Data Analytics Finance Tools Review: Prepare for a whirlwind tour of the fascinating world where finance meets data! We’ll explore the evolution of these powerful tools, from abacus-assisted calculations to the AI-powered marvels of today. Get ready for a journey that’s both enlightening and (dare we say) entertaining, as we dissect the best tools, unravel their secrets, and reveal how they’re reshaping the financial landscape. Buckle up, it’s going to be a wild ride!
This review delves into the core functionalities of leading data analytics tools specifically designed for the financial sector. We’ll examine their strengths and weaknesses, showcasing real-world applications in areas like risk management, fraud detection, and regulatory compliance. Think of it as a high-stakes game of financial Jenga, where each tool is a carefully placed block contributing to the stability (or potential collapse!) of the entire financial system. We’ll even peek into the crystal ball and speculate on the future of these game-changing technologies.
Introduction to Data Analytics in Finance
Finance, once a realm of gut feelings and spreadsheets thicker than phone books, has undergone a dramatic transformation. The rise of data analytics has injected a much-needed dose of precision and predictability into the often-chaotic world of money. No longer are financial decisions based solely on intuition; data now provides the compass, guiding us through the sometimes murky waters of investment, risk management, and fraud detection.
Data analytics in finance leverages vast quantities of information to identify trends, predict outcomes, and ultimately, make better-informed decisions. This involves collecting, cleaning, analyzing, and interpreting data from various sources, including market data, customer transactions, and internal financial records. The result? A clearer picture of the financial landscape, allowing for more strategic and profitable maneuvers.
The Evolution of Data Analytics Tools in Finance
The tools used in financial data analytics have evolved dramatically, mirroring the technological advancements of the past few decades. Early methods relied heavily on manual calculations and basic statistical analysis. Think legions of analysts hunched over calculators, poring over endless rows of numbers. Then came the dawn of spreadsheet software, a significant leap forward, allowing for more efficient data manipulation and analysis. However, the real revolution began with the advent of powerful statistical software packages and, more recently, the rise of machine learning and artificial intelligence. These sophisticated tools can now handle massive datasets, uncovering complex patterns and relationships that would be impossible to detect manually. This evolution has led to a shift from reactive to proactive strategies, allowing financial institutions to anticipate market changes and mitigate risks more effectively.
Examples of Data Analytics Improving Financial Decision-Making
The impact of data analytics on financial decision-making is profound and far-reaching. Consider algorithmic trading, where sophisticated algorithms analyze market data in real-time to execute trades at optimal prices. This high-frequency trading relies entirely on the speed and accuracy of data analytics. Another prime example is credit scoring, where algorithms assess the creditworthiness of individuals and businesses, helping lenders make informed lending decisions and minimizing the risk of defaults. Furthermore, fraud detection systems, powered by machine learning, analyze transaction patterns to identify suspicious activity, protecting financial institutions and customers from losses. Even seemingly mundane tasks, such as portfolio optimization, benefit immensely from data-driven insights, leading to more efficient allocation of assets and improved returns. The use of predictive modeling allows financial institutions to forecast future market trends, enabling them to adjust their strategies proactively and mitigate potential risks. For instance, predicting potential loan defaults allows banks to proactively adjust lending policies and reduce overall risk exposure.
