Economic Forecasting Methods Review sets the stage for this enthralling narrative, offering readers a glimpse into the fascinating—and sometimes hilariously inaccurate—world of predicting the future of economies. From ancient soothsayers to cutting-edge AI, we’ll explore the evolution of techniques used to peer into the economic crystal ball, examining both the triumphs and the spectacular failures along the way. Prepare for a journey through econometric models, qualitative guesswork, and hybrid approaches that blend the art and science of prediction.
This review delves into the various methods employed to forecast economic trends, exploring both quantitative approaches like ARIMA and VAR models, and qualitative methods such as the Delphi technique. We’ll dissect the strengths and weaknesses of each, comparing their accuracy, applications, and the inevitable limitations inherent in predicting the unpredictable. We’ll even tackle the thorny issue of evaluating forecast accuracy – because let’s face it, knowing *how wrong* you are is half the battle.
Introduction to Economic Forecasting Methods
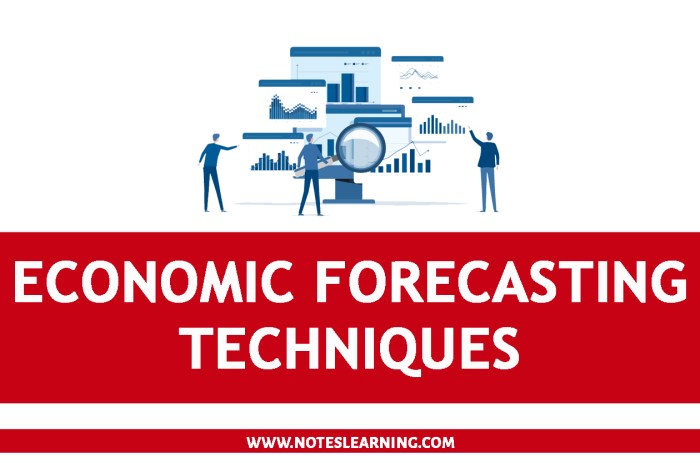
Predicting the future of the economy – a fool’s errand, some might say, yet a crucial task for policymakers, businesses, and even savvy grocery shoppers (who needs to know when to stock up on discounted avocados?). Economic forecasting, the noble (and often hilarious) attempt to peer into the economic crystal ball, has a rich and sometimes slightly embarrassing history.
A Historical Overview of Economic Forecasting Techniques
Early economic forecasting was, let’s be kind, rudimentary. Think crystal balls and perhaps a well-placed tea leaf. The development of econometrics in the early 20th century marked a significant shift. Suddenly, we had statistical methods to analyze economic data – a far cry from relying on the whims of fortune tellers. The post-World War II era saw the rise of large-scale macroeconomic models, behemoths of equations designed to capture the complexities of national economies. These models, while impressive in their ambition, often struggled to accurately predict even relatively straightforward economic events. The development of time series analysis, particularly ARIMA models, provided another significant leap forward, allowing for more sophisticated analysis of economic data over time. More recently, the rise of machine learning and artificial intelligence has opened up exciting new possibilities, though the accuracy of these methods is still being rigorously tested (and sometimes hilariously refuted by actual economic events).
Key Drivers Behind the Development of New Forecasting Methods
The quest for better forecasting methods is driven by several factors. The increasing complexity of global economies demands more sophisticated tools. Unexpected events, like the 2008 financial crisis or the COVID-19 pandemic, constantly highlight the limitations of existing models, spurring the development of more robust and adaptable techniques. The ever-increasing availability of data, thanks to technological advancements, also plays a crucial role, providing richer and more nuanced inputs for forecasting models. Finally, the desire for greater accuracy – and the potential for significant financial gains from accurate predictions – is a powerful motivator. Let’s face it, accurate forecasting can be quite lucrative.
