Data Analytics Finance Tools Review: Prepare yourself for a whirlwind tour of the fascinating world where spreadsheets meet sorcery and algorithms become your new best friend! We’ll unravel the mysteries of how financial institutions are leveraging the power of data analytics, transforming number crunching from a tedious chore into a high-stakes game of predictive prowess. Get ready for a journey through the exciting landscape of financial data, where insights are mined, risks are mitigated, and fortunes are—hopefully—made.
This review explores the diverse array of data analytics tools transforming the financial industry. From traditional spreadsheet software to sophisticated AI-powered platforms, we’ll examine their functionalities, compare their strengths and weaknesses, and delve into real-world applications across risk management, investment strategies, and regulatory compliance. We’ll even uncover the secrets to creating dazzling financial dashboards that would make even the most seasoned accountant weep with joy (tears of sheer data-driven brilliance, of course).
Introduction to Data Analytics in Finance
Finance, once the realm of spreadsheets and gut feelings, is undergoing a thrilling metamorphosis. Data analytics is no longer a futuristic fantasy; it’s the beating heart of modern financial decision-making. This transformation isn’t just about crunching numbers faster; it’s about unlocking insights previously hidden within the vast oceans of financial data, leading to smarter strategies and more profitable outcomes. Think of it as upgrading from a rusty abacus to a supercharged, AI-powered financial calculator.
The benefits of harnessing data analytics tools are numerous and profoundly impactful. Improved risk management, enhanced fraud detection, more accurate forecasting, and optimized investment strategies are just a few of the rewards. Essentially, data analytics allows financial institutions to move from reactive to proactive, transforming uncertainty into informed action. Imagine predicting market crashes before they happen – that’s the power we’re talking about (though, of course, perfect prediction remains elusive, even with the best tools!).
Types of Financial Data Used in Analytics
Financial data comes in many flavors, each offering unique insights. We’re not just talking about balance sheets and income statements anymore (though those still play a crucial role!). Consider the richness of transactional data – every credit card swipe, every stock trade, every loan application – all offering a treasure trove of information. Market data, encompassing everything from stock prices to interest rates, provides crucial context. Alternative data sources, such as social media sentiment and satellite imagery (yes, really!), are also gaining traction, providing unconventional yet valuable perspectives. Finally, internal data, encompassing operational metrics and customer behavior, provides a granular understanding of a company’s performance and its interactions with its client base.
Comparison of Traditional and Data-Driven Financial Analysis
The shift from traditional to data-driven approaches is nothing short of revolutionary. Let’s compare these two approaches using a simple table:
Feature | Traditional Financial Analysis | Data-Driven Financial Analysis |
---|---|---|
Data Source | Primarily historical financial statements | Historical and real-time data from diverse sources (financial statements, market data, transactional data, alternative data) |
Methodology | Relies heavily on manual calculations and pre-defined ratios | Utilizes advanced statistical modeling, machine learning, and AI |
Insights | Provides retrospective analysis and limited predictive capabilities | Offers both retrospective and predictive insights, identifying patterns and anomalies |
Decision Making | Primarily based on experience and intuition | Data-informed and evidence-based decision-making |
Types of Data Analytics Finance Tools
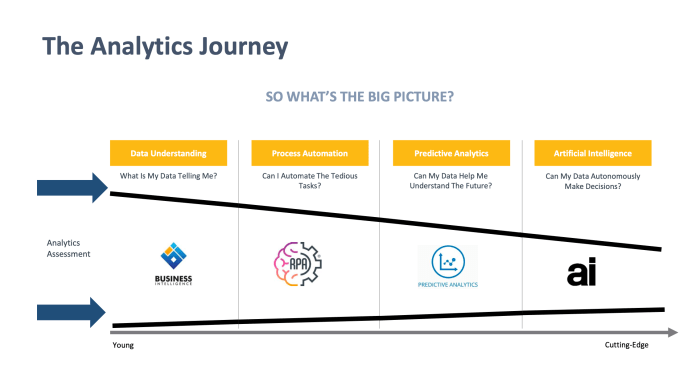
The world of finance is awash in data – more than a whale in the ocean, frankly. To navigate this deluge and extract meaningful insights, we need the right tools. Think of data analytics tools as your trusty financial submarines, capable of diving deep into the numbers and surfacing with treasures (or at least, actionable intelligence). This section explores the various types of these indispensable tools, comparing their strengths and weaknesses with the charm of a seasoned financial analyst recounting tales from the trenches.
