Mastering financial modeling is crucial for sound business decisions. This guide delves into the art of creating robust, reliable, and insightful financial models. We’ll explore the key components of a best-in-class model, from defining “best” practices to advanced techniques like Monte Carlo simulations and DCF modeling. We’ll also cover essential software, ethical considerations, and effective presentation strategies, ensuring you can build and communicate your financial models with confidence.
From defining clear assumptions and mitigating potential errors to effectively communicating complex financial information, this comprehensive guide equips you with the knowledge and skills to build high-quality financial models that drive informed decision-making. We’ll cover everything from basic principles to advanced techniques, ensuring a solid understanding for both beginners and experienced professionals.
Defining “Best” in Financial Modeling
Defining “best” in financial modeling isn’t about adhering to a single, rigid standard. Instead, it’s about creating a model that accurately reflects the underlying business, is reliable for its intended purpose, and is easily understood and maintained. A “best” model prioritizes clarity, accuracy, and robustness, tailored to the specific needs of the user and the complexity of the situation.
The criteria for determining “best” practices in financial modeling are multifaceted. A highly effective model will be both accurate and transparent. Accuracy stems from the use of reliable data and appropriate assumptions, while transparency ensures the model’s logic and calculations are readily understandable. This allows for easier auditing, validation, and future adjustments. Reliability is crucial; the model should consistently produce consistent and plausible results under various scenarios. Furthermore, a well-structured model is easier to update and maintain, minimizing errors and saving time in the long run.
Factors Contributing to Model Effectiveness and Reliability
Several key factors contribute to the effectiveness and reliability of a financial model. Data quality is paramount; inaccurate or incomplete data will inevitably lead to flawed results. The model’s structure should be logically sound and well-organized, making it easier to understand and debug. Appropriate assumptions are critical; these should be clearly stated, justified, and regularly reviewed. Robustness refers to the model’s ability to handle changes in inputs without producing unrealistic or nonsensical outputs. Finally, comprehensive documentation is essential for ensuring the model’s longevity and usability. A well-documented model makes it easy for others (and even the original creator, months or years later) to understand how it works and make necessary adjustments.
Comparison of Different Modeling Approaches
Different approaches to financial modeling exist, each with its own strengths and weaknesses. For example, a discounted cash flow (DCF) model is commonly used for valuation, but relies heavily on future projections which can be inherently uncertain. A comparable company analysis, on the other hand, uses market multiples to estimate value, but can be sensitive to the selection of comparable firms. Leveraged buyout (LBO) models are utilized to assess the financial feasibility of acquisitions, focusing on debt financing and returns to equity investors. Each approach has its place; the “best” approach depends entirely on the specific context and the information available. A DCF model might be appropriate for a mature, stable company with predictable cash flows, while a comparable company analysis might be more suitable for a young, rapidly growing firm with limited historical data.
Examples of Best Practices in Financial Modeling
Best practices often include using clear and consistent naming conventions for cells and sheets. This enhances readability and reduces the likelihood of errors. Another key practice is employing robust error checking and validation. This could involve using data validation rules in spreadsheets to ensure inputs fall within acceptable ranges. Furthermore, separating inputs, calculations, and outputs into distinct sections within the model enhances clarity and maintainability. This modular approach simplifies the process of reviewing and updating the model. Finally, regularly backing up the model and version controlling changes are crucial for preventing data loss and tracking modifications over time. A version control system allows for easy rollback to previous versions if errors are detected or adjustments are needed. For instance, imagine a model used for a major investment decision; the ability to track and revert to prior versions could save millions of dollars if an error is found.
Core Components of a Best-in-Class Model
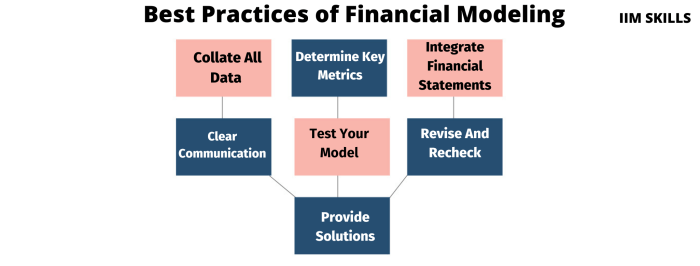
A best-in-class financial model is more than just a collection of numbers; it’s a robust, transparent, and easily understandable tool that provides accurate insights for informed decision-making. Its core strength lies in its design and the meticulous attention to detail in its construction. This section will delve into the key components that contribute to a model’s excellence.