Limitations of Traditional Forecasting Approaches
Traditional forecasting methods, while providing valuable insights, are not without their flaws. Many rely on the assumption of stable relationships between economic variables – an assumption that often proves to be rather optimistic, particularly in times of significant economic upheaval. Furthermore, these models often struggle to incorporate qualitative factors, such as consumer sentiment or geopolitical events, which can significantly influence economic outcomes. The inherent uncertainty surrounding future events means that even the most sophisticated models can produce inaccurate predictions. It’s a bit like trying to predict the weather – sometimes you get it right, sometimes you’re left wondering if you’ve accidentally consulted a particularly unreliable weather app.
Comparison of Forecasting Horizons and Methodologies
Forecasting horizons differ significantly, influencing the choice of appropriate methodologies.
Forecasting Horizon | Time Frame | Typical Methodologies | Example |
---|---|---|---|
Short-Term | Up to 1 year | Time series analysis (ARIMA), leading indicators, surveys | Predicting next quarter’s GDP growth |
Medium-Term | 1-5 years | Econometric models, input-output analysis, scenario planning | Forecasting the impact of a new infrastructure project |
Long-Term | Over 5 years | Long-run growth models, technological forecasting, demographic analysis | Projecting future population growth and its impact on resource demand |
Quantitative Forecasting Methods
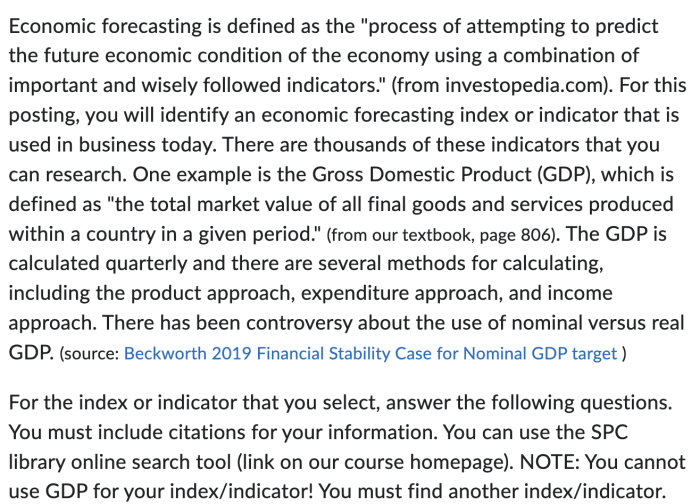
Predicting the future of the economy is a bit like predicting the weather – sometimes you nail it, sometimes you get soaked. Quantitative forecasting methods, however, offer a more structured, data-driven approach than gazing into a crystal ball (though those are fun too!). These methods rely on mathematical and statistical models to analyze historical data and project future trends. It’s less about guesswork and more about rigorous number crunching.
Econometric models are the workhorses of quantitative forecasting. They use statistical techniques to quantify the relationships between economic variables. Imagine them as sophisticated detective work, uncovering the hidden connections between, say, interest rates and consumer spending. This allows us to build models that can predict future values of key economic indicators.
Econometric Model Types: ARIMA and VAR
ARIMA (Autoregressive Integrated Moving Average) models are particularly useful for analyzing time series data – data collected over time, like monthly GDP figures. These models identify patterns and trends within the data to forecast future values. Think of it as a sophisticated algorithm that learns from the past to predict the future, much like a seasoned economist who has seen many economic cycles. VAR (Vector Autoregression) models, on the other hand, are more complex, capable of analyzing multiple time series simultaneously and exploring the interrelationships between them. For example, a VAR model could examine the impact of changes in inflation on unemployment and vice versa. It’s like having multiple detectives working together to solve a complex economic puzzle.
Model Selection and Parameter Estimation
Choosing the right model is crucial – using the wrong tool for the job can lead to inaccurate predictions (and some seriously embarrassing forecasts). Model selection involves assessing various criteria, such as the model’s fit to the historical data and its predictive power. Parameter estimation is the process of determining the numerical values that best represent the relationships between variables within the chosen model. This often involves complex statistical procedures, but the outcome is a refined model ready to make its predictions. Think of this stage as meticulously calibrating your forecasting machine to ensure accuracy. For example, in a regression model predicting housing prices, parameters might represent the influence of factors like square footage, location, and interest rates.
