Economic Forecasting Methods Review: Predicting the future of economies is a fool’s errand, some might say, akin to predicting the trajectory of a particularly caffeinated squirrel. Yet, the attempt is made daily, by policymakers wrestling with inflation, businesses hedging their bets, and economists desperately trying to avoid egg on their faces. This review delves into the fascinating, and often frustrating, world of economic forecasting, exploring both the sophisticated techniques and the inherent limitations of peering into the crystal ball of the global economy.
From the qualitative musings of expert panels to the quantitative crunching of econometric models, we’ll explore a range of methods, examining their strengths, weaknesses, and applicability in different contexts. We’ll dissect time series analysis, grapple with the complexities of regression, and even peek into the exciting potential of machine learning in this field. Prepare yourself for a rollercoaster ride through data, models, and the ever-elusive quest for economic certainty.
Introduction to Economic Forecasting Methods
Economic forecasting, the crystal ball gazing of the economics world, attempts to peer into the future and predict the performance of an economy. It’s a fascinating blend of art and science, relying on statistical models, historical data, and a healthy dose of educated guesswork. While not always accurate (more on that later!), it plays a crucial role in shaping policy and business decisions.
Economic forecasting is fundamentally important for both policymakers and businesses. For policymakers, accurate forecasts inform decisions on fiscal and monetary policy, impacting everything from interest rates to government spending. Imagine trying to craft a national budget without any idea of future tax revenues – it would be utter chaos! For businesses, forecasting helps with strategic planning, investment decisions, inventory management, and more. A company that accurately predicts a surge in demand can avoid stockouts and capitalize on the opportunity; conversely, a company blindsided by a downturn might find itself facing losses.
The inherent limitations of economic forecasting methods are significant. The economy is an incredibly complex system, influenced by countless interacting factors – from consumer confidence to global events, technological advancements to unpredictable natural disasters. No model can perfectly capture this complexity. Furthermore, unforeseen events (think Black Swan events!) can drastically alter the economic landscape, rendering even the most sophisticated forecasts obsolete. Data limitations, biases in data collection, and the inherent uncertainty of human behavior further contribute to the challenges. It’s a bit like predicting the weather – you can get pretty close sometimes, but surprises are always possible.
Forecasting Horizons and Applications
The timeframe of a forecast significantly influences its methodology and application. Different horizons require different approaches and yield different levels of precision.
Forecasting Horizon | Typical Timeframe | Typical Applications | Example |
---|---|---|---|
Short-Term | Up to 1 year | Inventory management, short-term investment strategies, near-term production planning | A retailer forecasting demand for holiday gifts in the next quarter. |
Medium-Term | 1-5 years | Capital budgeting, strategic planning, medium-term investment decisions, government budget planning | A company deciding whether to invest in a new factory expansion. |
Long-Term | 5+ years | Long-term investment planning, infrastructure projects, demographic forecasting, long-term economic growth projections | A government planning for infrastructure upgrades over the next decade. |
Qualitative Forecasting Methods
Predicting the future is a tricky business, especially in the unpredictable world of economics. While quantitative methods rely on hard numbers and statistical models (think complex equations and spreadsheets galore!), qualitative methods embrace the fuzzier, more intuitive side of forecasting. These methods tap into the wisdom of crowds, expert insights, and even gut feelings – all in the noble pursuit of peering into the economic crystal ball. It’s less about precise calculations and more about informed judgment calls.
Qualitative forecasting techniques, while seemingly less rigorous, offer valuable insights, particularly when dealing with complex, novel situations where historical data is scarce or unreliable. They provide a crucial counterpoint to quantitative models, offering a different perspective and potentially revealing blind spots in purely numerical approaches. Think of them as the seasoned detective’s intuition, complementing the forensic scientist’s meticulous analysis.