Categorizing Finance Data Analytics Tools
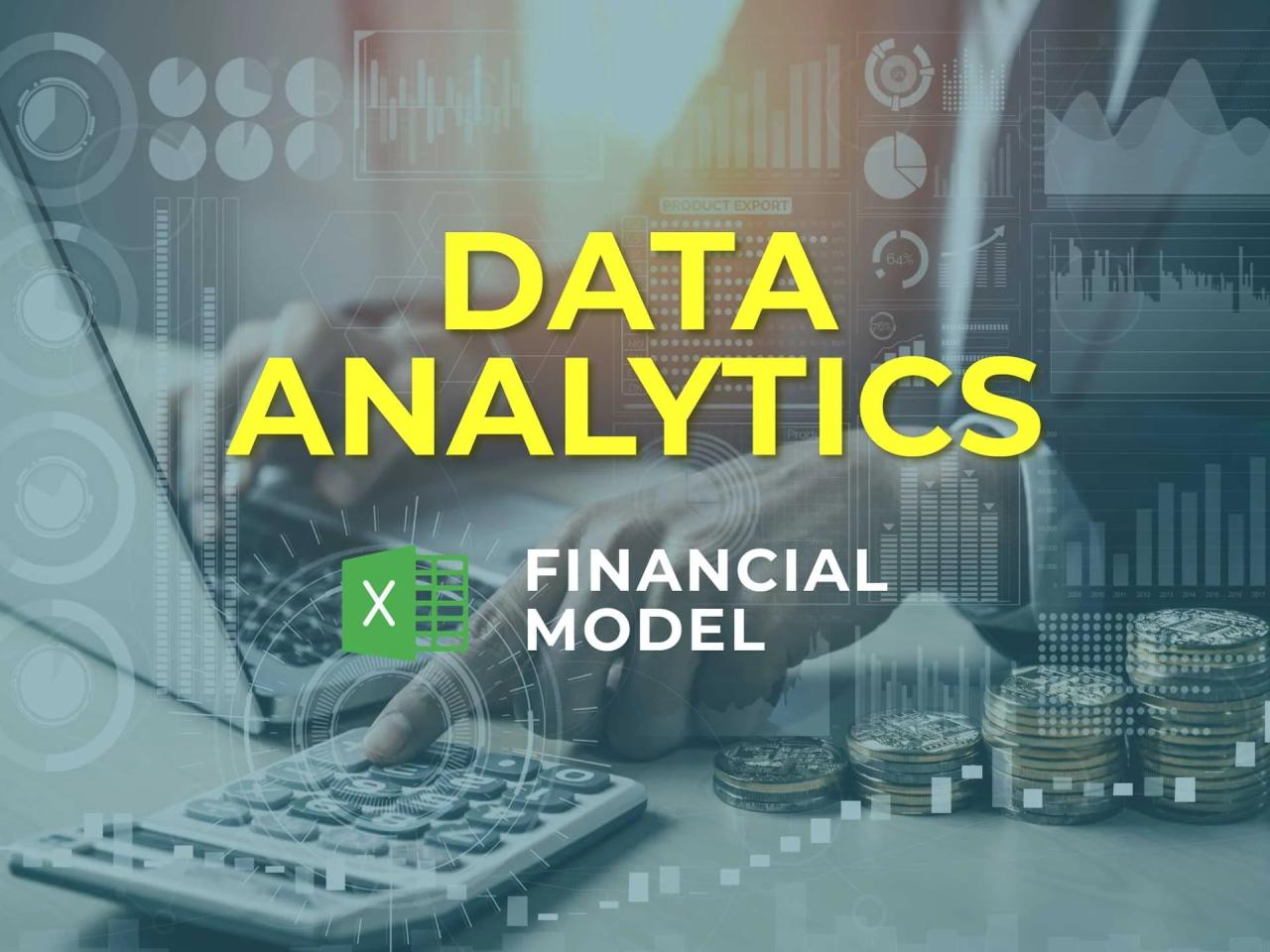
The world of finance is awash in data – a veritable ocean of numbers, transactions, and market movements. Navigating this ocean requires the right tools, and thankfully, a plethora of data analytics solutions exist to help financial professionals chart a course to profitability (and avoid the financial equivalent of hitting an iceberg). Let’s categorize these indispensable tools to better understand their strengths and weaknesses. Buckle up, it’s going to be a wild ride!
Data analytics tools in finance can be broadly categorized by their core functionality. While some tools may offer overlapping capabilities, understanding their primary focus helps in selecting the right tool for a specific task. Think of it like choosing the right tool from your toolbox – you wouldn’t use a hammer to screw in a screw, would you? (Unless you’re exceptionally strong and enjoy the satisfying *crack* of a broken screw.)
