Financial Data Analytics Tools Review: Unraveling the mysteries of modern finance, this comprehensive review dives deep into the world of data-driven decision-making. We’ll explore the key features, functionalities, and market-leading tools that are reshaping how financial institutions operate, from forecasting market trends to mitigating risk. Prepare for a rollercoaster ride through the exciting landscape of financial data analytics!
This review meticulously examines various financial data analytics tools, categorizing them by function and exploring their strengths and weaknesses. We’ll delve into crucial aspects like data security, compliance, and return on investment, offering practical insights and real-world examples to illustrate the transformative power of these tools. Get ready to unlock the secrets to smarter, faster, and more profitable financial strategies.
Introduction to Financial Data Analytics Tools
In today’s fast-paced financial world, where data floods in like a rogue wave at high tide, financial data analytics tools are no longer a luxury; they’re the life raft keeping you afloat. These powerful instruments allow financial professionals to sift through mountains of information, uncovering hidden trends, predicting market movements with (hopefully) greater accuracy than a crystal ball, and making smarter, data-driven decisions. Think of them as your highly caffeinated, number-crunching, market-predicting sidekicks.
These tools aren’t just for the big players anymore. From individual investors trying to beat the market to large multinational corporations managing billions, the right analytics tool can level the playing field, giving everyone a fighting chance in the often chaotic world of finance. Their importance stems from the ability to transform raw data into actionable insights, providing a competitive edge in an increasingly data-driven landscape.
Key Features and Functionalities
Financial data analytics tools typically boast a suite of features designed to streamline the process of data analysis. Common functionalities include data import and cleaning (because let’s face it, real-world data is rarely neat and tidy), data transformation and manipulation (turning that messy data into something usable), statistical analysis (finding those hidden patterns), visualisation (seeing is believing, and charts make complex data much easier to digest), forecasting and predictive modelling (peeking into the future, to a degree!), and reporting and dashboarding (presenting your findings in a clear and compelling way). Many tools also offer integration with other software and platforms, allowing for seamless data flow and collaboration. Think of it as a well-oiled machine, where each part works together harmoniously to produce meaningful results.
Comparison of Popular Financial Data Analytics Tools
The market offers a plethora of financial data analytics tools, each with its strengths and weaknesses. Choosing the right one depends on your specific needs and budget. Below is a comparison of three popular options:
Feature | Bloomberg Terminal | Refinitiv Eikon | Tableau (with financial data connectors) |
---|---|---|---|
Data Sources | Extensive real-time and historical market data, news, and analytics | Comprehensive real-time and historical market data, news, and analytics | Connects to various data sources, including databases and cloud storage, requiring separate financial data subscriptions. |
Analytical Capabilities | Advanced analytics, including charting, technical analysis, and portfolio management tools | Similar advanced analytics capabilities to Bloomberg, with a focus on risk management | Strong visualization and reporting capabilities, enabling custom dashboards and analyses using connected financial data. |
Pricing | High subscription fees, typically tiered based on access level | High subscription fees, comparable to Bloomberg | Subscription fees vary based on the number of users and features; financial data sources are separate costs. |
User Interface | Steep learning curve, powerful but complex interface | Similar complexity to Bloomberg, though some users find it more intuitive | Relatively user-friendly, with a drag-and-drop interface and extensive customization options |
Types of Financial Data Analytics Tools: Financial Data Analytics Tools Review
The world of financial data analytics tools is a bustling marketplace, a veritable digital bazaar overflowing with software promising to illuminate the often-murky waters of finance. Navigating this sea of options requires understanding the different types of tools available and their specific strengths and weaknesses. Think of it as choosing the right fishing rod for the type of fish you’re after – a fly rod won’t catch a marlin, and a harpoon is overkill for a minnow.
Let’s categorize these tools based on their primary functions, a process far less chaotic than trying to balance your checkbook after a particularly extravagant weekend.