Expert Opinion and Delphi Methods
Expert opinion methods leverage the knowledge and experience of individuals deemed experts in a particular field. These experts provide their forecasts based on their understanding of the economic landscape. Strengths include the ability to incorporate qualitative factors and insights that are difficult to quantify. Weaknesses, however, include potential biases, differing interpretations, and the risk of groupthink. The Delphi method attempts to mitigate these weaknesses by using a structured, iterative process involving anonymous feedback and expert revisions, ultimately leading to a more refined consensus. A real-world example would be using a panel of economists to predict the impact of a new government policy on inflation. The strengths of the Delphi method lie in its ability to reduce bias through anonymity and iteration, resulting in a more robust and well-considered forecast. However, the process can be time-consuming and expensive, and it still relies on the expertise and judgment of the participating individuals.
Comparison of Qualitative and Quantitative Forecasting Techniques
Quantitative forecasting relies heavily on historical data and statistical modeling, aiming for objective and numerical predictions. Examples include time series analysis and econometric modeling. Qualitative forecasting, on the other hand, relies on subjective judgments and expert opinions, offering a more nuanced and contextual understanding. While quantitative methods excel in situations with readily available historical data and stable patterns, qualitative methods are more appropriate when dealing with novel events, emerging trends, or scenarios where historical data is limited or unreliable. For example, predicting the impact of a disruptive technology like artificial intelligence on the job market would be better suited to a qualitative approach, leveraging expert opinions and scenario planning, rather than relying solely on past trends.
Situations Where Qualitative Methods Are Most Appropriate
Qualitative methods shine in situations characterized by high uncertainty, limited historical data, or the need to incorporate non-numerical factors. Forecasting the impact of a major geopolitical event, predicting consumer behavior in response to a novel marketing campaign, or assessing the potential market for a groundbreaking new product are all scenarios where qualitative methods are particularly well-suited. These methods provide a valuable framework for understanding the “why” behind potential economic outcomes, adding context and depth to purely numerical predictions. For instance, forecasting the success of a new sustainable energy initiative would benefit from incorporating qualitative factors like public perception, government policies, and technological feasibility alongside quantitative data on energy consumption.
Key Considerations When Using Qualitative Forecasting Techniques
The success of qualitative forecasting hinges on careful planning and execution. Several key considerations must be addressed:
- Expert Selection: Choosing experts with relevant expertise and diverse perspectives is crucial to avoid bias and ensure a well-rounded assessment.
- Bias Mitigation: Implementing techniques to minimize biases, such as anonymous feedback and structured questionnaires, is essential for objective results.
- Data Triangulation: Combining qualitative findings with quantitative data, where possible, can enhance the reliability and validity of the forecasts.
- Scenario Planning: Developing multiple scenarios based on different assumptions can help prepare for a wider range of possible outcomes.
- Transparency and Communication: Clearly documenting the methodology and assumptions used is essential for transparency and effective communication of the findings.
Quantitative Forecasting Methods
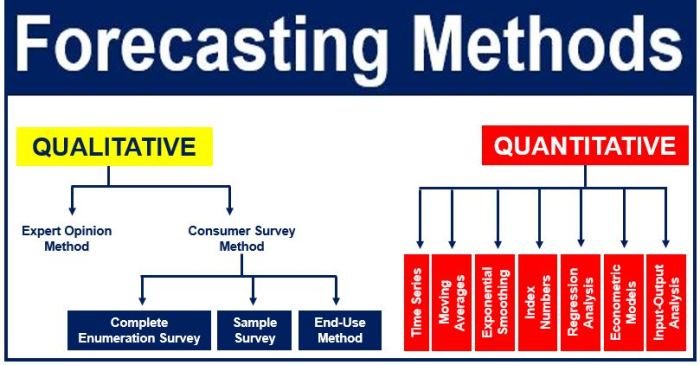
Predicting the future is a tricky business, like trying to catch smoke with a net. But in economics, we need to give it a good shot. Quantitative forecasting methods offer a more structured approach than their qualitative counterparts, relying on hard numbers and statistical techniques. Think of it as using a highly-tuned radar instead of relying on gut feeling. This section dives into the fascinating world of time series analysis, where we hunt down patterns in economic data to anticipate what’s coming next.