Finance Data Analytics Tools by Functionality
The following table organizes prominent data analytics tools used in finance, categorized by their primary function. We’ve tried to keep it concise, but remember, the world of finance tech is constantly evolving, so this isn’t an exhaustive list (though we’ve tried our best, we swear!).
Tool Name | Vendor | Key Features | Typical Use Cases |
---|---|---|---|
Tableau | Tableau Software (Salesforce) | Data visualization, interactive dashboards, data blending | Financial reporting, performance monitoring, identifying trends |
Power BI | Microsoft | Data visualization, interactive dashboards, data modeling, integration with Excel | Budgeting and forecasting, financial planning and analysis (FP&A), regulatory reporting |
Qlik Sense | Qlik | Data visualization, self-service BI, associative analytics | Risk management, fraud detection, customer analytics (for financial institutions) |
Python (with libraries like Pandas, NumPy, Scikit-learn) | Open-source community | Data manipulation, statistical analysis, machine learning | Algorithmic trading, risk modeling, portfolio optimization |
R (with libraries like dplyr, ggplot2, caret) | Open-source community | Statistical computing, data visualization, machine learning | Financial forecasting, time series analysis, credit scoring |
SAS | SAS Institute | Advanced analytics, statistical modeling, data mining | Regulatory compliance, risk assessment, fraud detection |
Bloomberg Terminal | Bloomberg L.P. | Real-time market data, financial analysis tools, news and research | Investment research, portfolio management, trading |
FactSet | FactSet Research Systems | Financial data, analytics, and research tools | Investment research, portfolio management, performance measurement |
Comparative Analysis of Leading Tools
Let’s delve into a more detailed comparison of three leading tools within each of the major categories (reporting, forecasting, and risk management). Remember, the “best” tool depends heavily on your specific needs and budget – choosing the right tool is a bit like choosing a financial instrument – it requires careful consideration of your risk tolerance and investment goals.
Reporting Tools Comparison:
Feature | Tableau | Power BI | Qlik Sense |
---|---|---|---|
Ease of Use | Excellent, intuitive drag-and-drop interface | Good, integrates well with Excel, but can be complex for large datasets | Good, but the associative engine can be overwhelming for beginners |
Data Visualization | Wide variety of charts and graphs, highly customizable | Strong visualization capabilities, but can feel less polished than Tableau | Excellent for exploring data relationships, but can be less intuitive for static reports |
Cost | Subscription-based, can be expensive for large organizations | Subscription-based, various licensing options available | Subscription-based, pricing varies depending on features and users |
Forecasting Tools Comparison (Note: This section requires more specific tools dedicated to forecasting, which often integrate with other platforms):
Feature | Python (with Statsmodels) | R (with forecast package) | Specialized Financial Forecasting Software (e.g., Anaplan) |
---|---|---|---|
Flexibility | Highly flexible, allows for custom model development | Good flexibility, many pre-built forecasting models available | More structured, less flexible but often easier to use for business users |
Scalability | Highly scalable, can handle large datasets | Scalable, but performance can be impacted by very large datasets | Scalability depends on the specific software, generally good for large organizations |
Cost | Open-source (free), but requires skilled personnel | Open-source (free), but requires skilled personnel | Subscription-based, can be expensive |
Risk Management Tools Comparison (Note: Many risk management tools are specialized and often integrate with other platforms):
Feature | SAS | Specialized Risk Management Software (e.g., Moody’s Analytics) | Python (with specialized libraries) |
---|---|---|---|