Comparison of Statistical Methods
Regression analysis and time series analysis are two key statistical methods employed in economic forecasting. Regression analysis examines the relationship between a dependent variable (what we want to predict) and one or more independent variables (factors influencing the dependent variable). Time series analysis, as mentioned earlier, focuses on patterns and trends within a single variable over time. The choice between these methods depends on the specific forecasting problem and the nature of the available data. It’s a bit like choosing the right tool from your toolbox – a hammer for one job, a screwdriver for another. For example, predicting future inflation might use time series analysis, while predicting consumer spending might involve regression analysis considering factors like income and consumer confidence.
Hypothetical Scenario: Predicting Retail Sales
Let’s imagine we want to predict retail sales for the next quarter. We could use a simple linear regression model. We’d collect historical data on retail sales, consumer confidence index, and disposable income. After running a regression analysis, we might find that retail sales (our dependent variable) are positively correlated with both consumer confidence and disposable income (our independent variables). The model would then generate an equation, allowing us to plug in projected values for consumer confidence and disposable income for the next quarter to predict the corresponding retail sales. For instance, if our model predicts a 5% increase in consumer confidence and a 3% increase in disposable income, the model might forecast a 4% increase in retail sales, demonstrating the model’s ability to combine different factors to generate a prediction. Naturally, the accuracy of this prediction depends on the model’s reliability and the accuracy of the input data.
Qualitative Forecasting Methods
Predicting the future is a tricky business, especially when dealing with the unpredictable human element. While quantitative methods rely on hard numbers and statistical wizardry, qualitative forecasting embraces the fuzzier, more intuitive side of prediction – think crystal balls, but with slightly more rigor. It’s the art of harnessing human expertise to gaze into the economic mists.
Qualitative forecasting methods rely on expert judgment and subjective opinions rather than solely on numerical data. This approach is particularly useful when dealing with novel situations, emerging technologies, or complex social phenomena where historical data is scarce or unreliable. Think predicting the impact of a groundbreaking new invention or anticipating consumer reactions to a major societal shift – numbers alone just won’t cut it.
Delphi Technique and Expert Surveys
The Delphi technique is like a sophisticated game of telephone, but instead of garbled gossip, you get refined expert opinions. It involves gathering anonymous feedback from a panel of experts through multiple rounds of questionnaires. Each round builds upon the previous one, allowing experts to refine their predictions based on the collective wisdom (or perhaps, collective wisdom-adjacent musings) of their peers. This iterative process aims to achieve a consensus forecast, hopefully minimizing the influence of individual biases and wild guesses. Expert surveys, on the other hand, are a more straightforward approach, directly soliciting opinions from experts through interviews or questionnaires. While less iterative than the Delphi technique, they can still provide valuable insights, especially when time is of the essence (or when your panel of experts are particularly chatty). For example, imagine using the Delphi technique to forecast the long-term impact of artificial intelligence on the job market, gathering input from economists, AI specialists, and sociologists. An expert survey could be used for a quicker assessment of consumer sentiment toward a new product launch.
Strengths and Weaknesses of Qualitative Methods Compared to Quantitative Methods
Qualitative forecasting methods, while charming in their reliance on human intuition, have their limitations. They lack the mathematical precision of quantitative methods and can be highly subjective, leading to potentially biased or unreliable predictions. The reliance on expert opinions also introduces the risk of groupthink and the potential for a single, influential expert to sway the overall forecast. However, these methods shine when dealing with situations where historical data is insufficient or where qualitative factors, such as consumer sentiment or political instability, are significant drivers of economic change. Quantitative methods, while precise, can sometimes miss the nuances of human behavior and unforeseen events. They are like powerful engines, but without a skilled driver, they can be quite dangerous. The ideal approach often involves combining both qualitative and quantitative methods to leverage their respective strengths, creating a more robust and comprehensive forecast.