Autoregressive Integrated Moving Average (ARIMA) Models
ARIMA models are the workhorses of time series analysis, a bit like a Swiss Army knife for economic forecasting. They’re based on the idea that past values of a variable can help predict its future values. Imagine predicting the price of a certain stock based on its past price fluctuations – that’s essentially what ARIMA models do, but with more mathematical rigor. The model’s parameters (p, d, q) determine the order of the autoregressive (AR), integrated (I), and moving average (MA) components. The ‘p’ represents the number of lagged values of the variable included, ‘d’ represents the degree of differencing required to make the series stationary (meaning the statistical properties don’t change over time), and ‘q’ represents the number of lagged forecast errors. Finding the optimal (p, d, q) combination often involves trial and error and various diagnostic tests to ensure model adequacy. For instance, a model might use the past three months’ sales figures (p=3) after differencing the data once (d=1) to account for a trend and then incorporate the average of the past two forecast errors (q=2). The complexity increases with higher values of p, d, and q.
Exponential Smoothing Methods
Exponential smoothing is a simpler, yet often surprisingly effective, forecasting technique. Unlike ARIMA, which considers multiple past values, exponential smoothing assigns exponentially decreasing weights to older data points. The most recent data points have the most influence on the forecast, while older data points gradually fade into the background. Think of it as giving more weight to recent customer reviews than older ones when assessing a product’s popularity. Several variations exist, including simple exponential smoothing (suitable for stable data), double exponential smoothing (for data with a trend), and triple exponential smoothing (for data with a trend and seasonality). The smoothing constant, denoted by α (alpha), controls the responsiveness of the forecast to recent changes; a higher α gives more weight to recent data, potentially leading to faster responses to shifts but also greater volatility in forecasts. For example, if we’re forecasting daily website traffic, a high alpha would make the forecast quickly adapt to sudden spikes or dips in traffic.
Interpreting Time Series Analysis Results
Interpreting the results of a time series analysis involves more than just looking at the predicted values. We need to assess the model’s goodness of fit, which tells us how well the model represents the actual data. Key metrics include the Mean Absolute Error (MAE), Mean Squared Error (MSE), Root Mean Squared Error (RMSE), and Mean Absolute Percentage Error (MAPE). Lower values indicate better accuracy. Additionally, we should examine diagnostic plots (like residual plots) to check for any patterns or heteroscedasticity (unequal variance) in the residuals (the differences between actual and predicted values). Patterns in the residuals suggest that the model is not capturing all the important information in the data, which means we might need a more sophisticated model or consider other factors. A good model will exhibit randomly scattered residuals with a mean of zero.
Comparison of Time Series Models
The choice of the best time series model depends on the data’s characteristics and the desired level of accuracy. Here’s a comparison:
Model | Complexity | Accuracy | Suitability |
---|---|---|---|
Simple Exponential Smoothing | Low | Moderate | Stable data with no trend or seasonality |
Double Exponential Smoothing | Medium | High | Data with a trend |
Triple Exponential Smoothing | High | High | Data with a trend and seasonality |
ARIMA | High | Potentially very high | Complex data with various patterns; requires careful model selection |
Quantitative Forecasting Methods
Predicting the future of the economy is a bit like predicting the weather – sometimes you get it spectacularly right, and other times you end up with a picnic basket in a hurricane. Econometric models, however, offer a more structured approach than simply gazing into a crystal ball (though we wouldn’t rule that out entirely if it worked). They use statistical methods to analyze economic data and forecast future trends. Think of them as the sophisticated, data-driven cousins of your average fortune cookie.
Regression analysis is the workhorse of econometric modeling. It allows us to examine the relationship between different economic variables, helping us understand how changes in one variable might influence another. For example, we might use regression to analyze the relationship between consumer spending and interest rates – a higher interest rate might lead to less borrowing and thus, lower spending. The beauty of regression is its ability to tease out these complex relationships from a jumble of data, revealing patterns that might otherwise remain hidden. It’s like finding a perfectly organized sock drawer in a chaotic room – unexpectedly delightful!