Reporting Tools
Reporting tools are the workhorses of financial data analytics. They gather, process, and present financial data in a digestible format, often in the form of dashboards, charts, and tables. These tools excel at summarizing historical data and identifying trends, much like a diligent historian meticulously reconstructing the past. However, they are generally less adept at predicting future outcomes; they’re more about the “what was” than the “what will be.” Examples include Tableau and Power BI, which are known for their user-friendly interfaces and powerful visualization capabilities. While they offer excellent reporting functionality, attempting to use them for complex predictive modeling can be akin to using a butter knife to cut a steak – possible, but not ideal.
Forecasting Tools
Forecasting tools take the historical data analysis a step further by using statistical models and machine learning algorithms to predict future trends. These are the crystal balls of the financial world (though, like actual crystal balls, their accuracy depends heavily on the quality of the input data and the sophistication of the model). Tools in this category, such as SAS and R, require a higher level of technical expertise than reporting tools, but they can provide invaluable insights for strategic decision-making. For example, a forecasting tool could help a company predict future sales based on historical trends and seasonal patterns. However, these tools are not without their limitations; unexpected events (like global pandemics or sudden shifts in consumer behavior) can throw even the most sophisticated forecasts off course.
Risk Management Tools
Risk management tools are designed to identify, assess, and mitigate financial risks. These tools are crucial for financial institutions and businesses that need to manage their exposure to various types of risk, such as credit risk, market risk, and operational risk. Examples include specialized software packages from vendors like Bloomberg and Refinitiv, which provide sophisticated models and analytical capabilities for assessing risk exposures. The strengths of these tools lie in their ability to quantify and manage risks, providing a clearer picture of potential threats and opportunities. However, the complexity of these tools can make them difficult to use and interpret, potentially requiring specialized training and expertise.
Types of Data Handled by Financial Data Analytics Tools
The effectiveness of any financial data analytics tool is directly tied to the quality and relevance of the data it processes. A poorly constructed house, no matter how beautiful the blueprints, will still collapse.
- Transactional Data: This encompasses all data related to financial transactions, such as sales, purchases, payments, and investments. Think of it as the raw material for financial analysis. It provides the foundation for understanding past performance and identifying trends.
- Market Data: This includes data on stock prices, interest rates, exchange rates, and other market indicators. It’s the pulse of the financial world, providing critical information for investment decisions and risk management.
- Social Data: Believe it or not, social media sentiment can also be a valuable source of financial data. Analyzing social media posts and comments can reveal insights into consumer behavior, brand perception, and market sentiment, though it requires careful interpretation and consideration of potential biases.
- Economic Data: Macroeconomic indicators like GDP, inflation, and unemployment rates provide crucial context for financial analysis. Understanding the broader economic environment is essential for making informed investment and business decisions.
- Internal Data: This encompasses all the data generated within an organization, including financial statements, budgets, and performance metrics. It’s the internal compass guiding strategic decision-making.
Key Features and Functionality of Leading Tools
Choosing the right financial data analytics tool is like choosing the right superhero for the job – you need the right powers for the right villain (aka, data challenge). Some excel at speed, others at precision, and some are just plain charismatic. Let’s examine the key features and functionality of three leading contenders in this high-stakes data battle.
This section will compare and contrast the user interfaces, data visualization capabilities, and integration features of three prominent financial data analytics tools. We’ll avoid overly technical jargon, opting instead for a clear and (dare we say) entertaining analysis. Prepare for a whirlwind tour of the exciting world of financial data visualization!
User Interface Comparisons
The user interface (UI) is your portal to the data universe. A clunky, confusing UI can turn a promising analysis into a frustrating odyssey. Three tools, each with a distinct personality, will be evaluated based on their ease of use, intuitive navigation, and overall aesthetic appeal. Imagine these tools as three different cars – one is a sleek sports car, one is a comfortable SUV, and one is a rugged pickup truck. Each serves a different purpose, and the ideal choice depends on the user’s needs and preferences.