Data analytics tools in finance are broadly categorized based on their functionality and complexity. From simple spreadsheets to sophisticated AI-powered platforms, each category serves a unique purpose and caters to different skill levels and analytical needs. The choice of tool often depends on the specific task at hand, the size of the dataset, and the user’s technical expertise. Choosing the wrong tool is like trying to build a skyscraper with toothpicks – it might look impressive initially, but it’s unlikely to stand the test of time (or a strong gust of wind).
Spreadsheet Software
Spreadsheet software, the workhorse of many a financial analyst, forms the foundation of basic data analysis. These ubiquitous tools allow for data entry, manipulation, and visualization through formulas, charts, and graphs. Think of Excel as the friendly neighborhood plumber – always available, often helpful, and capable of handling most everyday tasks.
Let’s compare three prominent players: Microsoft Excel, Google Sheets, and LibreOffice Calc. Excel boasts extensive functionality, powerful add-ins, and a vast user community. However, its price point can be a barrier for some. Google Sheets offers a collaborative, cloud-based alternative, free and accessible from anywhere with an internet connection. However, its functionality might lag behind Excel in some areas. LibreOffice Calc provides a free and open-source option, a good compromise for those seeking a powerful tool without the cost. Each has its strengths and weaknesses, much like a three-legged stool – one leg might be stronger than the others, but all three are needed for stability.
Statistical Packages
When spreadsheet software isn’t enough, statistical packages step in to handle more complex analyses. These tools are designed for statistical modeling, hypothesis testing, and other advanced analytical techniques. They’re like the specialized surgeons of the data world – called in for the intricate procedures that spreadsheets simply can’t handle.
Consider R, SAS, and SPSS. R is a powerful, open-source language favored for its flexibility and vast library of statistical packages. However, its steep learning curve can be daunting for beginners. SAS, a commercial package, offers a more user-friendly interface and robust data management capabilities, but comes with a hefty price tag. SPSS, another commercial package, is known for its user-friendly interface and comprehensive statistical procedures, but it can be less flexible than R.
Specialized Financial Analytics Platforms
These platforms are the heavy hitters, offering integrated solutions for various financial tasks, including portfolio management, risk analysis, and financial modeling. Think of them as the Swiss Army knives of finance – packed with tools for every conceivable task.
Bloomberg Terminal, Refinitiv Eikon, and FactSet are examples of such platforms. Bloomberg Terminal is known for its comprehensive data coverage and real-time market information. However, its high cost and complexity make it inaccessible to many. Refinitiv Eikon offers a more affordable alternative with a wide range of analytical tools. FactSet focuses on providing high-quality fundamental data and analytical tools, particularly for equity research. The choice depends heavily on the specific needs of the user and their budget – a bit like choosing a car; some prioritize speed, others fuel efficiency.
Key Features of Leading Data Analytics Finance Tools, Data Analytics Finance Tools Review
Tool | Data Handling | Analytical Capabilities | Cost |
---|---|---|---|
Microsoft Excel | Large datasets, various formats | Basic statistical analysis, charting | Subscription or one-time purchase |
R | Large datasets, various formats | Advanced statistical modeling, machine learning | Free and open-source |
Bloomberg Terminal | Real-time market data, financial statements | Portfolio analysis, risk management | High subscription cost |
SAS | Large datasets, various formats | Advanced statistical modeling, data mining | High licensing cost |
Tableau | Various data sources | Data visualization, interactive dashboards | Subscription based |
Applications of Data Analytics Tools in Finance
Data analytics tools are no longer a luxury in finance; they’re the lifeblood of efficient, profitable, and—dare we say—fun operations. From spotting sneaky fraudsters to optimizing investment portfolios with the precision of a Swiss watchmaker, these tools are revolutionizing the industry, one algorithm at a time. Let’s delve into the exciting world of data analytics applications in finance.