Regression Analysis in Economic Forecasting
Regression analysis forms the cornerstone of econometric modeling by quantifying the relationships between variables. A simple linear regression, for example, might model the relationship between GDP growth (Y) and inflation (X) as:
Y = β₀ + β₁X + ε
where β₀ is the intercept, β₁ represents the effect of inflation on GDP growth, and ε is the error term accounting for unpredictable factors. More complex models incorporate multiple independent variables and account for non-linear relationships. The coefficients (β₀ and β₁) are estimated using historical data, and these estimates are then used to predict future GDP growth based on forecasts of inflation. For instance, if historical data suggests that a 1% increase in inflation leads to a 0.5% decrease in GDP growth (β₁ = -0.5), we can use this relationship to predict future GDP growth given an inflation forecast. This predictive power allows policymakers to make informed decisions about monetary policy or fiscal stimulus.
Building and Validating an Econometric Model
Constructing a robust econometric model is a meticulous process. It begins with clearly defining the economic variable to be predicted (the dependent variable) and identifying potential influencing factors (independent variables). This selection process often involves economic theory and prior research. Next, we gather relevant historical data for all selected variables. Then, the chosen regression model is estimated using statistical software. The model’s performance is then rigorously evaluated using various statistical measures, including R-squared, adjusted R-squared, and diagnostic tests for heteroskedasticity and autocorrelation. Crucially, the model must be validated using out-of-sample data – data not used in the estimation process – to assess its predictive accuracy in unseen circumstances. This is like testing a new recipe on a group of friends who haven’t tasted it before. If it fails the out-of-sample test, we go back to the drawing board, perhaps refining variable selection or model specification.
Potential Biases and Limitations of Econometric Models
Despite their sophistication, econometric models are not infallible. One major concern is omitted variable bias. If we fail to include relevant variables, our estimates of the included variables’ effects might be biased. This is akin to trying to bake a cake without flour – the result will be less than stellar. Another limitation is the assumption of linearity. Economic relationships are often non-linear, and forcing a linear model onto non-linear data can lead to inaccurate predictions. Furthermore, structural breaks – sudden shifts in the underlying economic relationships – can render a model obsolete. Finally, forecasting inherently involves uncertainty, and econometric models only provide probabilistic predictions, not certainties. Remember, even the most sophisticated model can’t predict the next economic earthquake with perfect accuracy.
Hypothetical Econometric Model: Predicting Housing Prices
Let’s consider a model predicting average house prices (dependent variable) in a specific city. Independent variables could include average household income, interest rates, local property taxes, and the number of new housing units constructed. The model could be specified as:
House Price = β₀ + β₁ (Household Income) + β₂ (Interest Rates) + β₃ (Property Taxes) + β₄ (New Housing Units) + ε
. This model assumes a linear relationship between house prices and the independent variables. Estimating this model using historical data would yield coefficient estimates (β₁, β₂, β₃, β₄), which represent the effect of each independent variable on house prices. For instance, a positive β₁ suggests that higher household incomes lead to higher house prices, while a positive β₂ suggests that higher interest rates lead to lower house prices. To validate this model, we’d test its predictive power on out-of-sample data, comparing predicted house prices with actual house prices. This process helps us understand the model’s strengths and limitations in predicting housing prices in the future.
Forecasting Specific Economic Variables: Economic Forecasting Methods Review
Predicting the future is a fool’s errand, as they say, but economists, bless their cotton socks, try anyway. Forecasting specific economic variables like inflation, unemployment, and GDP growth presents unique challenges, requiring a blend of sophisticated models and a healthy dose of educated guesswork. The accuracy of these forecasts significantly impacts government policy, business decisions, and even individual financial planning. Let’s dive into the messy, wonderful world of economic forecasting specifics.
