynamic Stochastic General Equilibrium (DSGE) models: These are complex models based on microeconomic foundations, simulating the interactions of various economic agents and markets. DSGE models are often used by central banks for policy analysis and forecasting.
Comparison of Econometric Model Types
Model Type | Description | Applications | Advantages |
---|---|---|---|
AR | Predicts a variable based on its past values. | Forecasting GDP growth, inflation. | Simple, easy to estimate. |
VAR | Models the interdependencies of multiple variables. | Forecasting multiple macroeconomic variables simultaneously (e.g., inflation, unemployment, GDP). | Captures dynamic interactions between variables. |
DSGE | Based on microeconomic foundations, simulating interactions of economic agents and markets. | Policy analysis, forecasting macroeconomic aggregates, understanding economic shocks. | Provides a structural understanding of the economy. |
Structural Equation Modeling (SEM) | Represents complex relationships between multiple variables, including latent variables. | Analyzing complex economic systems, testing economic theories. | Handles latent variables and complex relationships. |
Forecasting Specific Economic Variables
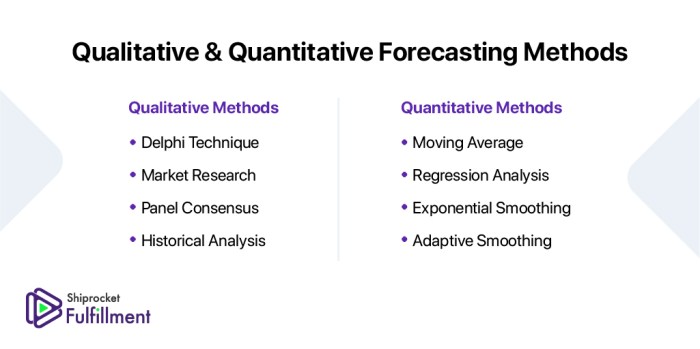
Accurately forecasting key economic variables is crucial for effective policymaking and informed business decisions. Different methodologies are employed depending on the variable being predicted, and the accuracy of these forecasts varies depending on the complexity of the economic system and the availability of reliable data. This section will delve into the methods used to forecast inflation, unemployment, and GDP growth, highlighting their applications in policy formulation.
Inflation Forecasting Methodologies
Inflation forecasting involves predicting the rate at which the general level of prices for goods and services is rising, and a variety of methods are used. These range from simple time-series models, which extrapolate past trends, to more complex econometric models that incorporate various economic indicators and relationships. A common approach involves using the Consumer Price Index (CPI) or Producer Price Index (PPI) as a basis for prediction. For example, a model might incorporate past inflation rates, current levels of unemployment, and expected changes in commodity prices to project future inflation. Central banks often use sophisticated models incorporating factors like monetary policy, exchange rates, and supply chain disruptions to inform their interest rate decisions. These models may also include forecasts of future energy prices and wage growth as significant drivers of inflation.
Unemployment Rate Forecasting Methods
Forecasting unemployment rates typically involves analyzing labor market dynamics and economic activity. Time-series analysis, using historical unemployment data to identify trends and patterns, is a fundamental approach. However, this is often supplemented with leading indicators like job openings, hiring intentions, and consumer confidence indices. Econometric models can be constructed to incorporate relationships between unemployment, GDP growth, and other economic variables. For instance, an increase in GDP growth is often associated with a decrease in unemployment, a relationship captured in Okun’s Law. The accuracy of unemployment forecasts can be significantly affected by unforeseen shocks, such as a global pandemic or a major technological disruption. For example, the COVID-19 pandemic led to unexpectedly high unemployment rates, highlighting the limitations of even sophisticated forecasting models in the face of unprecedented events.
Gross Domestic Product (GDP) Growth Forecasting Approaches
GDP growth forecasting relies on a combination of top-down and bottom-up approaches. Top-down methods use macroeconomic indicators like consumption, investment, government spending, and net exports to estimate overall GDP growth. Bottom-up approaches aggregate forecasts of individual sectors’ output to arrive at a national GDP estimate. Econometric models are frequently used, often incorporating leading indicators like consumer confidence, business investment plans, and housing starts. These models can be quite complex, incorporating various lags and feedback loops between different economic variables. For example, a model might predict GDP growth based on forecasts of consumer spending, business investment, and government spending, taking into account potential multiplier effects. The International Monetary Fund (IMF) and other international organizations produce regular GDP growth forecasts for various countries, often using sophisticated models that integrate global economic conditions.