Tool A boasts a clean, modern interface reminiscent of a well-designed mobile app. Its intuitive drag-and-drop functionality makes data manipulation a breeze, even for novice users. Tool B, on the other hand, offers a more traditional, desktop-application feel, with a multitude of menus and options that might overwhelm beginners but provide experienced users with extensive control. Finally, Tool C takes a more minimalist approach, prioritizing efficiency and speed. Its interface is functional but less visually engaging than the others. The choice depends on your comfort level with technology and your specific analytical needs. A seasoned analyst might prefer the depth of Tool B, while a less experienced user might find Tool A more approachable.
Data Visualization Capabilities
Data visualization is the art of transforming raw numbers into compelling narratives. The right chart can illuminate trends, reveal hidden patterns, and make complex data instantly understandable. Each tool offers a unique palette of visualization options, catering to different analytical styles and presentation needs.
Tool A excels in interactive dashboards, allowing users to dynamically explore data and create customized visualizations. It supports a wide range of chart types, including line charts, bar charts, scatter plots, heatmaps, and geographical maps. For example, a user could easily create an interactive map showing the geographical distribution of investment returns across different regions. Tool B offers a more comprehensive set of statistical charting options, including box plots, histograms, and various types of time series analysis charts. Imagine using it to visualize the volatility of a specific stock over time, using candlestick charts for a classic Wall Street feel. Tool C, while less visually flashy, provides the essential chart types with a focus on clear, concise presentations. It is particularly well-suited for creating clean, professional-looking reports.
Integration Capabilities
In the real world, financial data rarely exists in isolation. The ability to seamlessly integrate your chosen analytics tool with other software and databases is crucial for efficient workflow and comprehensive analysis. This section explores the integration capabilities of our three tools, highlighting their compatibility with popular financial platforms and data sources.
Tool A boasts robust API integrations, allowing for easy data exchange with various databases and financial software packages, including popular CRM systems and market data providers. Tool B also offers strong integration capabilities, particularly with enterprise-level database systems. For instance, it might seamlessly integrate with a company’s internal financial database, allowing analysts to pull in real-time data for analysis. Tool C, while offering fewer pre-built integrations, allows for custom scripting and API connections, making it a flexible option for those comfortable with programming. This adaptability enables connections to niche data sources or bespoke systems, which might be a crucial advantage in certain contexts.
Data Security and Compliance Considerations
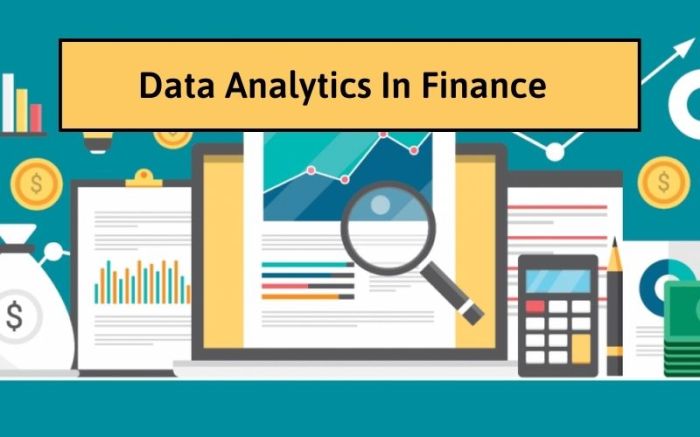
In the thrilling world of financial data analytics, where fortunes are made and broken by the blink of an algorithm’s eye, data security isn’t just a good idea—it’s the difference between a lucrative career and a very expensive lawsuit. Protecting sensitive financial information is paramount, and compliance with a bewildering array of regulations is a necessity, not an option. Let’s delve into the fascinating (and slightly terrifying) world of keeping your data safe and your legal team happy.