Inflation Forecasting
Inflation, that insidious creep of rising prices, is notoriously difficult to predict accurately. The complexities of supply chains, global events, and consumer behavior create a volatile environment. Traditional methods, such as using past inflation rates (a simple moving average, for instance), often fall short. More advanced techniques incorporate leading indicators like commodity prices, producer price indices (PPIs), and surveys of consumer expectations. For example, the Federal Reserve utilizes a sophisticated model incorporating a wide range of variables to predict inflation, constantly refining it based on new data. A failure to accurately predict inflation can lead to significant economic consequences; for instance, underestimating inflation can erode purchasing power, while overestimating it can lead to unnecessarily tight monetary policy, hindering economic growth.
Unemployment Rate Forecasting
Forecasting unemployment rates requires understanding the intricate interplay between labor market dynamics and the broader economy. Methods range from simple time series analysis, projecting trends based on historical data, to more complex models incorporating factors like job creation, labor force participation rates, and technological advancements. The Okun’s Law, which posits an inverse relationship between unemployment and GDP growth, is frequently used. For example, if GDP growth is projected to be robust, a lower unemployment rate is typically anticipated. However, structural changes in the labor market, such as automation or shifts in industry, can complicate these predictions. Accurate unemployment rate forecasting is crucial for governments designing social safety nets and for businesses planning hiring strategies.
GDP Growth Forecasting
Predicting GDP growth, the overall measure of economic output, is a multifaceted endeavor. Different methodologies exist, each with its strengths and weaknesses. Simple extrapolations of past growth rates are often used as a baseline, but these are rarely sufficient. More sophisticated models incorporate leading indicators like consumer confidence, business investment, and government spending. Input-output models trace the flow of goods and services through the economy, providing a detailed picture of interdependencies. Econometric models, which utilize statistical techniques to estimate relationships between variables, are also commonly employed. For example, the Conference Board’s Leading Economic Index (LEI) is a composite index of several economic indicators designed to predict future economic activity, including GDP growth. Accurate GDP growth forecasts are vital for policymakers in setting fiscal and monetary policies.
Key Indicators for Forecasting Economic Variables
Understanding the key indicators used to forecast these crucial economic variables is essential. The accuracy of predictions hinges on the quality and timeliness of this data.
- Inflation: Consumer Price Index (CPI), Producer Price Index (PPI), Commodity Prices, Wage Growth, Survey of Consumer Expectations, Import Prices.
- Unemployment Rate: Job Creation/Destruction Numbers, Labor Force Participation Rate, Initial Claims for Unemployment Insurance, Quits Rate, Job Openings.
- GDP Growth: Consumer Spending, Business Investment, Government Spending, Net Exports, Housing Starts, Durable Goods Orders, Consumer Confidence Index, Leading Economic Indicators.
Evaluating Forecast Accuracy
Predicting the future of the economy is a bit like predicting the weather – sometimes you get it spectacularly right, and sometimes you’re left wondering if your crystal ball needs a new battery. Evaluating the accuracy of economic forecasts is therefore crucial, not just for bragging rights, but for improving future predictions and making informed decisions. Let’s delve into the fascinating world of forecast evaluation metrics.
Methods for Evaluating Forecast Accuracy
Several methods exist to assess how well our economic forecasts align with reality. These methods quantify the difference between predicted and actual values, allowing for a numerical evaluation of forecasting performance. The choice of method depends on the specific context and the nature of the data.
Mean Absolute Error (MAE)
MAE measures the average absolute difference between the predicted and actual values. It’s easy to understand and calculate, making it a popular choice. The formula is:
MAE = (1/n) * Σ|Actuali – Forecasti|
where ‘n’ is the number of observations, Actuali is the actual value for observation i, and Forecasti is the corresponding forecast. A lower MAE indicates better accuracy. For example, if we’re forecasting GDP growth and our MAE is 0.5%, it means our forecasts are, on average, off by 0.5 percentage points.