Modeling Capabilities | Advanced statistical modeling, robust for complex risk scenarios | Specialized models for various risk types (credit, market, operational) | Highly flexible, allows for custom model development but requires expertise |
Data Integration | Good data integration capabilities, but can be complex to set up | Often integrates directly with financial data sources | Requires data wrangling and integration using Python libraries |
Cost | Expensive, requires specialized training | Expensive, often requires consulting services | Open-source (free), but requires skilled personnel and potentially additional library costs |
Specific Tool Deep Dives (Examples)
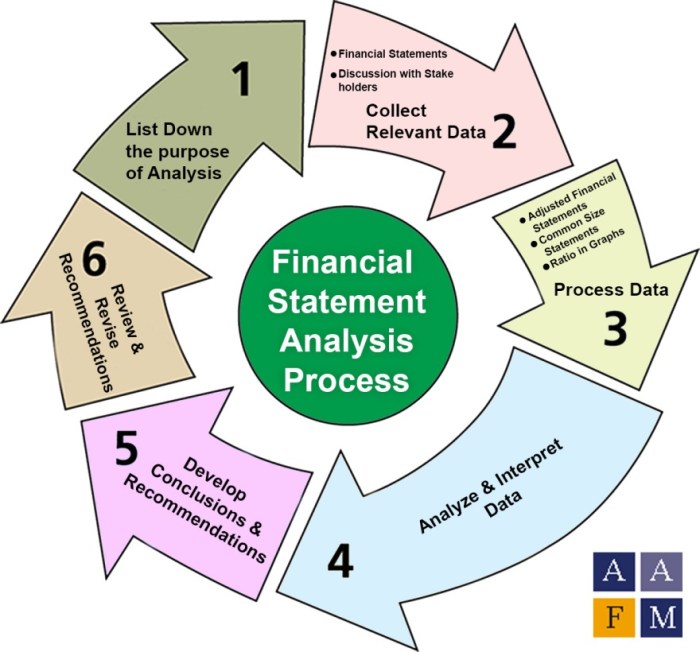
Choosing the right data analytics tool for financial tasks can feel like navigating a minefield of spreadsheets – exciting, potentially lucrative, and fraught with the danger of accidental explosions (of frustration). Let’s illuminate the path with a closer look at some popular choices, focusing on their strengths in the financial world. We’ll avoid the overly technical jargon and instead focus on practical application.
Tableau for Financial Visualization
Tableau’s strength lies in its user-friendly interface and powerful visualization capabilities. Financial analysts can leverage Tableau to create compelling dashboards showcasing key performance indicators (KPIs), portfolio performance, and risk assessments. Its drag-and-drop functionality makes creating interactive charts and graphs a breeze, even for those less familiar with coding.
For example, imagine visualizing a portfolio’s asset allocation. A simple pie chart in Tableau could clearly show the percentage of investments in stocks, bonds, and real estate. Further, interactive elements allow users to drill down into specific asset classes to see individual holdings and their performance. A color-coded heatmap could then overlay risk scores for each asset, providing a comprehensive overview of the portfolio’s risk profile at a glance. This visual representation surpasses the limitations of static spreadsheets, offering a dynamic and insightful perspective.
Consider a scenario involving a comparison of investment performance across different funds. A line chart in Tableau, comparing the returns of multiple funds over time, would immediately highlight top performers and underperformers. Users could add interactive filters to segment the data by investment strategy, risk level, or other relevant factors. The resulting dynamic visualization allows for a quick understanding of fund performance trends, supporting informed decision-making.
Power BI for Financial Reporting and Analysis
Power BI, Microsoft’s business analytics service, excels at connecting to various data sources, transforming raw data into insightful reports, and sharing those reports with colleagues. Its strong integration with Excel makes it a natural fit for many financial workflows.
Let’s say we want to track key financial metrics for a company across different quarters. Power BI can seamlessly integrate data from various sources, such as accounting software and CRM systems, to create a comprehensive dashboard. This dashboard could display revenue, expenses, profit margins, and other crucial indicators, enabling quick identification of trends and anomalies. The ability to create interactive reports, with drill-down capabilities to analyze underlying data, adds significant value. For instance, a user could click on a specific revenue figure to see a detailed breakdown by product line or region.
Furthermore, Power BI’s ability to create interactive maps is particularly useful for visualizing geographically dispersed financial data. Imagine displaying sales performance across different regions. A map in Power BI could instantly highlight high-performing and underperforming areas, allowing management to quickly identify opportunities for growth or areas requiring attention. The visual representation helps to communicate complex financial information in a concise and readily understandable format.