Risk Management with Data Analytics
Data analytics plays a crucial role in mitigating financial risks. Sophisticated algorithms can sift through mountains of transactional data to identify unusual patterns indicative of fraudulent activity. Credit scoring models, powered by machine learning, assess the creditworthiness of individuals and businesses with far greater accuracy than traditional methods. Similarly, market risk assessment utilizes advanced statistical techniques to predict potential market downturns and inform hedging strategies. Imagine a world where fraud is detected before it even happens – that’s the power of predictive analytics in risk management. For example, a bank might use anomaly detection algorithms to flag suspicious transactions based on factors like transaction amount, location, and time of day, preventing significant financial losses.
Investment Management and Data Analytics
Investment management is undergoing a data-driven transformation. Portfolio optimization algorithms leverage historical data and market trends to construct diversified portfolios that maximize returns while minimizing risk. Algorithmic trading systems execute trades at optimal prices and speeds, often outperforming human traders. Sentiment analysis, a powerful tool in this space, gauges market sentiment by analyzing news articles, social media posts, and other textual data, providing valuable insights into investor behavior and potential market shifts. For instance, an algorithmic trading system might identify a profitable arbitrage opportunity by analyzing price discrepancies across different exchanges in milliseconds, executing trades automatically and generating profits.
Regulatory Compliance and Reporting with Data Analytics
Navigating the complex world of financial regulations is a significant challenge for financial institutions. Data analytics streamlines regulatory compliance and reporting processes. These tools automate the collection, analysis, and reporting of data required by regulatory bodies, ensuring accuracy and reducing the risk of non-compliance. For example, a bank can use data analytics to monitor transactions for compliance with anti-money laundering (AML) regulations, automatically flagging suspicious activities for further investigation. The ability to rapidly generate accurate regulatory reports saves time and resources, allowing financial institutions to focus on their core business.
Financial Forecasting and Budgeting with Data Analytics
Accurate financial forecasting and budgeting are critical for successful financial planning. Data analytics tools enhance the accuracy and efficiency of these processes. By analyzing historical financial data, market trends, and economic indicators, these tools can generate more accurate forecasts and budgets, enabling better decision-making. For instance, a retail company can use data analytics to forecast sales for the upcoming quarter by analyzing past sales data, seasonality, and promotional activities. This allows the company to optimize inventory levels, staffing, and marketing campaigns, leading to improved profitability. Predictive models, based on historical data and external factors, provide insights into potential future scenarios, enabling proactive planning and risk mitigation.
Data Visualization and Reporting in Finance: Data Analytics Finance Tools Review
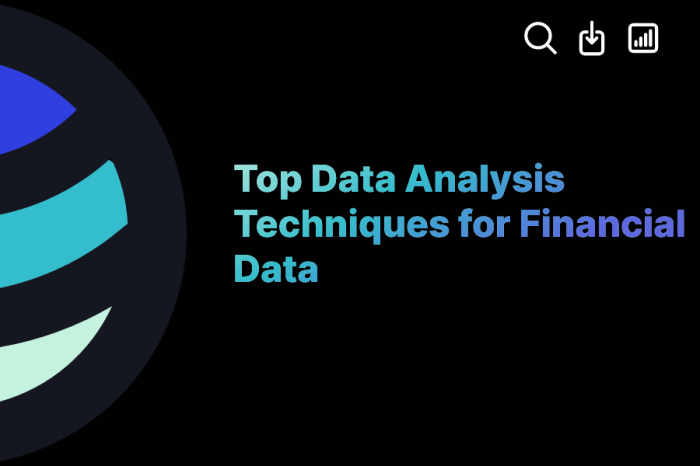
Let’s face it, financial data can be drier than a week-old bagel. Unless, of course, you spice it up with some dazzling data visualization. Transforming raw numbers into compelling visuals is the key to unlocking financial insights and, more importantly, getting your point across without inducing a coma in your audience. Think of it as financial data’s very own superhero origin story – from drab spreadsheet to dynamic, insightful graphic novel.
Data visualization is paramount in effectively communicating financial insights. A picture, as they say, is worth a thousand spreadsheets (and probably a few thousand hours of tedious analysis). By presenting complex data in a clear, concise, and visually appealing manner, we can transform confusing numbers into easily digestible narratives that inform decision-making. This isn’t just about making pretty pictures; it’s about enabling stakeholders to quickly grasp key trends, identify potential problems, and celebrate successes – all without needing a finance degree.