Root Mean Squared Error (RMSE)
RMSE, similar to MAE, calculates the average difference between predicted and actual values. However, it squares the differences before averaging them and then takes the square root. This gives more weight to larger errors. The formula is:
RMSE = √[(1/n) * Σ(Actuali – Forecasti)2]
A lower RMSE also indicates better accuracy. Because it penalizes larger errors more heavily than MAE, RMSE is often preferred when large errors have more significant consequences. For instance, in financial markets, a large forecasting error could lead to substantial losses.
Mean Absolute Percentage Error (MAPE)
MAPE expresses the error as a percentage of the actual value. This is useful for comparing forecasts across different scales or units. The formula is:
MAPE = (1/n) * Σ[|Actuali – Forecasti| / Actuali] * 100%
A lower MAPE indicates better accuracy. Imagine forecasting sales figures for different products – using MAPE allows for a direct comparison of forecast accuracy even if the products have vastly different sales volumes.
Comparison of Evaluation Methods
Each method has its strengths and weaknesses. MAE is simple and easy to interpret, while RMSE gives more weight to larger errors. MAPE provides a percentage-based measure, useful for comparisons across different scales. The “best” method depends on the specific application and the priorities of the forecaster. For example, in a situation where large errors are particularly costly, RMSE might be preferred over MAE.
Interpreting Results and Improving Models
Lower values of MAE, RMSE, and MAPE generally indicate better forecast accuracy. However, the interpretation also depends on the context. A low RMSE of 0.1% in forecasting inflation might be excellent, while a low RMSE of 10% in forecasting housing prices might be considered quite poor.
By comparing the accuracy metrics across different forecasting models, we can identify which model performs best. For example, if a model using ARIMA produces a lower RMSE than a model using exponential smoothing, we might favor the ARIMA model. Furthermore, understanding the sources of errors can help refine the model, perhaps by incorporating additional variables or adjusting model parameters.
Illustrative Example of Forecast Accuracy Metrics
Metric | Model A | Model B | Interpretation |
---|---|---|---|
MAE | 2.5 | 1.8 | Model B is more accurate than Model A, as it has a lower MAE. |
RMSE | 3.2 | 2.1 | Similar to MAE, Model B shows better accuracy due to lower RMSE. The larger difference between MAE and RMSE in Model A suggests the presence of some larger errors. |
MAPE | 15% | 10% | Again, Model B demonstrates superior accuracy with a lower MAPE. This means Model B’s percentage errors are smaller on average. |
The Role of Data and Technology in Economic Forecasting
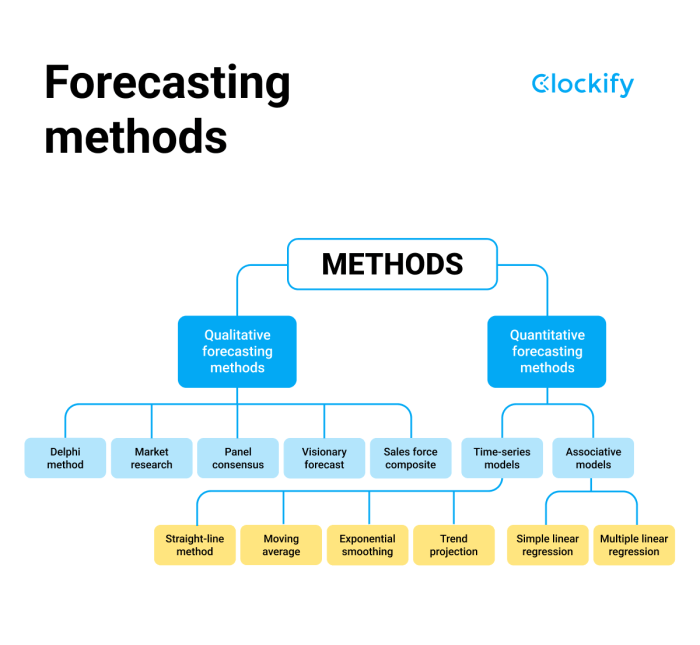
The marriage of economics and technology has yielded some surprisingly adorable offspring – namely, significantly improved economic forecasting. Gone are the days of relying solely on gut feelings and crystal balls (mostly gone, anyway). Now, we have terabytes of data and algorithms that can sift through it faster than you can say “quantitative easing.” This section explores the delightful dance between data, technology, and the increasingly accurate prediction of economic trends.