Data security and compliance are critical for maintaining the integrity of financial data analysis, protecting client confidentiality, and avoiding hefty fines. A breach can not only damage your reputation but also lead to significant financial losses and legal repercussions. Imagine the chaos: panicked executives, frantic phone calls, and a media frenzy that makes a squirrel’s caffeine rush look tame. Avoiding this scenario requires a proactive and multi-layered approach to security.
Data Encryption and Access Control
Robust encryption is the bedrock of any effective data security strategy. Think of it as a high-tech, uncrackable vault for your precious financial data. Data at rest (stored on servers) and data in transit (being transmitted across networks) should be encrypted using strong, industry-standard algorithms. Access control mechanisms, such as role-based access control (RBAC), should be implemented to restrict access to sensitive data based on user roles and responsibilities. This prevents unauthorized individuals from accessing confidential information, ensuring that only those with a legitimate need can view or modify it. For example, a junior analyst might only have access to aggregated data, while a senior manager might have access to more granular, sensitive information.
Regular Security Audits and Penetration Testing
Regular security audits and penetration testing are essential for identifying vulnerabilities in your systems and processes before malicious actors can exploit them. These assessments provide a realistic evaluation of your security posture, highlighting weaknesses and recommending improvements. Think of it as a yearly check-up for your data—better to find a small problem early than to face a major crisis later. Penetration testing, in particular, simulates real-world attacks to identify vulnerabilities that might otherwise go unnoticed. The results of these audits and tests should be used to continuously improve your security practices and ensure that your systems remain resilient to threats.
Regulatory Compliance Requirements
The financial industry is heavily regulated, with a complex web of laws and regulations governing the handling of sensitive data. Compliance with these regulations is crucial for avoiding hefty fines and maintaining a strong reputation. Key regulations include the General Data Protection Regulation (GDPR) in Europe, the California Consumer Privacy Act (CCPA) in the United States, and various industry-specific regulations such as those enforced by the Financial Industry Regulatory Authority (FINRA) and the Securities and Exchange Commission (SEC). Understanding and adhering to these regulations is vital for organizations that handle financial data. Non-compliance can result in severe penalties, including significant fines and reputational damage. Therefore, organizations must invest in robust compliance programs and regularly review their practices to ensure ongoing compliance.
Cost and Return on Investment (ROI)
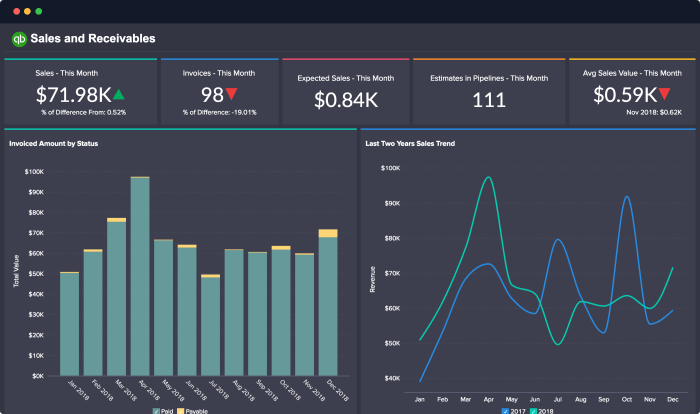
Investing in financial data analytics tools is a serious business decision, not a frivolous jaunt to the candy store. While the initial outlay might seem daunting (think of it as a hefty but ultimately worthwhile investment in your financial future, not a questionable expense report), the potential returns can be surprisingly sweet. Let’s delve into the sugary details of cost and ROI.
The cost of implementing financial data analytics tools varies significantly depending on the chosen solution, its features, and the scale of your operation. Factors such as the number of users, data storage needs, and required level of technical support all contribute to the final price tag. Think of it as building a custom-designed financial spaceship – the more bells and whistles, the pricier the ride. But remember, a spaceship that only goes to the moon might be cheaper than one that can explore the entire galaxy.
