Financial Forecasting Tools: Prepare yourself for a delightful romp through the surprisingly exciting world of predicting your financial future! We’ll delve into the fascinating (and sometimes slightly terrifying) art of peering into the fiscal crystal ball, exploring the various tools and techniques used to make sense of those pesky numbers. Get ready to ditch the guesswork and embrace the power of informed financial decisions. This isn’t your grandpappy’s budgeting; this is high-tech financial forecasting!
This exploration will cover various types of forecasting tools – from humble spreadsheets to sophisticated software platforms – and the diverse forecasting methods they employ. We’ll uncover the secrets of data preparation, the art of interpreting results (and knowing when to ignore the scary ones!), and the crucial role of integration with other business systems. Think of it as a crash course in financial fortune-telling, but with significantly less reliance on tea leaves.
Types of Financial Forecasting Tools
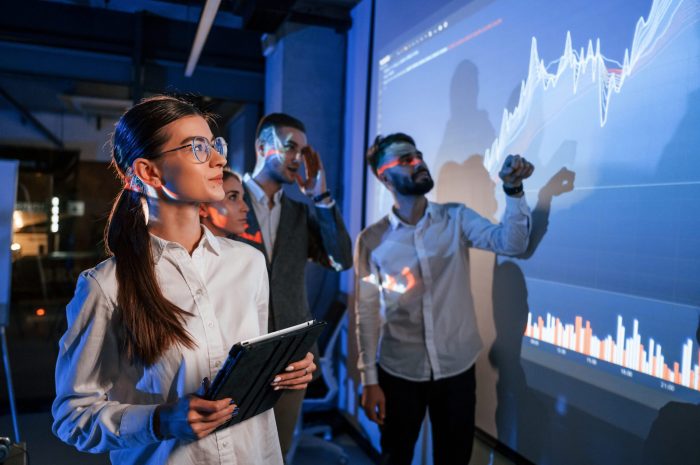
Choosing the right financial forecasting tool can be a bit like choosing a financial advisor – you want someone (or something) that understands your needs and won’t leave you bankrupt. Fortunately, unlike some financial advisors, these tools are relatively inexpensive and won’t try to sell you a timeshare in the Bahamas. Let’s explore the landscape of available options.
Spreadsheet Software
Spreadsheet software, the workhorses of the financial world, offer a surprisingly robust foundation for financial forecasting. Their accessibility and versatility make them a popular choice for individuals and small businesses. However, their limitations become apparent as complexity increases.
Tool Name | Category | Key Features | Typical User |
---|---|---|---|
Microsoft Excel | Spreadsheet Software | Formulas, charts, macros, extensive add-ins. | Individuals, small businesses, financial analysts. |
Google Sheets | Spreadsheet Software | Collaboration features, real-time updates, integration with other Google services. | Teams, individuals requiring collaboration, budget-conscious users. |
LibreOffice Calc | Spreadsheet Software | Open-source, compatible with Microsoft Excel, basic financial functions. | Users seeking free and open-source alternatives. |
Spreadsheet software strengths include ease of use, accessibility, and cost-effectiveness. Weaknesses include limitations in handling large datasets, lack of advanced forecasting models, and potential for human error.
Dedicated Financial Planning Software
These software packages offer more sophisticated features tailored specifically for financial forecasting. They typically include pre-built templates, advanced modeling capabilities, and reporting tools. However, they often come with a higher price tag and a steeper learning curve.
Tool Name | Category | Key Features | Typical User |
---|---|---|---|
Adaptive Insights | Dedicated Financial Planning Software | Cloud-based, collaborative planning, scenario modeling, sophisticated reporting. | Mid-sized to large businesses, financial planning teams. |
Anaplan | Dedicated Financial Planning Software | Highly scalable, in-memory calculation engine, robust API integrations. | Large enterprises, complex financial modeling needs. |
Vena | Dedicated Financial Planning Software | Excel-based interface, cloud connectivity, workflow automation. | Companies seeking a balance between Excel familiarity and advanced features. |
Dedicated financial planning software strengths include advanced features, better scalability, and improved accuracy. Weaknesses include higher costs, steeper learning curves, and potentially less flexibility than spreadsheets.