Big Data’s Impact on Economic Forecasting
The sheer volume, velocity, and variety of data available today – the holy trinity of “Big Data” – has revolutionized economic forecasting. We’re no longer limited to government statistics and quarterly reports. Now, we can incorporate data from social media sentiment, credit card transactions, satellite imagery (think crop yields!), and even electricity consumption to paint a far more nuanced picture of the economic landscape. For example, analyzing search trends for terms like “layoff” or “unemployment benefits” can provide early warnings of potential economic downturns, far exceeding the lag time of traditional indicators. This allows for more timely and effective policy responses.
Machine Learning Algorithms in Economic Forecasting
Machine learning algorithms, particularly those based on artificial neural networks and deep learning, are proving incredibly effective in identifying complex patterns and relationships within vast datasets that would be impossible for human analysts to spot. These algorithms can sift through millions of data points, identifying subtle correlations and predicting future trends with remarkable accuracy. For instance, algorithms have been used to forecast inflation rates more accurately than traditional econometric models, taking into account factors like supply chain disruptions and energy prices with greater granularity. The results are, dare we say, breathtakingly accurate in many cases.
Data Quality and Availability Challenges in Economic Forecasting
While the potential of big data is enormous, the reality is often messier. Data quality is paramount, and inconsistent or inaccurate data can lead to flawed forecasts. Furthermore, access to comprehensive and reliable data can be a significant hurdle, particularly in developing economies or for specific niche sectors. Data silos, differing data definitions, and the ever-present issue of data bias (both conscious and unconscious) can severely limit the effectiveness of even the most sophisticated algorithms. A classic example is the challenge of accurately forecasting housing prices, where data on shadow markets or unrecorded transactions can skew results.
Advancements in Computing Power and Forecasting Capabilities
The exponential growth in computing power has been a game-changer for economic forecasting. Complex algorithms that were once computationally prohibitive are now readily accessible, allowing for the development of more sophisticated models and the processing of larger datasets. Cloud computing has further democratized access to these powerful resources, making advanced forecasting techniques available to a wider range of researchers and practitioners. This increased computational power allows for real-time analysis and forecasting, enabling faster responses to changing economic conditions. Imagine: economic forecasts updated hourly, not quarterly! It’s a beautiful thought, isn’t it?
Future Directions in Economic Forecasting
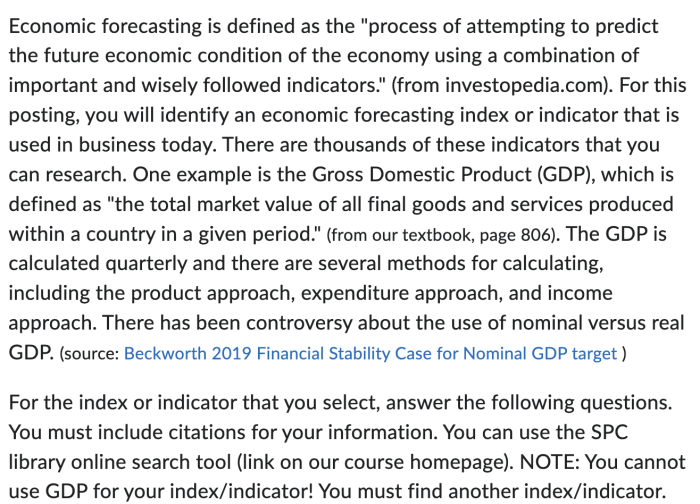
Economic forecasting, while seemingly a dry and dusty pursuit, is actually a thrilling rollercoaster ride of data, analysis, and the occasional spectacular crash. The future of this field is anything but predictable, promising exciting advancements and daunting challenges. We’ll explore the emerging trends that will shape how we peek into the economic crystal ball (which, let’s be honest, is often more cloudy than clear).