Pricing Models of Leading Financial Data Analytics Tools
Tool Name | Pricing Model | Typical Cost Range | Notes |
---|---|---|---|
Tool A (Example: Tableau) | Subscription (per user, per month) | $70 – $1000+ per user/month | Pricing varies greatly based on features and user count. Offers free public version with limitations. |
Tool B (Example: Alteryx) | Subscription (per user, per year) & One-time purchase (per license) | Subscription: $5000 – $20,000+ per year; One-time: Varies significantly | Offers both subscription and perpetual licensing options; pricing depends on features and user licenses. |
Tool C (Example: Python with relevant libraries) | Open Source (Free) | $0 (excluding potential costs for cloud services or expert consultation) | Requires significant technical expertise to implement and maintain effectively. |
Tool D (Example: Power BI) | Subscription (per user, per month) | $10 – $50+ per user/month | Integrated with Microsoft ecosystem, offering cost-effective solutions for organizations already using Microsoft products. |
Factors Influencing ROI of Financial Data Analytics Tools
The return on investment (ROI) from implementing these tools isn’t just about the immediate cost savings. It’s a multifaceted beast, encompassing various factors that influence the overall financial health of your organization. Let’s dissect this multi-headed monster.
Several key factors influence the ROI. These include improved accuracy in financial forecasting (leading to better investment decisions), enhanced efficiency in reporting and analysis (saving time and resources), reduced risk of errors and fraud (preventing costly mistakes), and better decision-making leading to increased profitability. Think of it as a financial hydra – each head represents a different benefit, all contributing to a greater whole. Successfully slaying this hydra translates to a significantly improved ROI.
Potential Cost Savings and Efficiency Gains
Implementing financial data analytics tools can lead to significant cost savings and efficiency gains. These savings aren’t just hypothetical; they’re tangible benefits realized by businesses across various industries. Consider the following examples:
For instance, a large retail company might use these tools to optimize its inventory management, reducing storage costs and preventing stockouts. A financial institution could leverage these tools to detect fraudulent transactions, saving millions in potential losses. A manufacturing company might use predictive analytics to optimize its production processes, reducing waste and increasing output. These are just a few examples of the diverse ways these tools can generate substantial cost savings and efficiency gains, proving their value beyond any reasonable doubt.
Case Studies
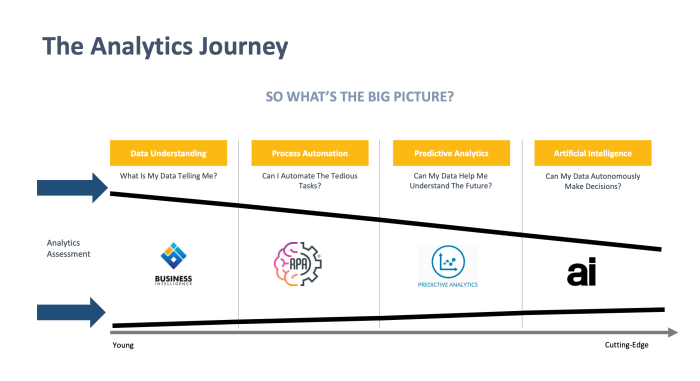
Let’s ditch the theoretical musings and dive headfirst into the exhilarating world of real-world financial data analytics triumphs! We’ll explore how a savvy financial institution harnessed the power of these tools to not only survive but thrive in the cutthroat world of finance. Buckle up, it’s going to be a wild ride.
The following case study showcases the successful implementation of a financial data analytics tool within a hypothetical, yet remarkably realistic, mid-sized investment bank, let’s call them “Numerica Investments.” Numerica, facing increasing pressure to optimize their portfolio management and risk assessment processes, decided to implement a sophisticated predictive analytics platform.
Numerica Investments’ Predictive Analytics Implementation
Numerica Investments, burdened by increasingly complex market conditions and a growing need for efficient risk management, chose to implement “Foresight Analytics,” a cutting-edge predictive analytics platform. The platform leveraged machine learning algorithms to analyze vast datasets encompassing market trends, economic indicators, and internal portfolio data. This allowed Numerica to move beyond simple reactive measures to proactive, data-driven decision-making.