Specialized Forecasting Platforms
These platforms are designed for highly specific forecasting tasks, often incorporating advanced statistical models and machine learning. They are generally the most expensive and require specialized expertise.
Tool Name | Category | Key Features | Typical User |
---|---|---|---|
Forecast Pro | Specialized Forecasting Platforms | Wide range of statistical forecasting models, robust data analysis capabilities. | Demand planners, supply chain managers, statisticians. |
Demand Solutions | Specialized Forecasting Platforms | Advanced algorithms, collaboration tools, integration with ERP systems. | Large enterprises with complex supply chains. |
DataRobot | Specialized Forecasting Platforms | AI-driven forecasting, automated model building, explainable AI features. | Data scientists, businesses needing highly accurate, automated forecasts. |
Specialized forecasting platforms offer the most sophisticated capabilities but often come with a steep learning curve and high cost, making them suitable only for businesses with very specific needs and the resources to support them.
Forecasting Methods Employed
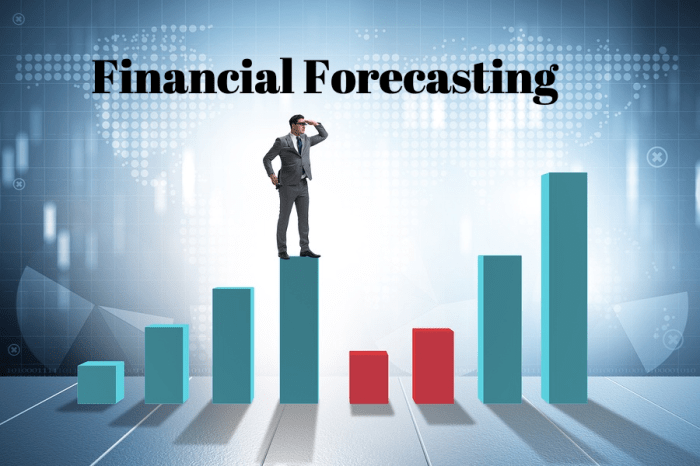
Financial forecasting, while sounding like a high-stakes poker game with your company’s future on the line, is actually a fascinating blend of art and science. We’re not predicting the next lottery winner here, but rather using robust methods to make informed estimations about your business’s financial health. These methods provide a roadmap, allowing you to navigate the often-murky waters of future financial performance. Let’s dive into the exciting world of forecasting methodologies.
Several methods exist, each with its own strengths and weaknesses, making the choice dependent on the specific data and desired level of accuracy. Choosing the right tool is crucial – picking the wrong one is like trying to bake a cake with a hammer; it’s just not going to work.
Time Series Analysis
Time series analysis examines data points collected over time to identify patterns and trends. Think of it as a financial detective story, where the data points are clues leading to predictions about the future. This method is particularly useful for forecasting sales, revenue, and other metrics that exhibit consistent patterns over time. The mathematical backbone often involves techniques like moving averages (simple, weighted, exponential), ARIMA models (Autoregressive Integrated Moving Average), and exponential smoothing. These methods aim to smooth out the noise in the data and reveal the underlying trends. For example, a company analyzing monthly sales data over the past five years could use exponential smoothing to predict sales for the next quarter.
Regression Analysis
Regression analysis explores the relationship between a dependent variable (what you want to predict, like profit) and one or more independent variables (factors influencing profit, like advertising spend or market size). It’s like building a mathematical model that helps you understand how different factors contribute to the outcome. The most common type is linear regression, where the relationship is assumed to be linear. However, more complex models exist to handle non-linear relationships. The underlying principle involves minimizing the sum of squared errors between the predicted and actual values. A retail company could use regression analysis to predict sales based on factors like advertising expenditure, seasonality, and competitor pricing.