The incorporation of behavioral economics promises to inject a much-needed dose of realism into our models. For too long, we’ve assumed perfectly rational actors—a hilarious simplification of human behavior. By acknowledging biases, heuristics, and the occasional bout of irrational exuberance (or despair), we can build models that are less prone to the kind of forecasting blunders that make economists look like…well, economists.
The Rise of Behavioral Economics in Forecasting
Traditional economic models often rely on the assumption of rational agents. However, behavioral economics recognizes the influence of psychological factors, cognitive biases, and emotional responses on economic decision-making. Incorporating these insights can lead to more accurate predictions, particularly in situations involving uncertainty or market sentiment. For instance, a model that accounts for herd behavior might better predict market crashes than one that assumes purely rational investor behavior. The challenge lies in quantifying these behavioral factors and integrating them effectively into existing econometric frameworks. This is a bit like trying to predict the weather by factoring in the moods of individual clouds – challenging, but potentially rewarding.
The Crucial Role of Uncertainty and Risk, Economic Forecasting Methods Review
Predicting the future is inherently uncertain. Ignoring this fundamental truth has led to many forecasting failures. Future advancements will need to focus on explicitly modeling and quantifying uncertainty, perhaps by employing techniques like Bayesian methods or scenario planning. This means moving beyond simple point forecasts (a single prediction) to provide ranges of possible outcomes, along with associated probabilities. Imagine instead of predicting a 2% GDP growth, we predict a range of 1% to 3%, with a higher probability assigned to 2%. This offers a more nuanced and realistic picture. Think of it as providing a weather forecast that includes the probability of sunshine, rain, and hail – a far cry from simply stating “sunny” and being completely wrong when it pours.
Data Science’s Transformative Potential
The sheer volume of data available today, from social media trends to satellite imagery of crop yields, is staggering. Advanced data science techniques, such as machine learning and artificial intelligence, offer the potential to sift through this data deluge and uncover hidden patterns and relationships that traditional methods miss. For example, sentiment analysis of social media posts could provide early warnings of economic shifts. Imagine a system that analyzes millions of tweets daily, identifying shifts in consumer confidence long before traditional economic indicators show a change. This is akin to having a super-powered economic crystal ball that’s constantly being updated with real-time information from the entire planet. The challenge, of course, is in ensuring the accuracy and reliability of these data-driven forecasts, and preventing the algorithms from learning and perpetuating existing biases.
Final Thoughts
So, can we truly predict the future of the economy? The answer, unsurprisingly, is a nuanced “maybe.” While perfect prediction remains a holy grail, the methods reviewed here provide valuable tools for navigating the uncertainties inherent in economic landscapes. By understanding the strengths and limitations of various approaches, policymakers and businesses can make more informed decisions, mitigating risks and capitalizing on opportunities. Ultimately, the journey of economic forecasting is less about achieving infallible predictions and more about refining our understanding of complex systems and improving our ability to make strategic choices in the face of uncertainty – a worthy endeavor indeed.
Top FAQs
What’s the difference between leading, lagging, and coincident indicators?
Leading indicators predict future economic activity (e.g., consumer confidence), lagging indicators confirm past activity (e.g., unemployment rate), and coincident indicators reflect current economic conditions (e.g., industrial production).
How can I choose the best forecasting method for my needs?
The optimal method depends on factors like data availability, forecasting horizon, and the desired level of accuracy. Consider the trade-off between model complexity and predictive power.
What are some common pitfalls to avoid in economic forecasting?
Beware of overfitting models, ignoring data limitations, and placing undue faith in any single forecasting method. Always consider multiple perspectives and potential biases.