Examples of Forecasts in Policy-Making
Economic forecasts are fundamental to policy decisions at both the national and international levels. Central banks use inflation and unemployment forecasts to set monetary policy, adjusting interest rates to manage inflation and promote full employment. Governments use GDP growth forecasts to plan budgets, setting spending levels and tax rates based on projected economic activity. For example, if GDP growth is expected to be strong, the government might increase spending on infrastructure projects or reduce taxes to stimulate further growth. Conversely, during an economic downturn, governments might increase social safety net spending and implement fiscal stimulus measures.
Key Indicators for Economic Variables
The accuracy of economic forecasts depends heavily on the quality and availability of data. Below is a list of key indicators commonly used in forecasting each variable:
- Inflation: Consumer Price Index (CPI), Producer Price Index (PPI), inflation expectations, commodity prices, wage growth, exchange rates.
- Unemployment Rate: Unemployment rate, labor force participation rate, job openings, hiring intentions, consumer confidence index, quits rate.
- GDP Growth: Real GDP growth, consumer spending, business investment, government spending, net exports, inventory investment, housing starts, consumer confidence index.
Challenges and Limitations in Economic Forecasting
Economic forecasting, while a crucial tool for policymakers and businesses, is inherently imperfect. Numerous factors contribute to inaccuracies and limitations, making it essential to understand these challenges to interpret forecasts effectively and make informed decisions. The inherent complexity of economic systems, coupled with the unpredictable nature of human behavior and global events, makes perfect prediction impossible.
Sources of Uncertainty and Error in Economic Forecasts
Several sources contribute to the uncertainty and error inherent in economic forecasts. These include limitations in data availability and quality, the inherent complexity of economic models, and the unpredictable nature of external shocks. For example, incomplete or inaccurate data on employment, inflation, or consumer spending can significantly skew forecast results. Furthermore, even sophisticated econometric models rely on assumptions that may not always hold true in the real world. Finally, unexpected events, such as natural disasters or geopolitical upheavals, can dramatically alter economic trajectories, rendering existing forecasts obsolete. The inherent difficulty in predicting human behavior, including consumer confidence and investment decisions, further compounds these challenges.
Impact of Unforeseen Events on Forecasting Accuracy
Unforeseen events, by their very nature, represent a significant challenge to accurate economic forecasting. The COVID-19 pandemic, for instance, demonstrated the fragility of even the most sophisticated models. Forecasts made prior to the pandemic dramatically underestimated the severity of the economic downturn, highlighting the limitations of relying solely on historical data and established patterns. Similarly, the outbreak of war in Ukraine in 2022 had unforeseen consequences for global energy prices and supply chains, significantly impacting economic forecasts across various sectors. These events underscore the need for models that incorporate a degree of flexibility and the ability to adapt to rapidly changing circumstances.
Limitations of Using Historical Data for Future Predictions
While historical data forms the foundation of most economic forecasting methods, relying solely on past trends for future predictions has inherent limitations. Structural changes in the economy, such as technological advancements or shifts in global trade patterns, can render historical data less relevant. For example, the rapid growth of the internet and e-commerce significantly altered consumer behavior and retail landscapes, making predictions based solely on pre-internet data unreliable. Furthermore, the emergence of new economic policies or regulatory changes can also dramatically alter the economic landscape, making past trends a poor indicator of future performance. Extrapolating past trends without accounting for these structural breaks can lead to significant forecast errors.
Strategies for Improving the Accuracy of Economic Forecasts
Improving the accuracy of economic forecasts requires a multi-faceted approach. This involves refining data collection methods to ensure data accuracy and completeness, enhancing the sophistication of econometric models to account for non-linear relationships and structural breaks, and incorporating qualitative information from expert surveys and market sentiment indicators. Furthermore, employing scenario planning techniques to anticipate a range of possible outcomes, rather than relying on a single point forecast, can improve the robustness of predictions. Finally, regular evaluation and revision of forecasting models based on actual outcomes are crucial for continuous improvement.