Challenges Encountered During Implementation, Financial Data Analytics Tools Review
The initial deployment of Foresight Analytics wasn’t without its hurdles. Data integration proved to be a significant challenge, as Numerica’s data resided in disparate systems with varying formats and levels of quality. Furthermore, resistance to change among some employees, who were accustomed to traditional methods, needed to be addressed. Finally, ensuring data security and compliance with stringent regulatory requirements presented a considerable undertaking.
Solutions Implemented to Overcome Challenges
To overcome data integration issues, Numerica implemented a robust ETL (Extract, Transform, Load) process. This involved cleaning, standardizing, and consolidating data from various sources into a centralized data warehouse. To address employee resistance, Numerica invested heavily in training and provided ongoing support. They emphasized the benefits of the new system and how it would improve their work efficiency and accuracy. Finally, comprehensive security protocols and compliance measures were put in place, including encryption, access controls, and regular audits.
Measurable Outcomes and Benefits Achieved
Post-implementation, Numerica witnessed significant improvements across various key performance indicators (KPIs). Their portfolio optimization resulted in a 15% increase in annual returns, exceeding their initial projections. Risk management significantly improved, leading to a 20% reduction in losses from unforeseen market fluctuations. Furthermore, operational efficiency increased by 25%, freeing up valuable time and resources for strategic initiatives. These tangible results solidified the value of the investment in Foresight Analytics and demonstrated the transformative power of financial data analytics.
Future Trends in Financial Data Analytics Tools
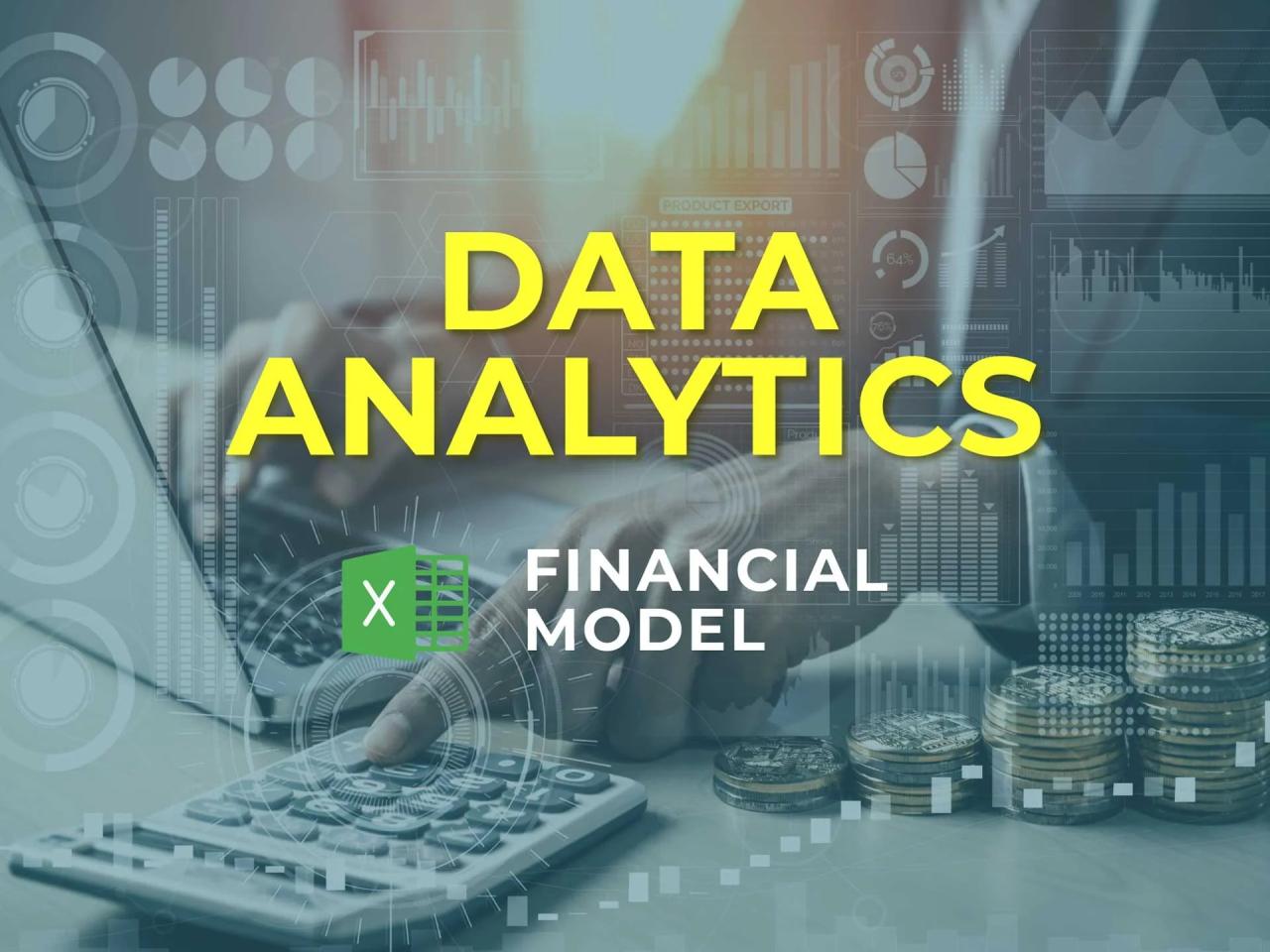
The world of financial data analytics is hurtling towards a future where algorithms dream in spreadsheets and robots negotiate billion-dollar deals. It’s a thrilling, slightly terrifying prospect, but one that’s shaping the industry at an unprecedented pace. Prepare for a wild ride as we explore the emerging trends poised to revolutionize how we crunch numbers and make financial decisions.