Types of Charts and Graphs for Financial Data
Various chart types cater to different financial data representations, each with its strengths. Choosing the right chart is crucial for effective communication. The wrong chart can obscure insights, while the right one can illuminate hidden patterns. Let’s explore a few key players in the financial visualization arena.
- Line Charts: Ideal for showcasing trends over time, such as revenue growth, stock prices, or expenditure patterns. A line chart’s smooth curves elegantly illustrate the ebb and flow of financial performance.
- Bar Charts: Perfect for comparing different categories, like comparing sales across various product lines or investment returns across different asset classes. The distinct bars provide immediate visual comparisons.
- Scatter Plots: Useful for identifying correlations between two variables, such as the relationship between marketing spend and sales revenue. Clustering or patterns in the data points reveal potential relationships.
- Pie Charts: Effective for showing the proportion of different components within a whole, such as the allocation of a portfolio across different asset classes. A visually appealing way to demonstrate the relative contribution of each component.
Creating a Compelling Financial Report Using Data Visualization
Let’s craft a mini-financial report using data visualization. Imagine we’re analyzing the quarterly performance of a fictional company, “Acme Corp.”
Quarter | Revenue | Expenses | Profit |
---|---|---|---|
Q1 | $100,000 | $60,000 | $40,000 |
Q2 | $120,000 | $70,000 | $50,000 |
Q3 | $150,000 | $80,000 | $70,000 |
Q4 | $180,000 | $90,000 | $90,000 |
A compelling visual representation would involve a combined bar chart and line chart. The bar chart would visually compare revenue, expenses, and profit across the four quarters. A line chart overlaid on top would clearly demonstrate the trend of profit growth over time. This combination provides a clear, concise summary of Acme Corp.’s financial performance, highlighting both the absolute values and the growth trajectory. Adding clear labels and a title (“Acme Corp. Quarterly Performance”) completes the picture.
Best Practices for Designing Effective Financial Dashboards
Financial dashboards are the ultimate power tools for visualizing key performance indicators (KPIs). They’re the executive summary of your data, presenting the most critical information at a glance. To make them truly effective, however, requires careful design.
- Prioritize Key Metrics: Focus on the most important KPIs. Avoid overwhelming the dashboard with irrelevant data.
- Visual Clarity: Use clear, concise charts and graphs. Avoid cluttered visuals or overly complex designs.
- Interactive Elements: Incorporate interactive elements, such as drill-down capabilities, to allow users to explore the data in more detail.
- Consistent Design: Maintain a consistent visual style throughout the dashboard to ensure ease of navigation and understanding.
- Regular Updates: Ensure the dashboard is regularly updated with the latest data to maintain its relevance and value.
Challenges and Considerations in Using Data Analytics Tools
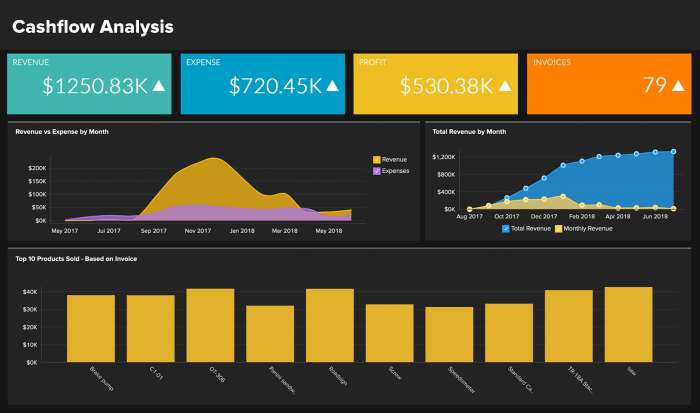
Embarking on the thrilling journey of data analytics in finance is like scaling Mount Everest – exhilarating, rewarding, but fraught with potential pitfalls. While the panoramic views from the summit (i.e., insightful financial decisions) are breathtaking, the climb itself requires careful planning, the right gear, and a healthy dose of risk management. Ignoring the challenges can lead to a swift and unceremonious descent back to the base camp of uninformed decision-making.
Data analytics in finance, while promising, isn’t without its hurdles. Navigating these challenges requires a strategic approach, combining technical expertise with a robust understanding of financial regulations and ethical considerations. Failure to address these issues can lead to inaccurate analyses, regulatory violations, and even reputational damage. Let’s delve into the specifics.