Moving Averages, Financial Forecasting Tools
Moving averages are a straightforward method that smooths out short-term fluctuations in data to reveal underlying trends. Imagine it like averaging your grades over a semester rather than focusing on each individual quiz score. Simple moving averages average the data over a specific period, weighted moving averages give more weight to recent data, and exponential moving averages give exponentially decreasing weights to older data. The mathematical foundation is simply calculating the average of a specified number of data points. A financial analyst could use a 12-month moving average of stock prices to identify long-term trends and make investment decisions.
ARIMA Models
ARIMA (Autoregressive Integrated Moving Average) models are more sophisticated time series models that account for autocorrelations within the data. They’re particularly useful when dealing with non-stationary time series, meaning the statistical properties of the data change over time. These models involve intricate mathematical formulations, combining autoregressive (AR), integrated (I), and moving average (MA) components. The parameters of the model are estimated using statistical methods, and the model is then used to forecast future values. An economist could use an ARIMA model to predict inflation based on historical inflation rates and other economic indicators.
Qualitative Forecasting Methods
While the previous methods are quantitative, relying heavily on numerical data, qualitative methods utilize expert judgment and intuition. This approach involves surveys, expert panels, or the Delphi method, where experts provide their forecasts anonymously. While lacking the mathematical rigor of quantitative methods, these approaches are invaluable when historical data is limited or unreliable. A new startup, lacking historical sales data, might rely on expert opinions from industry veterans to estimate its initial market share.
Applicability of Forecasting Methods
Method | Data Type | Strengths | Weaknesses |
---|---|---|---|
Time Series Analysis | Sales, Revenue, Cash Flow | Identifies trends and patterns, relatively simple to implement for basic models | Assumes past trends will continue, sensitive to outliers, may not capture sudden shifts |
Regression Analysis | Sales, Expenses, Profit | Considers multiple factors, provides insights into relationships between variables | Requires significant data, assumptions about linearity can be violated, prone to overfitting |
Moving Averages | Sales, Stock Prices, Cash Flow | Simple to understand and implement, smooths out short-term fluctuations | Lags behind actual data, may not capture rapid changes, choice of window size is crucial |
ARIMA Models | Sales, Economic Indicators, Inflation | Handles non-stationary data, captures complex relationships | Requires statistical expertise, can be computationally intensive, model selection can be challenging |
Qualitative Methods | Market Share, New Product Sales | Useful when historical data is scarce, incorporates expert knowledge | Subjective, prone to bias, less precise than quantitative methods |
Data Input and Preparation
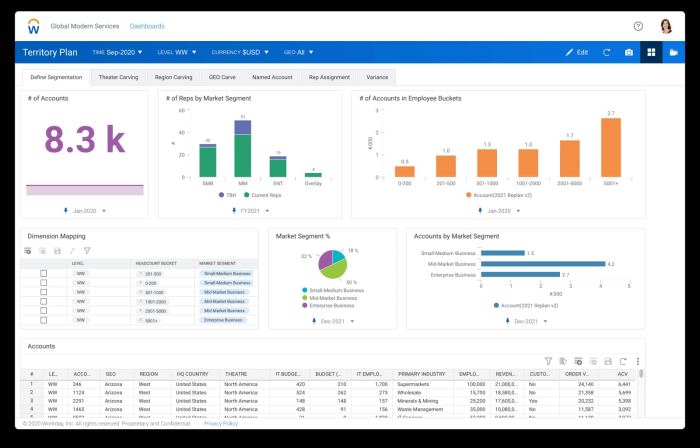
Preparing your financial data for forecasting is like prepping ingredients for a gourmet meal – if you don’t do it right, the final product will be a disaster (or at least, a bit bland). Accurate forecasting hinges on clean, consistent, and validated data. Let’s dive into the delicious world of data preparation.
The process involves three key steps: cleaning, transforming, and validating your data. Data cleaning involves identifying and correcting or removing errors. Data transformation involves converting data into a format suitable for your forecasting tool. Finally, data validation confirms the accuracy and reliability of your prepared data. Think of it as a three-course meal for your forecasting model.