The convergence of powerful computing, increasingly sophisticated algorithms, and the sheer volume of available data is creating a perfect storm of innovation. This isn’t just about faster processing; it’s about unlocking entirely new insights and capabilities that were previously unimaginable. The implications for the financial industry are profound, promising both immense opportunities and significant challenges.
Artificial Intelligence and Machine Learning in Financial Data Analytics
AI and machine learning are no longer futuristic fantasies; they are rapidly becoming integral components of financial data analytics tools. These technologies are capable of identifying complex patterns, predicting future trends, and automating tasks with a level of accuracy and speed that surpasses human capabilities. For example, AI-powered fraud detection systems are already saving financial institutions millions by flagging suspicious transactions in real-time, while machine learning algorithms are being used to personalize investment strategies and optimize portfolio management. The integration of these technologies is leading to more accurate risk assessments, more efficient trading strategies, and a more personalized customer experience.
Enhanced Data Visualization and Reporting Capabilities
The ability to effectively communicate complex financial data is paramount. Future financial data analytics tools will prioritize intuitive and visually engaging dashboards and reports. Imagine interactive 3D models illustrating portfolio performance, or heatmaps that instantly highlight areas of risk. These advancements will move beyond simple charts and graphs, allowing users to explore data in more dynamic and insightful ways. For instance, a tool might use augmented reality to overlay key financial metrics onto a real-world map, showing the geographic distribution of investments or the impact of economic events on different regions. This enhanced visualization will lead to better decision-making and improved communication across teams.
Increased Automation and Streamlining of Processes
Financial data analytics tools are rapidly automating tasks that previously required significant manual effort. This includes data cleaning, preprocessing, and even the generation of reports. This automation frees up analysts to focus on higher-level tasks such as strategic planning and interpretation of results. For example, robotic process automation (RPA) can automate repetitive tasks like reconciling accounts or processing invoices, while machine learning can automate the identification of outliers and anomalies in datasets. The result is a significant increase in efficiency and a reduction in human error.