Evaluating Forecast Accuracy: A Flowchart
A flowchart for evaluating forecast accuracy would begin with the collection of actual economic data. This data is then compared to the previously generated forecast. The difference between the actual and forecasted values represents the forecast error. This error is then analyzed using metrics such as Mean Absolute Error (MAE), Root Mean Squared Error (RMSE), or Mean Absolute Percentage Error (MAPE). These metrics quantify the magnitude of the forecast error. Based on the analysis of these metrics, the forecasting model is evaluated for accuracy. If the accuracy is deemed unsatisfactory, adjustments are made to the model, potentially including improvements in data collection, model specification, or the incorporation of new variables. The revised model is then used to generate a new forecast, and the process repeats iteratively. The flowchart would visually represent this cyclical process of forecasting, error analysis, and model refinement.
Future Trends in Economic Forecasting
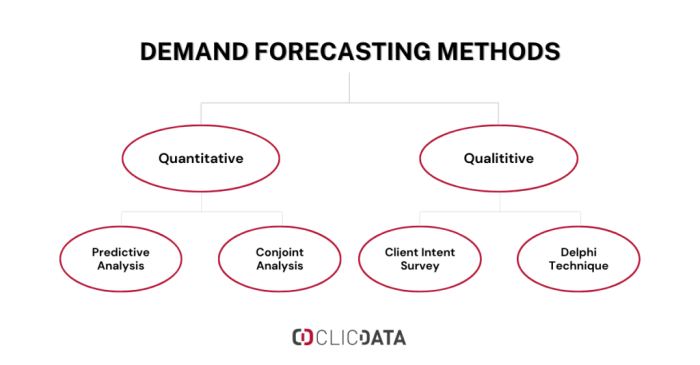
Economic forecasting is undergoing a rapid transformation, driven by advancements in data science, artificial intelligence, and a heightened awareness of global challenges like climate change. These trends are not merely incremental improvements; they represent a fundamental shift in how we approach predicting future economic conditions. The integration of sophisticated technologies and a more nuanced understanding of systemic risks are reshaping the field, leading to more accurate, robust, and insightful forecasts.
The increasing availability of data and the development of powerful analytical tools are significantly enhancing the accuracy and sophistication of economic forecasts. This section will explore some of the key future trends shaping this evolution.
The Role of Big Data and Machine Learning in Improving Forecasting Accuracy
Big data, encompassing vast and varied datasets from diverse sources, offers unprecedented opportunities for economic forecasting. Machine learning algorithms, capable of identifying complex patterns and relationships within these datasets, are proving particularly effective. For instance, machine learning models can analyze transactional data from millions of credit card purchases to predict consumer spending patterns with greater accuracy than traditional methods. Furthermore, the ability of these algorithms to handle unstructured data, such as social media sentiment or news articles, allows for the incorporation of qualitative factors that were previously difficult to quantify. The combination of big data and machine learning leads to more granular, real-time insights into economic activity, improving the timeliness and accuracy of forecasts.
The Application of Artificial Intelligence in Economic Forecasting
Artificial intelligence (AI) is extending the capabilities of machine learning, enabling the development of more sophisticated and adaptive forecasting models. AI-powered systems can not only identify patterns but also learn and adapt to changing economic conditions in real-time. For example, AI algorithms can be used to create dynamic models that adjust their parameters based on new information, providing more accurate predictions in volatile economic environments. Furthermore, AI can automate many of the tasks involved in economic forecasting, such as data collection, cleaning, and model building, freeing up economists to focus on higher-level analysis and interpretation. The use of AI in economic forecasting is still in its early stages, but its potential to revolutionize the field is undeniable.