Data Cleaning
Data cleaning is where the real fun begins (or, let’s be honest, the tedious but crucial part). This involves identifying and dealing with inconsistencies, inaccuracies, and missing values. Imagine trying to bake a cake with a missing ingredient – it’s not going to turn out well! Common errors include typos, inconsistencies in formatting (e.g., dates in different formats), and outliers that significantly skew your results. For example, a revenue figure of $1,000,000,000 instead of $1,000,000 would drastically alter your forecast.
Data Transformation
Once your data is clean, it’s time to transform it. This might involve converting data types (e.g., text to numbers), creating new variables (e.g., calculating ratios or growth rates), or standardizing units (e.g., converting currencies). Think of this as seasoning your ingredients to bring out the best flavors. A common transformation is converting nominal data into a format suitable for regression analysis. For example, you might need to create dummy variables for categorical data like product type or region.
Data Validation
After cleaning and transforming your data, validation is essential. This involves checking the accuracy and consistency of your data to ensure it aligns with your expectations and business realities. You might compare your data to historical records or industry benchmarks. For example, if your forecast for sales growth is 50% higher than the average growth rate of your competitors, you might want to re-examine your data and assumptions. A thorough validation process ensures that your forecast is reliable and believable, not just a figment of your (or your software’s) imagination.
Challenges in Data Preparation and Strategies for Overcoming Them
Preparing financial data isn’t always a walk in the park. You might encounter missing data, inconsistencies across different data sources, or data that’s simply not reliable. For example, during a period of significant economic uncertainty, you might find that your historical data is no longer a good predictor of future performance. Strategies to overcome these challenges include using imputation techniques for missing data, standardizing data formats, and employing data quality checks. Sometimes, it might even involve going back to the source and getting more accurate information – a bit of detective work might be necessary.
Examples of Common Data Errors and How to Identify and Correct Them
Let’s look at some common culprits and how to catch them. Inconsistent date formats (e.g., MM/DD/YYYY vs. DD/MM/YYYY) can lead to errors in time series analysis. Outliers (extremely high or low values) can skew your results, and these need careful examination to determine whether they represent genuine events or data entry errors. Missing values require imputation (filling in missing data using statistical methods) or removal, depending on the extent and nature of the missing data. Finally, duplicate data can inflate your results and lead to inaccurate forecasts. Regular data checks and validation steps are crucial to identify and correct these errors before they ruin your forecasting efforts.
Interpretation of Forecast Results
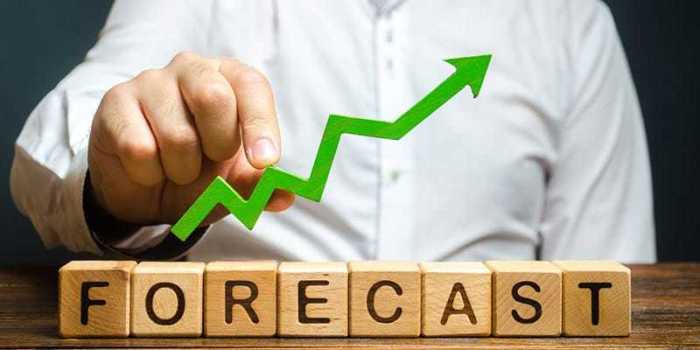
So, you’ve fed your financial forecasting tool mountains of data, wrestled with algorithms, and finally, *voilà*! A forecast emerges, shimmering like a freshly-minted gold coin (though hopefully less prone to inflation). But before you start planning your yacht purchase, let’s delve into the crucial art of interpreting these numerical pronouncements. Understanding your forecast isn’t just about reading the numbers; it’s about deciphering the story they tell.
Interpreting forecast results involves more than simply glancing at the numbers; it requires a keen eye for detail and a healthy dose of skepticism. We need to move beyond simply accepting the output as gospel truth and instead, critically assess its implications. This involves identifying trends and patterns, assessing accuracy, and, crucially, acknowledging the ever-present shadow of uncertainty.