Python Libraries (Pandas, NumPy, Scikit-learn) for Advanced Financial Modeling
Python, combined with powerful libraries like Pandas, NumPy, and Scikit-learn, opens the door to sophisticated financial modeling and analysis. These libraries provide the tools for tasks ranging from portfolio optimization to fraud detection.
For portfolio optimization, Python’s capabilities shine. Using libraries like Scikit-learn, we can implement algorithms like the mean-variance optimization model. This involves defining constraints (e.g., risk tolerance, investment goals) and letting the algorithm find the optimal asset allocation that maximizes returns for a given level of risk. The results can then be visualized using Matplotlib or Seaborn, creating charts showing the efficient frontier – a set of optimal portfolios with varying levels of risk and return.
Fraud detection is another area where Python’s power becomes evident. Using Pandas for data manipulation and Scikit-learn for machine learning algorithms, we can build models to identify potentially fraudulent transactions. For instance, we might train a model on historical transaction data, labeling fraudulent and legitimate transactions. The model can then analyze new transactions, flagging those that have a high probability of being fraudulent. The model’s performance can be evaluated using metrics like precision and recall, ensuring its accuracy and effectiveness in identifying fraudulent activities.
Data Sources and Integration
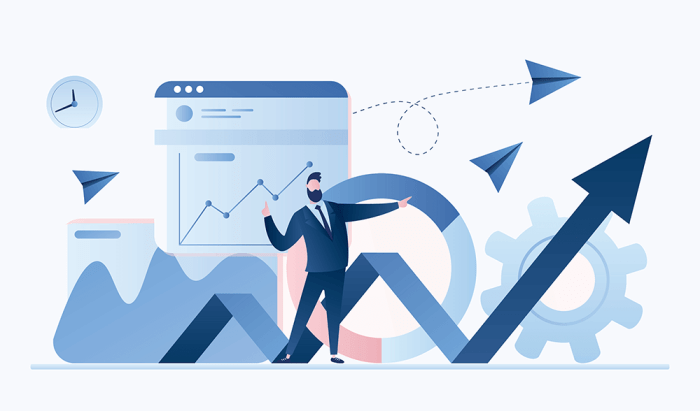
Ah, data integration – the beautiful ballet of wrestling disparate datasets into a harmonious whole. It’s less swan lake and more a wrestling match with a particularly stubborn octopus, but the results are equally breathtaking (if you manage to win). In the world of financial data analytics, successfully integrating your data is the key to unlocking truly insightful conclusions, rather than a confusing jumble of numbers that make even the most seasoned analyst question their career choices.
Financial data, like a particularly mischievous family, comes in all shapes and sizes. We’re talking transaction data (the bread and butter), market data (the volatile spice), and regulatory reports (the slightly tedious but essential side dish). Each source presents its own unique challenges, from incompatible formats to maddening inconsistencies. But fear not, intrepid data wranglers! With the right tools and techniques, you can tame this wild beast and extract valuable insights.
Common Financial Data Sources, Data Analytics Finance Tools Review
Transaction data, encompassing everything from credit card purchases to high-frequency trades, forms the backbone of many financial analyses. Market data, including stock prices, interest rates, and exchange rates, provides the external context crucial for understanding market trends and assessing risk. Regulatory reports, mandated by bodies like the SEC or FCA, offer a crucial compliance layer, ensuring accuracy and transparency. These reports, while often dense and complex, are goldmines of information for understanding industry trends and regulatory impacts. Think of it as finding buried treasure – tedious, but potentially incredibly rewarding.
Challenges of Data Integration
Integrating these diverse data sources is like trying to fit a square peg into a round hole (and then trying to fit that into a triangular hole). Inconsistent data formats, missing values, and different time zones are just a few of the obstacles that can trip up even the most experienced analysts. Data silos, where data is trapped in isolated systems, further complicate matters. Consider the case of a bank trying to analyze customer behavior: data from its core banking system might not easily integrate with data from its CRM or fraud detection systems. The resulting picture is fragmented and unreliable, akin to trying to assemble a jigsaw puzzle with half the pieces missing.