The Potential Impact of Climate Change on Economic Forecasting
Climate change presents significant challenges for economic forecasting. Extreme weather events, rising sea levels, and shifts in agricultural productivity can all have profound impacts on economic activity. Integrating climate-related risks into economic models is crucial for developing accurate and robust forecasts. This requires incorporating climate projections from scientific models into economic scenarios, assessing the potential economic impacts of various climate change pathways, and developing strategies for adapting to and mitigating these impacts. For example, a model might predict decreased agricultural output in certain regions due to drought, leading to higher food prices and impacting overall economic growth. This integration is vital for making informed policy decisions and preparing for future economic challenges.
The Growing Importance of Scenario Planning in Economic Forecasting
Scenario planning is becoming increasingly important as the global economy faces growing uncertainty. This approach involves developing multiple plausible scenarios for the future, each based on different assumptions about key drivers of economic change. By considering a range of possibilities, rather than relying on a single point forecast, scenario planning allows for a more robust and nuanced understanding of potential risks and opportunities. For instance, an economic forecast might include scenarios for a global pandemic, a major geopolitical event, or a significant technological disruption, enabling policymakers and businesses to prepare for a wider range of outcomes. This approach is particularly valuable in navigating the complexity of interconnected global systems.
The Potential Impact of Advancements in Technology on Economic Forecasting Methods
Advancements in computing power, data storage, and communication technologies are fundamentally changing the landscape of economic forecasting. The ability to process and analyze vast datasets in real-time allows for the development of more sophisticated models that incorporate a wider range of variables and capture more complex relationships. Cloud computing enables collaborative forecasting efforts, allowing researchers to share data and models more easily. Furthermore, advancements in visualization tools allow for a more intuitive and effective communication of forecast results to policymakers and the public. For example, the development of high-performance computing allows for the simulation of complex economic systems, providing a more accurate representation of the interconnectedness of various economic factors. This leads to more robust and comprehensive forecasts.
Final Wrap-Up
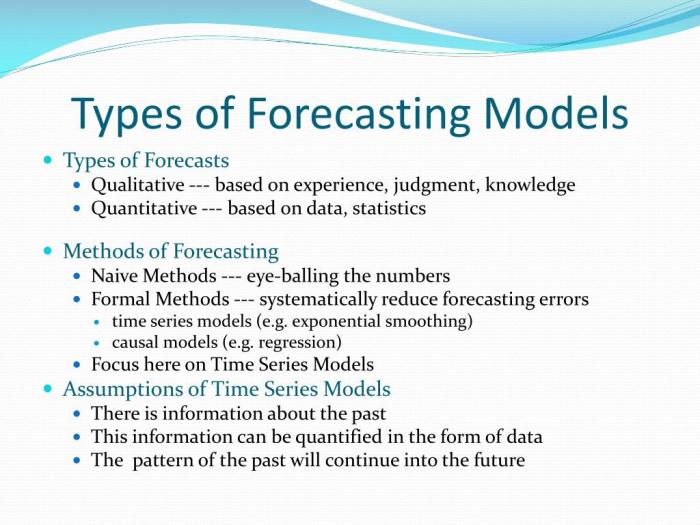
Mastering economic forecasting is not about achieving perfect prediction, but rather about understanding the inherent uncertainties and employing the most appropriate tools to inform decision-making. While technological advancements and sophisticated models continue to refine the process, the human element—the interpretation of data and the incorporation of qualitative insights—remains indispensable. By understanding the strengths and limitations of different approaches, policymakers, businesses, and individuals can better navigate the complexities of the economic landscape and make more informed choices.
Essential Questionnaire
What is the difference between leading, lagging, and coincident indicators?
Leading indicators precede economic changes, lagging indicators follow them, and coincident indicators occur simultaneously with economic shifts.
How do unforeseen events affect forecast accuracy?
Unforeseen events like pandemics or geopolitical crises introduce significant uncertainty and can drastically reduce forecast accuracy, requiring model adjustments and scenario planning.
What role does data quality play in economic forecasting?
Data quality is paramount. Inaccurate or incomplete data leads to flawed models and unreliable forecasts. Robust data collection and validation are essential.
Can economic forecasting predict individual market movements?
No, economic forecasting primarily focuses on aggregate trends and macroeconomic variables, not individual market fluctuations, which are subject to numerous micro-level factors.