Identifying Key Trends and Patterns
Forecasts are not static snapshots; they reveal dynamic trends and patterns over time. For example, a forecast showing consistently increasing revenue over several quarters suggests a healthy growth trajectory. Conversely, a downward trend in profit margins might indicate the need for cost-cutting measures or a reassessment of pricing strategies. By carefully examining the forecast’s evolution, we can identify turning points, cyclical fluctuations, and long-term growth or decline. Imagine a line graph: a steadily rising line speaks volumes about consistent growth, while a jagged line might hint at market volatility and the need for more robust risk management. Identifying these patterns allows for proactive strategic planning and informed decision-making.
Assessing Forecast Accuracy and Reliability
While no forecast is perfect (a crystal ball would be far more convenient, wouldn’t it?), we can assess their reliability using various metrics. One common approach is to compare the forecast to actual results from previous periods. Calculating the Mean Absolute Deviation (MAD) or the Mean Absolute Percentage Error (MAPE) can provide quantitative measures of forecast accuracy. A low MAD or MAPE indicates a more accurate forecast. For example, if our forecast predicted $10 million in revenue and the actual revenue was $9.8 million, the error is relatively small, suggesting a reliable forecast. However, a consistently high error rate should prompt a review of the forecasting methodology and data inputs.
Considering Uncertainty and Risk
Let’s face it: the future is inherently uncertain. Even the most sophisticated forecasting tools cannot predict unforeseen events like global pandemics or sudden shifts in consumer behavior. Therefore, interpreting forecast results requires acknowledging the inherent uncertainty and incorporating risk assessment into the decision-making process. This might involve conducting sensitivity analyses to assess how changes in key variables (e.g., interest rates, exchange rates) impact the forecast. Scenario planning, where different possible future scenarios are modeled, can also provide valuable insights into potential risks and opportunities. For instance, a forecast might show optimistic and pessimistic scenarios, allowing businesses to prepare for both positive and negative outcomes. Remember, a robust forecast acknowledges uncertainty; it doesn’t try to eliminate it.
Integration with Other Systems
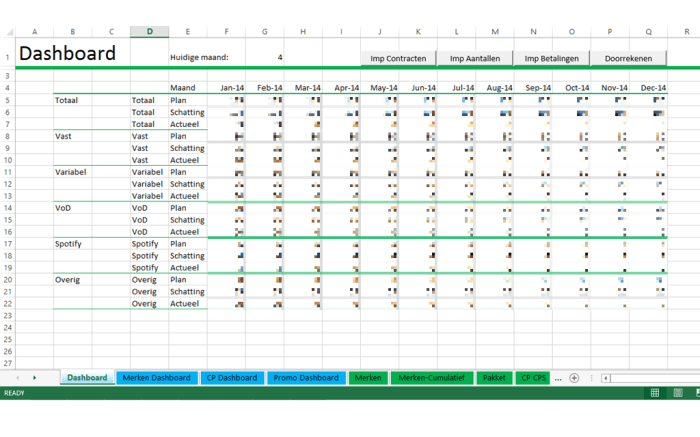
Financial forecasting tools, while powerful on their own, truly shine when integrated with other business systems. Think of it as a well-oiled machine – each part contributes, but the seamless interaction creates a masterpiece of efficiency and insight. Integrating these tools allows for a more holistic view of the business, leading to better, more informed decisions. Ignoring this crucial step is like trying to bake a cake with only one ingredient – flour. You might get something, but it won’t be a cake.
Integrating financial forecasting tools with other business systems, such as Enterprise Resource Planning (ERP) systems and Customer Relationship Management (CRM) systems, offers significant advantages, but also presents unique challenges. The key is to carefully consider the benefits and potential pitfalls before embarking on this digital dance.
Methods of Integration
Several methods facilitate the integration of financial forecasting tools with other business systems. These methods range from simple data exports and imports to more sophisticated real-time data exchange using Application Programming Interfaces (APIs). Direct database connections are also common, offering a high degree of efficiency and speed. The choice of method depends largely on the technical capabilities of the systems involved and the desired level of integration. For instance, a smaller business might opt for simpler data exports, while a large corporation would likely leverage APIs for real-time data synchronization.