Data Cleaning and Preprocessing Techniques
Data cleaning is the unglamorous but essential process of identifying and correcting errors in your data. Imagine it as spring cleaning for your data – getting rid of the dust bunnies (outliers), fixing broken furniture (inconsistent formats), and generally making everything presentable. This might involve handling missing values (imputation techniques, like using the mean or median), smoothing noisy data (applying moving averages), and standardizing data formats (converting dates to a consistent format). For example, standardizing currency formats across various transaction records ensures that the total spending is calculated correctly. A seemingly simple task, but a crucial one for the integrity of your analysis. Failure to do so could lead to inaccurate conclusions, which is why this is a crucial part of the process.
Risk Management Applications
Data analytics has revolutionized financial risk management, transforming it from a largely reactive process to a proactive, data-driven discipline. Gone are the days of relying solely on gut feelings and historical averages; now, sophisticated algorithms and powerful visualization tools allow financial institutions to identify, assess, and mitigate risks with unprecedented accuracy and speed. This shift has not only improved profitability but also enhanced the stability and resilience of the entire financial ecosystem. Let’s delve into the fascinating world of how data analytics tackles financial risks.
Data analytics tools are employed across various risk categories, including credit risk, market risk, and operational risk. In credit risk management, for instance, machine learning models analyze vast datasets of borrower information – credit history, income, debt-to-income ratios, and even social media activity – to predict the likelihood of default. This allows lenders to make more informed lending decisions, optimize pricing, and minimize potential losses. Similarly, in market risk management, these tools analyze market trends, economic indicators, and historical data to model potential portfolio losses and develop effective hedging strategies. Operational risk, encompassing everything from internal fraud to system failures, also benefits from data analytics; by identifying patterns and anomalies in transaction data, institutions can proactively address vulnerabilities and prevent costly disruptions.
Credit Risk Assessment Using Machine Learning
Machine learning algorithms, particularly those based on techniques like logistic regression and random forests, are exceptionally effective in credit scoring. These models analyze numerous variables to generate a credit score that predicts the probability of default with significantly higher accuracy than traditional methods. For example, a bank might use a machine learning model to assess the creditworthiness of small business loan applicants. The model would consider factors such as the applicant’s revenue, expenses, credit history, industry, and even macroeconomic indicators. By analyzing these factors, the model can provide a more accurate prediction of the applicant’s likelihood of default, enabling the bank to make more informed lending decisions and reduce its risk exposure. This leads to a reduction in non-performing loans and improved profitability.
Market Risk Management with Monte Carlo Simulations
Monte Carlo simulations are a powerful tool for assessing market risk. These simulations involve generating thousands of possible future scenarios based on historical data and probabilistic models. By running these simulations, financial institutions can estimate the potential range of portfolio returns under various market conditions. For example, an investment firm might use Monte Carlo simulations to assess the risk of its equity portfolio. The simulation would consider factors such as the volatility of individual stocks, correlations between stocks, and potential market downturns. By running the simulation many times, the firm can generate a distribution of potential portfolio returns, providing a clearer picture of the portfolio’s risk profile. This information can then be used to make informed decisions about portfolio diversification and risk management strategies.
Hypothetical Scenario: Operational Risk and Anomaly Detection
Imagine a large online brokerage experiencing a sudden surge in unusual login attempts from a specific geographic region. This could signal a potential coordinated cyberattack aimed at compromising customer accounts. A tool like Splunk, known for its powerful log analysis capabilities, could be employed to analyze security logs, network traffic data, and other relevant data sources to identify the source of these suspicious activities. By correlating various data points, Splunk could pinpoint the exact nature of the attack, the affected accounts, and potentially even the perpetrators. This early detection and rapid response, facilitated by data analytics, could prevent significant financial losses and reputational damage.