Advanced Predictive Modeling and Forecasting
Predictive modeling is becoming increasingly sophisticated, leveraging AI and machine learning to forecast future financial performance with greater accuracy. These models can incorporate a wider range of data sources, including social media sentiment and news articles, to provide a more holistic view of the market. For example, a bank might use predictive modeling to forecast loan defaults, allowing them to proactively manage risk and mitigate potential losses. Similarly, investment firms are using these models to predict market movements and optimize trading strategies. The enhanced predictive capabilities of future tools will provide a crucial advantage in a rapidly changing financial landscape.
Potential Future Features of Financial Data Analytics Tools
The future of financial data analytics tools is brimming with possibilities. The following features are likely to become increasingly common:
- Integrated AI-powered assistants: Tools that offer personalized guidance and insights, acting as virtual financial analysts.
- Real-time data streaming and analysis: The ability to process and analyze massive datasets in real-time, allowing for immediate responses to market changes.
- Advanced natural language processing (NLP): Tools that can understand and interpret financial news and reports, extracting key insights and automatically updating models.
- Blockchain integration: Secure and transparent data management using blockchain technology to enhance data integrity and traceability.
- Enhanced cybersecurity and compliance features: Robust security measures to protect sensitive financial data and ensure compliance with regulations.
Conclusion
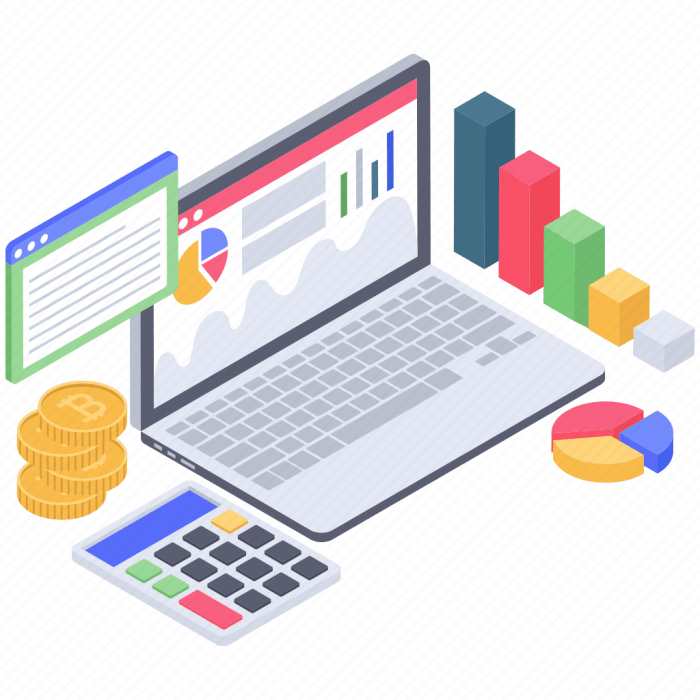
From crunching numbers to predicting the future, financial data analytics tools are revolutionizing the financial world. This review has highlighted the crucial role these tools play in modern finance, emphasizing the importance of data security, compliance, and strategic ROI. By understanding the capabilities and limitations of different tools, financial professionals can make informed decisions, optimize operations, and gain a significant competitive edge. So, buckle up and prepare to harness the power of data!
FAQ Guide
What are the ethical considerations of using financial data analytics tools?
Ethical considerations include data privacy, bias in algorithms, and responsible use of predictive models to avoid discriminatory outcomes. Transparency and accountability are paramount.
How do I choose the right financial data analytics tool for my needs?
Consider your budget, the types of data you need to analyze, the level of technical expertise within your team, and the specific functionalities required (e.g., forecasting, risk management). A thorough needs assessment is crucial.
What is the future of AI in financial data analytics?
AI and machine learning are poised to revolutionize financial data analytics by automating tasks, improving accuracy of predictions, and uncovering previously hidden insights. Expect more sophisticated tools with enhanced predictive capabilities.