Benefits of Integration
The benefits of integrating financial forecasting tools with other business systems are numerous and impactful. Imagine having access to real-time sales data from your CRM system directly feeding into your forecasting model. This allows for more accurate and responsive forecasts, reducing the risk of costly miscalculations. Similarly, integration with an ERP system can provide up-to-the-minute information on inventory levels, production costs, and other critical operational data, leading to more comprehensive and reliable forecasts. The resulting improvements in forecasting accuracy and timeliness translate directly into better decision-making and improved business performance. For example, a company using integrated systems might predict a potential inventory shortage and proactively adjust production schedules, avoiding stockouts and lost sales.
Challenges of Integration
While the benefits are clear, integrating these systems is not without its challenges. Data compatibility issues are a common hurdle. Different systems often use different data formats and structures, requiring careful data transformation and mapping to ensure seamless data flow. Maintaining data consistency and accuracy across multiple systems is also crucial. Inconsistent data can lead to inaccurate forecasts and flawed decision-making. Furthermore, the initial investment in integration can be substantial, requiring specialized expertise and potentially significant IT resources. Consider the potential for security vulnerabilities – robust security measures are essential to protect sensitive financial data.
Data Flow between Forecasting Tool and ERP System
A visual representation of the data flow between a financial forecasting tool and a hypothetical ERP system is beneficial for understanding the integration process.
Data Flow Diagram:
Imagine a flowchart. The ERP system (the “source”) contains various data points: sales orders, production costs, inventory levels, etc. Arrows indicate the flow of this data. These data points are extracted from the ERP system, possibly through an API or direct database connection. The data then undergoes a transformation process, cleaning and formatting it for compatibility with the financial forecasting tool. This cleaned data is then input into the forecasting tool. The forecasting tool processes this data, applying chosen forecasting methods (e.g., ARIMA, exponential smoothing) to generate financial forecasts. Finally, the forecast results are then sent back to the ERP system, or perhaps to a data visualization dashboard, for review and analysis. This continuous loop of data flow allows for dynamic adjustments and iterative improvements in the forecasting process. For example, if sales figures unexpectedly increase, the ERP system feeds this new data into the forecasting tool, which adjusts the forecast accordingly.
Visualizations and Reporting
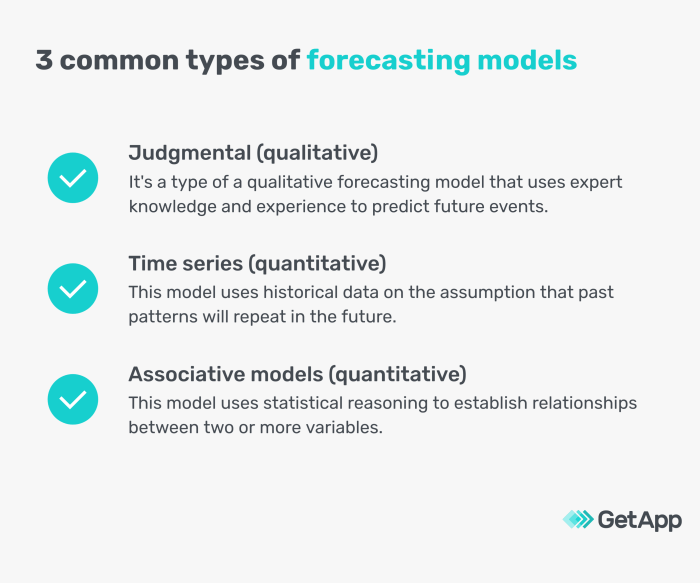
Financial forecasting tools aren’t just about crunching numbers; they’re about making those numbers sing (or at least, hum a catchy tune). Effective visualization transforms complex financial data into easily digestible insights, turning potential spreadsheet nightmares into compelling narratives. Think of it as translating accountant-speak into plain English (with maybe a few witty charts thrown in for good measure).
Visual representations are the key to unlocking the true potential of your forecast. They allow stakeholders to quickly grasp the overall picture, identify trends, and make informed decisions without needing a PhD in finance. A well-designed visualization can be worth a thousand (well, maybe a hundred) words, especially when those words are about projected revenue or potential losses.