Regulatory Compliance and Data Security
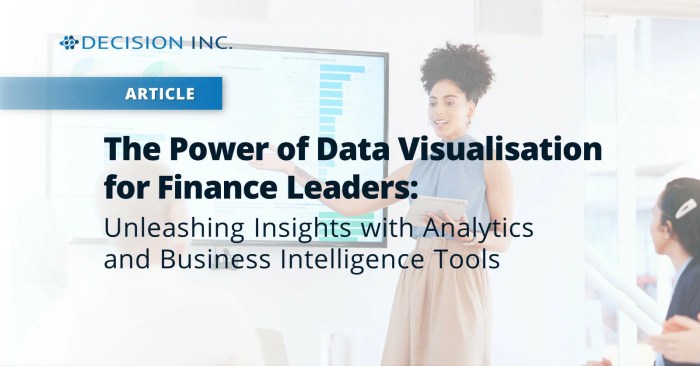
Navigating the wild west of financial data analytics requires more than just a trusty lasso and a keen eye for trends; it demands a healthy respect for the law and a fortress-like approach to data security. Ignoring these aspects can lead to more than just a few bruised egos – it can result in hefty fines and reputational damage that would make even the most seasoned cowboy hang up their spurs.
The financial industry is a heavily regulated landscape, and the use of data analytics is no exception. Regulations like GDPR (in Europe), CCPA (in California), and various others around the globe dictate how financial institutions can collect, store, process, and utilize customer data. These regulations often focus on transparency, consent, data minimization, and the right to be forgotten – concepts that might sound like something from a futuristic utopia, but are very much the present-day reality for finance professionals. Non-compliance can lead to significant penalties, impacting not just the bottom line but also the public’s trust.
Data Security and Privacy in Financial Data Analytics
Data security and privacy are paramount in financial data analytics. The sensitive nature of financial information—including personally identifiable information (PII), transaction details, and account balances—requires robust security measures to prevent unauthorized access, use, disclosure, disruption, modification, or destruction. A breach could not only expose sensitive customer data but also lead to significant financial losses and reputational harm, potentially crippling a financial institution. Think of it as guarding Fort Knox, except instead of gold, you’re protecting the financial well-being of countless individuals and businesses.
Best Practices for Ensuring Compliance and Protecting Sensitive Financial Data
Implementing robust data security and privacy measures is crucial for compliance. This includes establishing comprehensive data governance policies, implementing strong access controls, employing encryption techniques for data at rest and in transit, regularly conducting security audits and penetration testing, and establishing incident response plans. Think of it as building a Swiss bank-level security system for your data, complete with multiple layers of protection and a well-trained security team constantly monitoring for threats. Further, regular employee training on data security best practices and awareness of relevant regulations is essential. Keeping your team up-to-date on the latest threats and compliance requirements is akin to giving them the best armor and weapons in the fight against data breaches. Finally, consider implementing data loss prevention (DLP) tools to monitor and prevent sensitive data from leaving the organization’s control. This is your final line of defense, ensuring that even if a breach occurs, the damage is minimized.
Future Trends in Financial Data Analytics Tools
The world of finance is undergoing a thrilling metamorphosis, fueled by the relentless march of technology. Data analytics tools, once the exclusive domain of spreadsheet wizards and number-crunching gurus, are evolving at warp speed, promising both unprecedented opportunities and, let’s be honest, a few delightfully chaotic surprises. The future of financial data analytics is less about crunching numbers and more about harnessing the power of intelligent algorithms to make sense of the ever-expanding universe of financial data.
The convergence of artificial intelligence (AI), machine learning (ML), and cloud computing is reshaping the financial landscape in profound ways. These technologies are no longer futuristic fantasies; they are actively transforming how financial institutions operate, manage risk, and serve their clients. The implications are vast, impacting everything from fraud detection to algorithmic trading and personalized financial advice. We’re moving from reactive analysis to proactive prediction, and the results are, to put it mildly, mind-boggling.
Artificial Intelligence and Machine Learning in Finance
AI and ML are rapidly becoming indispensable tools in the financial industry. AI algorithms can sift through massive datasets, identifying patterns and anomalies that would be impossible for humans to detect. This capability is revolutionizing fraud detection, allowing institutions to proactively identify and prevent fraudulent transactions in real-time. Machine learning models, trained on historical data, can predict market trends with increasing accuracy, informing investment strategies and risk management decisions. For example, a leading investment bank might use an ML model to predict stock price movements, enabling them to optimize their trading algorithms and potentially increase profitability. Imagine a world where market crashes are predicted weeks in advance – that’s the power of AI and ML at play.