Comparison of Qualitative Forecasting Methods
The effectiveness of different qualitative methods depends heavily on the specific context and the expertise of the individuals involved. Choosing the right method is crucial for obtaining meaningful results.
- Delphi Technique: Advantages include reduced bias due to anonymity and iterative feedback; Disadvantages include time-consuming nature and potential difficulty in achieving consensus.
- Expert Surveys: Advantages include relative speed and simplicity; Disadvantages include potential for bias from individual experts and limited scope for refining opinions.
- Scenario Planning: Advantages include exploration of multiple potential futures and identification of critical uncertainties; Disadvantages include complexity and potential for overwhelming detail.
- Market Research: Advantages include direct input from potential consumers and identification of emerging trends; Disadvantages include cost and potential for biased sampling.
Hybrid Forecasting Methods: Economic Forecasting Methods Review
The world of economic forecasting isn’t a black-and-white affair; it’s more of a delightfully chaotic blend of numbers and intuition. While purely quantitative or qualitative methods each have their strengths, the truly savvy forecaster recognizes the power of synergy. Hybrid methods, combining the precision of quantitative models with the nuanced insights of qualitative approaches, offer a more robust and often surprisingly accurate predictive power. Think of it as the economic equivalent of a perfectly balanced cocktail – a little bit of this, a little bit of that, and the result is something far greater than the sum of its parts.
Combining quantitative and qualitative methods offers several compelling advantages. Quantitative methods, such as time series analysis or econometric modeling, provide a structured, data-driven approach, offering objectivity and the ability to handle large datasets. However, they can sometimes miss the subtle shifts in consumer sentiment or unforeseen geopolitical events. Qualitative methods, such as expert surveys or Delphi techniques, capture these intangible factors, providing context and insights that purely quantitative models often overlook. The marriage of these two approaches mitigates the weaknesses of each, resulting in a more comprehensive and reliable forecast.
Examples of Hybrid Forecasting Models and Their Applications
Several hybrid models effectively integrate quantitative and qualitative approaches. One example is the combination of ARIMA (Autoregressive Integrated Moving Average) models with expert judgment. ARIMA models, a powerful quantitative technique, can predict future values based on past data. However, incorporating expert opinions can adjust the ARIMA forecast to account for unforeseen events or emerging trends. For instance, a forecast for the housing market might use ARIMA to analyze historical sales data, but then incorporate expert assessments of potential interest rate changes or shifts in government policy. Another example is the use of neural networks, a sophisticated quantitative method, combined with qualitative factors like consumer confidence indices. This approach could be used to predict sales for a new product, leveraging the network’s ability to identify complex patterns in historical data while also considering the impact of consumer sentiment on purchasing decisions. A further example would be the integration of scenario planning (qualitative) with econometric models (quantitative) to explore potential future outcomes in a dynamic environment, like anticipating the impact of climate change on agricultural yields.
Integrating Different Data Sources in a Hybrid Model
Integrating diverse data sources is crucial for successful hybrid forecasting. This involves a careful consideration of data quality, consistency, and relevance. Quantitative data, such as macroeconomic indicators (GDP, inflation), financial data (stock prices, interest rates), and sales figures, should be rigorously cleaned and pre-processed to ensure accuracy. Qualitative data, such as expert opinions, survey results, and news sentiment, requires a structured approach to capture and quantify the information. Techniques like sentiment analysis can convert textual data into numerical scores, allowing for integration with quantitative models. Weighting schemes can then be applied to combine the quantitative and qualitative inputs, reflecting their relative importance in the forecast. For instance, a weighted average could be used, assigning higher weights to more reliable or relevant data sources.