Chart Types for Financial Forecasts
Financial forecasting tools offer a diverse range of chart types, each best suited for different types of data and insights. Choosing the right chart is crucial for effective communication. A poorly chosen chart can obscure the story your data is trying to tell, while a well-chosen one can illuminate it beautifully.
- Line Charts: Ideal for showing trends over time, perfect for visualizing revenue projections, expenses, or cash flow over a period of months or years. Imagine a gracefully curving line showing your projected profits soaring skyward – a visual masterpiece!
- Bar Charts: Excellent for comparing different categories, such as sales across various regions or product lines. Think of colorful bars representing regional sales, with the tallest bar proudly proclaiming the most successful region.
- Pie Charts: Useful for displaying the proportion of different components within a whole, such as the allocation of a budget across various departments. A perfectly segmented pie chart shows a clear and concise picture of your budget breakdown.
- Scatter Plots: Show the relationship between two variables, such as sales and marketing spend. This allows for identifying correlations and potential causal relationships. A carefully plotted scatter graph can reveal hidden connections between your investments and returns.
Example of an Effective Visualization: Sales Forecast
Imagine a line chart showing projected sales for the next three years. The x-axis represents time (in months or quarters), and the y-axis represents sales revenue (in dollars). The chart displays three lines: one for the optimistic scenario, one for the pessimistic scenario, and one for the most likely scenario. Each line’s color coding makes it instantly clear which forecast it represents. The chart also includes data labels showing the projected revenue for key points in time. This clear, concise visual instantly communicates the range of potential outcomes and allows stakeholders to easily understand the risk involved.
Sample Forecast Report
This report summarizes the financial forecast for the next fiscal year. It uses data from the company’s historical performance and market research, employing a time-series analysis forecasting method.
- Key Finding 1: Projected revenue growth of 15% year-over-year, driven by strong sales in the new product line.
- Key Finding 2: Operating expenses are projected to increase by 10%, primarily due to increased marketing investments.
- Key Finding 3: Net income is expected to improve by 20%, resulting in a healthy profit margin.
Recommendations: Continue investing in the new product line, while carefully monitoring operating expenses to ensure profitability. Explore opportunities to further reduce costs without sacrificing product quality or market share. Consider implementing a contingency plan to mitigate potential risks, such as a sudden economic downturn or unexpected supply chain disruptions.
Cost and Accessibility
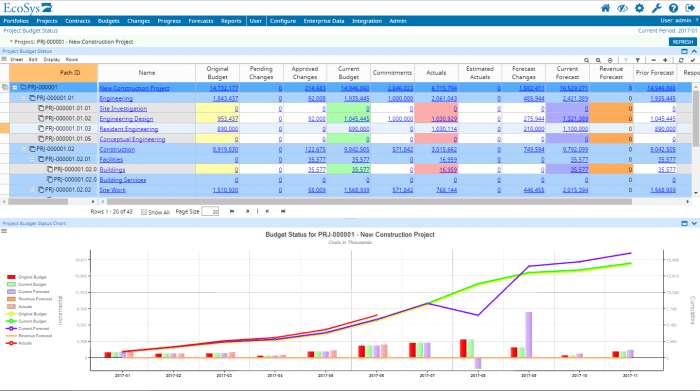
Choosing the right financial forecasting tool is a bit like choosing a financial advisor – you want someone (or something) reliable, capable, and, let’s be honest, not going to break the bank. The cost and accessibility of these tools vary wildly, so careful consideration is crucial to avoid a budgetary black hole or a user experience so frustrating it makes you want to stick to spreadsheets.
The pricing models for financial forecasting tools are as diverse as the tools themselves. Some operate on a subscription basis, offering various tiers with different features and levels of support. Think of it like Netflix for your financial future – you pay a monthly or annual fee for access. Others are one-time purchases, a bit like buying a high-quality calculator – a significant upfront cost, but no recurring expenses. Then there are hybrid models, combining aspects of both, offering a basic version for free and premium features via subscription.