Cloud Computing and Big Data Analytics
The sheer volume of financial data generated daily is staggering. Cloud computing provides the necessary infrastructure to store, process, and analyze this big data efficiently. Cloud-based platforms offer scalability and flexibility, allowing financial institutions to adapt to changing data volumes and computational demands. Furthermore, cloud computing fosters collaboration, enabling different departments and even external partners to access and share data seamlessly. A hypothetical scenario: imagine a hedge fund utilizing a cloud-based platform to analyze global market data in real-time, enabling them to make split-second trading decisions based on the most up-to-date information. This level of speed and access is simply not possible with traditional on-premise solutions.
Predictive Analytics and Risk Management
The ability to predict future outcomes is a holy grail for the financial industry. Advanced analytics techniques, fueled by AI and ML, are enabling more accurate and sophisticated risk management. For example, credit scoring models are becoming increasingly sophisticated, leveraging alternative data sources to assess creditworthiness more accurately. This leads to better loan approvals, reduced defaults, and ultimately, a healthier financial system. Similarly, sophisticated models are being used to predict market volatility and systemic risk, allowing financial institutions to better manage their exposure and protect themselves from potential losses. The recent improvements in predicting economic downturns, though still imperfect, demonstrate the power of these predictive tools.
The Rise of Explainable AI (XAI)
While the predictive power of AI is impressive, understanding *why* a model makes a particular prediction is crucial, especially in the highly regulated financial industry. Explainable AI (XAI) focuses on creating transparent and interpretable AI models. This is vital for regulatory compliance and building trust. Imagine a scenario where an AI model rejects a loan application. With XAI, financial institutions can provide clear explanations for the decision, ensuring fairness and transparency. This addresses concerns about bias and discrimination inherent in traditional and less transparent models.
Ultimate Conclusion
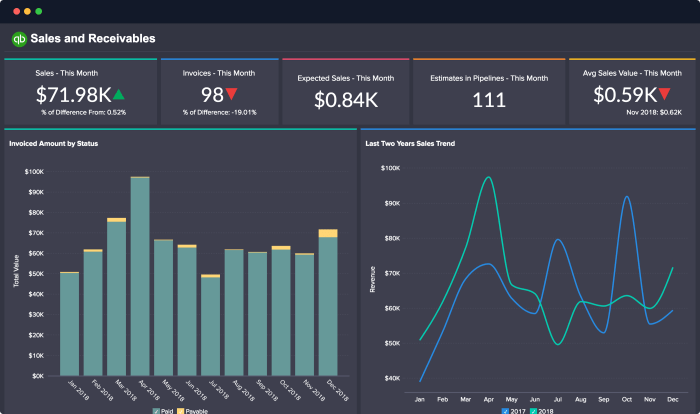
So, there you have it – a comprehensive (and hopefully, amusing) look at the data analytics tools revolutionizing the world of finance. From the historical context to the bleeding-edge advancements, we’ve covered the gamut. While the world of finance might sometimes feel like navigating a minefield of spreadsheets, these tools offer a powerful path towards informed decision-making and – let’s be honest – a significantly less headache-inducing experience. Now go forth and conquer those datasets!
Detailed FAQs: Data Analytics Finance Tools Review
What are the ethical considerations of using AI in financial data analytics?
Ethical considerations are paramount. Bias in algorithms, data privacy concerns, and the potential for misuse (like algorithmic trading gone rogue) need careful attention. Robust oversight and transparent processes are crucial.
How much does it cost to implement these tools?
Costs vary wildly depending on the tool, its features, the scale of implementation, and required training. Expect a range from free open-source options to enterprise solutions costing tens of thousands (or even millions) annually.
What level of technical expertise is needed?
It depends on the tool and the complexity of the tasks. Some tools offer user-friendly interfaces, while others require advanced programming skills (think Python wizards!). The learning curve varies greatly.