Building a Simple Hybrid Model, Economic Forecasting Methods Review
Let’s consider a simplified example of predicting quarterly sales for a hypothetical coffee shop. We’ll use a simple linear regression (quantitative) model based on past sales data and combine it with expert opinion (qualitative) regarding upcoming marketing campaigns.
First, we collect historical quarterly sales data for the past two years. We then build a linear regression model, using time as the independent variable and sales as the dependent variable. This model provides a baseline prediction. Next, we consult the marketing team, who estimate that a new advertising campaign will increase sales by 15% in the next quarter. We incorporate this qualitative input by adjusting the linear regression forecast upward by 15%. This adjusted figure represents our hybrid forecast, incorporating both historical trends and expert judgment. The model’s accuracy will depend on the reliability of the data and the expertise of the marketing team. While this is a simplified example, it illustrates the basic principle of integrating quantitative and qualitative data in a hybrid forecasting model. More sophisticated hybrid models might employ more complex statistical techniques and incorporate a wider range of qualitative data sources.
Evaluating Forecast Accuracy
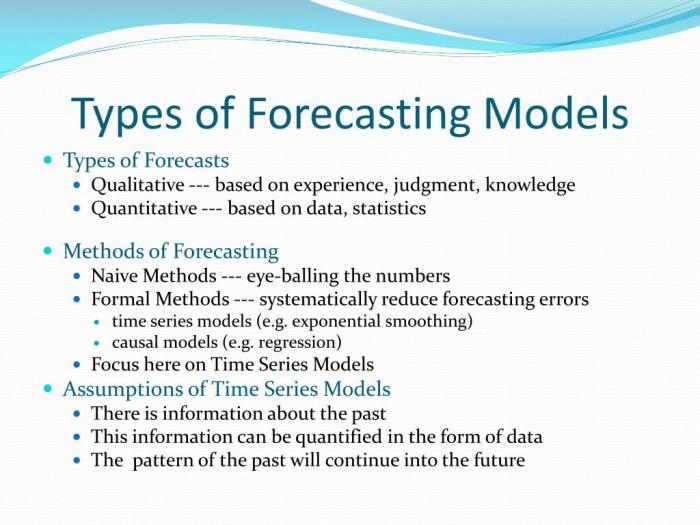
Predicting the future of the economy is a bit like predicting the weather in Scotland – you can make an educated guess, but you’re bound to be wrong sometimes. Therefore, evaluating the accuracy of our economic forecasts is crucial. This involves employing various metrics to quantify how far off our predictions are from reality and choosing the right metrics based on the specific forecasting problem. Let’s dive into the wonderfully wonky world of forecast evaluation!
Forecast Accuracy Metrics
Several metrics exist to assess forecast accuracy, each with its own strengths and weaknesses. The choice of metric depends heavily on the context – what are we trying to predict? How costly are different types of errors? Are we more concerned about consistently underestimating or overestimating? Let’s examine some of the most popular options.
Metric | Formula | Interpretation | Example |
---|---|---|---|
Mean Absolute Error (MAE) | MAE = (1/n) * Σ|Actuali - Forecasti| |
Average absolute difference between actual and forecasted values. Simple to understand but doesn’t penalize large errors disproportionately. | Imagine forecasting monthly ice cream sales. If MAE is 100 units, it means on average, our forecasts were off by 100 units. |
Root Mean Squared Error (RMSE) | RMSE = √[(1/n) * Σ(Actuali - Forecasti)²] |
Square root of the average of squared differences. Penalizes larger errors more heavily than MAE. Useful when large errors are particularly costly. | A lower RMSE indicates a better fit. For example, if RMSE for predicting GDP growth is 0.5%, it suggests our forecasts are, on average, off by 0.5% in terms of squared error. |
Mean Absolute Percentage Error (MAPE) | MAPE = (1/n) * Σ(|Actuali - Forecasti| / Actuali) * 100% |
Average percentage difference. Useful for comparing accuracy across different scales. However, it can be undefined if actual values are zero. | A MAPE of 5% suggests that, on average, our forecasts are off by 5% of the actual value. Useful for comparing forecasts across different products or markets. |
Symmetric Mean Absolute Percentage Error (sMAPE) | sMAPE = (1/n) * Σ(2 * |Actuali - Forecasti| / (|Actuali| + |Forecasti|) * 100% |
Similar to MAPE but addresses the issue of undefined values when actual values are zero. | Provides a more robust measure of forecast accuracy than MAPE, especially when dealing with data containing zero values. A lower sMAPE indicates better forecast accuracy. |
Selecting Appropriate Accuracy Metrics
The best metric isn’t a one-size-fits-all solution. Consider the following factors:
* Cost of errors: Are large errors significantly more problematic than small ones? If so, RMSE is a better choice than MAE.