Data Quality Issues
The age-old adage, “garbage in, garbage out,” rings particularly true in the world of financial data analytics. Inaccurate, incomplete, or inconsistent data can lead to flawed models and ultimately, poor financial decisions. For example, imagine a bank relying on a credit scoring model trained on data containing numerous missing values or inconsistencies in the definition of “income.” The resulting credit risk assessment would be unreliable, potentially leading to increased loan defaults. Ensuring data quality requires meticulous data cleaning, validation, and ongoing monitoring to identify and correct errors. This often involves sophisticated data profiling techniques and the establishment of clear data quality standards.
Data Security Concerns
Financial data is incredibly sensitive. Breaches can result in significant financial losses, reputational damage, and regulatory penalties. The implementation of robust security measures, including encryption, access controls, and regular security audits, is paramount. Consider the case of a hedge fund that experienced a data breach, resulting in the theft of proprietary trading algorithms and sensitive client information. The financial and reputational consequences were devastating.
Cost of Implementation
Implementing data analytics tools isn’t cheap. The initial investment in software, hardware, and skilled personnel can be substantial. Furthermore, ongoing maintenance and updates require a continuous financial commitment. A comprehensive cost-benefit analysis is crucial before embarking on any data analytics initiative. For example, a small investment firm might find that the cost of implementing a sophisticated risk management system outweighs the potential benefits, while a large multinational bank may readily absorb these costs due to the substantial potential returns.
Data Governance and Compliance
Data governance establishes a framework for managing data throughout its lifecycle, ensuring its quality, security, and compliance with relevant regulations. Compliance with regulations like GDPR (General Data Protection Regulation) and CCPA (California Consumer Privacy Act) is non-negotiable. Failure to comply can result in hefty fines and legal repercussions. Imagine a financial institution failing to obtain proper consent before using customer data for targeted advertising; the penalties could be severe.
Ethical Considerations
The use of AI-driven analytics in finance raises several ethical concerns. Bias in algorithms, for example, can lead to discriminatory outcomes. Transparency and explainability are also crucial to ensure fairness and accountability. Consider the potential for algorithmic bias in loan applications, where a model trained on historical data might inadvertently discriminate against certain demographic groups.
Strategies to Mitigate Risks
Implementing robust data governance policies and procedures is paramount to mitigate risks. This includes establishing clear data ownership, access controls, and data quality standards. Regular audits and security assessments are crucial for identifying and addressing vulnerabilities. Furthermore, investing in employee training and awareness programs is vital to ensure that employees understand the importance of data security and ethical considerations. Finally, developing a comprehensive incident response plan will help to minimize the impact of any data breaches.
- Implement robust data governance policies and procedures.
- Conduct regular security assessments and audits.
- Invest in employee training and awareness programs.
- Develop a comprehensive incident response plan.
- Prioritize data quality through rigorous cleaning and validation processes.
- Employ advanced encryption and access control mechanisms.
- Regularly review and update data analytics tools and processes.
Future Trends in Data Analytics for Finance
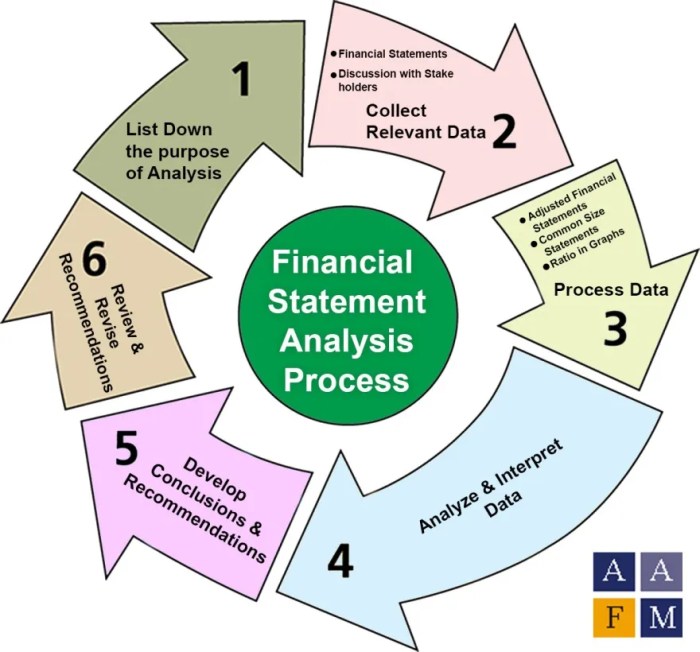
The future of financial data analytics is less about spreadsheets and more about sentient robots predicting market movements (almost). Seriously though, the field is undergoing a rapid transformation, driven by advancements in technology and a growing appetite for data-driven decision-making. Expect the unexpected – and probably a few surprises along the way.