Pricing Models Comparison
Subscription-based models offer predictable monthly or annual costs, but you’re locked into a contract. One-time purchases represent a larger upfront investment but avoid ongoing fees. Hybrid models provide flexibility, but managing different access levels can become complex. Consider the size of your organization and the expected frequency of use when choosing between these models. A small business might find a one-time purchase more cost-effective, while a large corporation might prefer the scalability of a subscription. For example, a small bakery might find a simple, one-time purchase tool sufficient, while a multinational corporation would likely benefit from a more comprehensive, subscription-based platform offering advanced analytics and scalability.
Factors Affecting Cost-Effectiveness
Evaluating the cost-effectiveness of a financial forecasting tool requires looking beyond the price tag. Consider the tool’s features, accuracy, ease of use, and integration capabilities. A cheaper tool with limited functionality might ultimately cost more in time and resources if it’s inefficient or inaccurate. Think of it as buying a cheap, unreliable car – the initial saving might seem appealing, but the constant repairs and downtime will quickly negate any advantage. Factors such as the level of technical support offered, the availability of training resources, and the potential for future upgrades should also be factored into the overall cost-benefit analysis. For example, a tool with excellent customer support might justify a higher price if it significantly reduces the time spent troubleshooting problems.
Accessibility and User-Friendliness
Accessibility encompasses both the technical requirements and the user experience. Some tools require specialized software or hardware, potentially adding to the overall cost and complexity. Others are cloud-based, accessible from any device with an internet connection, making them far more convenient and accessible to a wider range of users. User-friendliness is equally important. A tool with a clunky interface or a steep learning curve can negate the benefits of advanced features. Ideally, a financial forecasting tool should be intuitive, easy to navigate, and require minimal technical expertise to operate effectively. Imagine trying to use a complex piece of software to forecast your company’s profits – if it takes weeks to learn, the time savings are negated. A user-friendly interface, on the other hand, can significantly streamline the process, making forecasting efficient and accessible to a broader team.
Closure: Financial Forecasting Tools
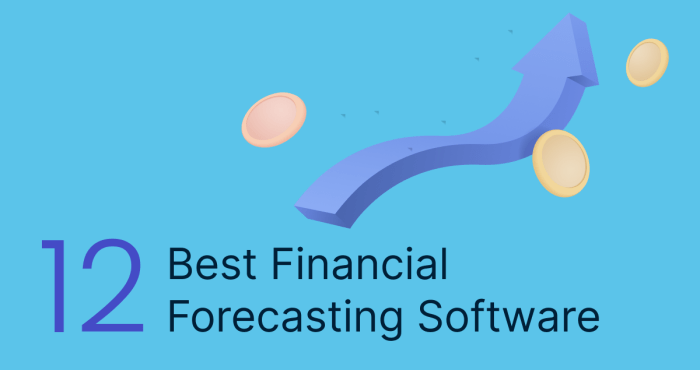
So, there you have it: a whirlwind tour of the world of financial forecasting tools. While the process might seem daunting at first, remember that even the most complex forecasts begin with a single number (or perhaps a slightly messy spreadsheet). By understanding the various tools, methods, and interpretations, you can transform financial forecasting from a mysterious process into a powerful tool for strategic planning and growth. Now go forth and conquer those spreadsheets – or at least, make them slightly less terrifying.
Quick FAQs
What if my forecast is wildly inaccurate?
Don’t panic! Inaccurate forecasts are a learning opportunity. Analyze what went wrong, adjust your methods, and refine your data. It’s a marathon, not a sprint (unless your finances are *really* on fire).
How much does financial forecasting software cost?
Prices vary wildly, from free spreadsheet solutions to expensive enterprise-level platforms. Consider your needs and budget carefully – a Ferrari might be nice, but a reliable bicycle might be more practical.
Is it possible to forecast with limited data?
Yes, but the accuracy will be lower. Focus on gathering as much relevant data as possible, and use forecasting methods suitable for limited datasets. It’s like baking a cake with half the ingredients – it might still be edible, but don’t expect a masterpiece.
Can I use these tools for personal finance?
Absolutely! Many tools are scalable and adaptable for both personal and business use. Start small, experiment, and find what works best for you. You deserve a financially stable future, even if it involves a few spreadsheets.