* Scale of data: If comparing forecasts across different scales (e.g., sales of different products), MAPE or sMAPE is more appropriate.
* Presence of zero values: Avoid MAPE if your data contains zero values; use sMAPE instead.
* Interpretability: MAE is generally easier to interpret than RMSE.
Comparing Forecast Accuracy
Once you’ve chosen your accuracy metric(s), comparing the accuracy of different forecasting methods is straightforward. Simply calculate the chosen metric for each method and compare the results. Lower values generally indicate higher accuracy. Remember, a single metric might not tell the whole story; it’s often beneficial to use multiple metrics to get a comprehensive view. For example, a model might have a low MAE but a high RMSE, indicating good overall accuracy but a tendency towards occasional large errors.
Challenges and Future Directions in Economic Forecasting
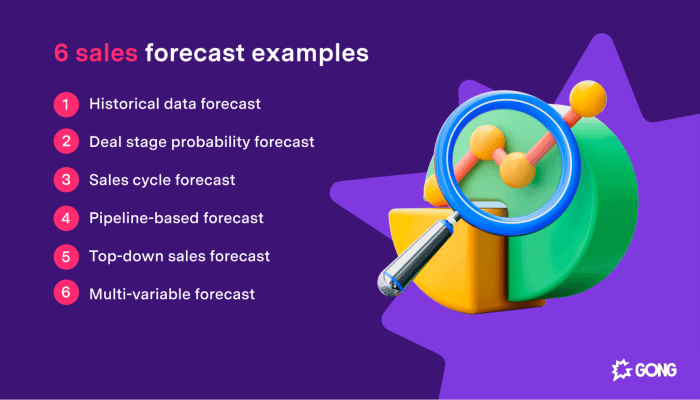
Predicting the future of the economy is like trying to herd cats – chaotic, unpredictable, and occasionally hilarious. While economists have developed sophisticated tools, forecasting remains a challenging endeavor, particularly in our increasingly complex and volatile world. The inherent uncertainties, coupled with the sheer volume of data available, present both opportunities and significant hurdles.
Economic forecasting faces a perfect storm of challenges. The interconnectedness of global markets means a sneeze in one country can trigger a full-blown pandemic (metaphorically speaking, of course… mostly) elsewhere. Unforeseen events, from geopolitical upheavals to technological disruptions, can render even the most meticulously crafted models obsolete faster than a politician’s campaign promise. Furthermore, the very act of forecasting can influence the outcome, creating a self-fulfilling prophecy (or, conversely, a self-defeating one).
The Role of Big Data and Artificial Intelligence
The sheer volume of data generated daily presents both a blessing and a curse. Big data, encompassing everything from social media sentiment to satellite imagery of agricultural yields, offers a wealth of information previously unavailable to forecasters. Artificial intelligence (AI), with its ability to process and analyze this data at unprecedented speeds, holds immense potential for improving forecast accuracy. For example, AI algorithms can identify subtle patterns and correlations that might escape human observation, leading to more nuanced and accurate predictions. However, the challenge lies in effectively harnessing this power, ensuring data quality, and addressing potential biases embedded within the algorithms themselves. Imagine an AI trained primarily on historical data from a period of low inflation – its predictions might be wildly inaccurate during a period of high inflation.