The convergence of big data, artificial intelligence (AI), and machine learning (ML) is reshaping the financial landscape at a breakneck pace. We’re talking about analyzing massive datasets, identifying subtle patterns invisible to the human eye, and predicting future trends with increasing accuracy. This isn’t just about improving efficiency; it’s about unlocking entirely new possibilities for investment strategies, risk management, and fraud detection.
The Impact of Cloud Computing on Financial Data Analytics
Cloud computing is the unsung hero of this data revolution. Its scalability and cost-effectiveness allow financial institutions of all sizes to access and process vast amounts of data previously beyond their reach. Imagine a small hedge fund having the same analytical power as a multinational bank – that’s the democratizing effect of the cloud. This increased accessibility fuels innovation and fosters a more competitive market, leading to better products and services for consumers. Moreover, cloud-based solutions offer enhanced security and disaster recovery capabilities, mitigating risks associated with on-premise data storage. For example, many financial institutions are leveraging cloud services like Amazon Web Services (AWS) and Microsoft Azure to build their data analytics platforms, enabling them to scale their operations and reduce IT infrastructure costs.
Innovative Applications of Data Analytics in the Future of Finance
The integration of blockchain technology and decentralized finance (DeFi) presents exciting new opportunities. Blockchain’s inherent transparency and security can revolutionize financial transactions, providing auditable records and reducing the risk of fraud. DeFi platforms, powered by smart contracts and decentralized applications (dApps), are already experimenting with innovative lending, borrowing, and investment models, often leveraging AI and ML for risk assessment and portfolio optimization. For instance, AI-powered algorithms are being used to assess creditworthiness on DeFi platforms, potentially extending financial services to individuals previously excluded from traditional banking systems. Imagine a future where microloans are automatically disbursed based on real-time data analysis of a borrower’s blockchain activity – a far cry from traditional credit scoring methods.
Predictions for the Future Landscape of Data Analytics Finance Tools
We foresee a future where data analytics tools become increasingly sophisticated, intuitive, and user-friendly. Expect more integration with other financial technologies, including robo-advisors and algorithmic trading platforms. The demand for specialized skills in data science and AI will continue to grow, creating new career opportunities in the financial sector. We can anticipate the emergence of niche tools tailored to specific financial needs, such as personalized risk management dashboards and AI-driven fraud detection systems. Furthermore, the ethical considerations surrounding the use of AI in finance will come to the forefront, leading to stricter regulations and increased transparency. For example, we might see the development of explainable AI (XAI) techniques to ensure that algorithmic decisions are understandable and unbiased. This shift towards transparency and accountability is crucial for maintaining public trust in the increasingly automated financial system.
Ending Remarks
So, there you have it – a glimpse into the exciting and ever-evolving world of data analytics in finance. While the challenges are real (data quality, security, ethical considerations – oh my!), the potential rewards are even greater. As we navigate the future of finance, fueled by increasingly sophisticated tools and techniques, one thing remains certain: data is king, and those who master its analysis will reign supreme. Now go forth and conquer those spreadsheets!
Expert Answers
What are the ethical considerations of using AI in financial analytics?
AI in finance raises ethical concerns regarding bias in algorithms, data privacy violations, and the potential for unfair or discriminatory outcomes. Robust oversight and ethical guidelines are crucial.
How can I choose the right data analytics tool for my needs?
Consider your budget, technical expertise, data volume, and specific analytical needs. Start with a clear understanding of your goals and explore free trials or demos before committing.
What is the future of data visualization in finance?
Expect more interactive and immersive visualizations, leveraging AI for automated insights and personalized dashboards, further enhancing decision-making.