Uncertainty and Risk in Economic Forecasting
Acknowledging uncertainty isn’t just good practice; it’s essential. Economic forecasts should not be presented as definitive predictions, but rather as probabilistic statements reflecting a range of possible outcomes. This requires incorporating measures of uncertainty and risk into the forecasting process, such as using confidence intervals or scenario planning. For instance, a forecast might predict 2% GDP growth with a 95% confidence interval of 1% to 3%, acknowledging the inherent uncertainty in the prediction. Failing to account for uncertainty can lead to disastrous policy decisions based on overly optimistic (or pessimistic) forecasts. The 2008 financial crisis serves as a stark reminder of the perils of neglecting risk assessment in economic modeling.
Future Developments in Economic Forecasting Methodologies
The future of economic forecasting likely involves a greater integration of diverse data sources, advanced statistical techniques, and agent-based modeling. Agent-based models simulate the interactions of individual economic agents (consumers, firms, etc.), allowing forecasters to explore the potential consequences of various policies or shocks. Furthermore, advancements in machine learning, particularly deep learning, could lead to the development of more sophisticated and accurate forecasting models. We can anticipate a shift towards more dynamic and adaptive models capable of learning and adjusting in real-time as new data becomes available. This evolution necessitates a greater emphasis on interdisciplinary collaboration, bringing together economists, statisticians, computer scientists, and data scientists to tackle the complex challenges of economic forecasting. The future isn’t just about better models; it’s about building models that are more robust, transparent, and adaptable to the ever-changing economic landscape.
Summary
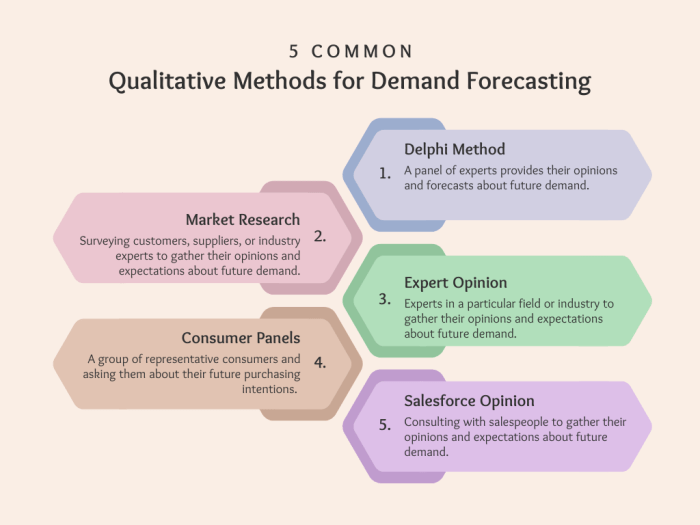
So, can we truly predict the future of the economy? The short answer, as any seasoned economist will tell you, is a resounding “maybe.” This Economic Forecasting Methods Review has shown us that while perfect prediction remains elusive (thank goodness for that, otherwise, where’s the fun?), a sophisticated blend of quantitative and qualitative methods, coupled with a healthy dose of humility, offers the best chance at navigating the turbulent waters of economic uncertainty. And who knows, maybe one day we’ll all be able to accurately predict the next market crash…or at least have a good laugh trying.
Answers to Common Questions
What are the ethical considerations in economic forecasting?
Predicting economic downturns can have significant ethical implications. Misleading forecasts can lead to poor policy decisions and harm vulnerable populations. Transparency and responsible communication of uncertainties are crucial.
How do geopolitical events affect economic forecasts?
Geopolitical events, like wars or trade disputes, introduce significant uncertainty and volatility, making accurate forecasting extremely challenging. These events often require adjustments to models and forecasts.
Can economic forecasting predict individual company performance?
While macroeconomic forecasting provides a general economic context, it doesn’t directly predict individual company performance. That requires a different set of analyses and models focused on specific company factors.