Essential Components of a Robust Financial Model
The following table Artikels the critical components necessary for building a high-quality financial model. Each component plays a vital role in ensuring accuracy, reliability, and usability.
Component | Description | Importance | Example |
---|---|---|---|
Assumptions | Clearly defined and documented inputs that drive the model’s calculations. | Forms the foundation of the model; inaccuracies here propagate throughout. | Growth rates, discount rates, market share projections. |
Data Input & Validation | Structured and verifiable data sources; robust checks for accuracy and consistency. | Ensures the model’s reliability and reduces the risk of errors. | Using validated financial statements, referencing industry benchmarks. |
Formulas & Calculations | Accurate and transparent formulas that reflect the underlying financial logic. | Accuracy of the model hinges on the correctness of these calculations. | Using standard accounting principles (GAAP or IFRS) for calculations. |
Structure & Formatting | Clear, logical structure with well-formatted outputs and easy navigation. | Improves model usability and makes it easier to audit and understand. | Using color-coding, clear labels, and consistent formatting. |
Documentation & Audit Trail | Comprehensive documentation explaining the model’s logic, assumptions, and data sources. | Enables others to understand and verify the model’s results. | Detailed notes explaining each section and formula, version control. |
Sensitivity Analysis & Scenario Planning | Exploring the impact of changes in key assumptions on the model’s outputs. | Provides insights into the model’s robustness and potential risks. | Varying discount rates to see their impact on net present value. |
The Importance of Clear Assumptions and Their Impact on Model Accuracy
Assumptions are the bedrock of any financial model. They represent the predictions and estimations about future events that drive the model’s calculations. Clearly defining and documenting these assumptions is crucial. Ambiguous or poorly defined assumptions can lead to significant inaccuracies and unreliable results. For example, an inaccurate assumption about future revenue growth can drastically alter projected profitability and valuation. Therefore, a thorough sensitivity analysis, testing the model’s response to variations in key assumptions, is vital to understanding the model’s robustness and limitations.
Best Practices for Data Input and Validation Within a Financial Model
Data validation is a critical aspect of building a reliable model. Best practices include using multiple data sources to cross-check information, implementing data validation rules within spreadsheets (e.g., data type checks, range checks), and documenting the data sources and their reliability. Automated checks can also be implemented to flag inconsistencies or potential errors. For instance, a model predicting future sales might use historical sales data, market research reports, and expert opinions, cross-referencing these sources to minimize errors.
Potential Sources of Error in Financial Modeling and Methods for Mitigation
Several factors can introduce errors into financial models. These include incorrect formulas, inaccurate data input, flawed assumptions, and inadequate testing. Mitigation strategies include rigorous testing of formulas, thorough data validation, sensitivity analysis to assess the impact of assumption changes, and peer review to identify potential blind spots. For instance, a circular reference in a formula could lead to incorrect results; rigorous testing can help identify and correct such errors. Similarly, using outdated or unreliable data sources can significantly impact model accuracy; therefore, regular data updates and validation are essential.
Advanced Modeling Techniques
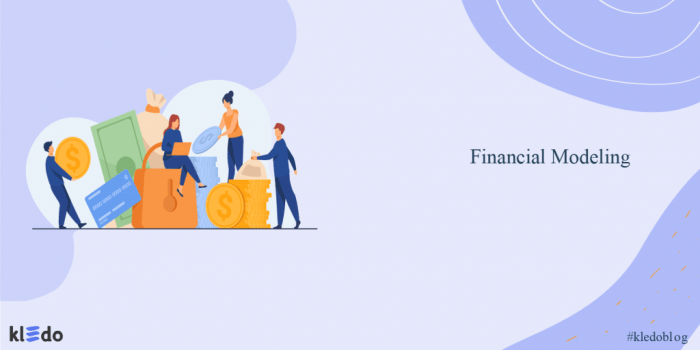
Building robust and insightful financial models often requires incorporating advanced techniques that go beyond basic spreadsheet functionality. These techniques enhance the model’s predictive power, allowing for a more comprehensive understanding of risk and uncertainty inherent in financial forecasting. This section explores several key advanced modeling techniques crucial for creating best-in-class financial models.
Scenario Analysis and Sensitivity Testing
Scenario analysis and sensitivity testing are complementary techniques used to assess the impact of changes in key assumptions on a model’s output. Scenario analysis involves creating multiple “what-if” scenarios by altering several input variables simultaneously. For instance, a company might model different economic scenarios (e.g., recession, moderate growth, strong growth) to see how projected profits vary under each condition. Sensitivity testing, on the other hand, isolates the impact of individual variables. By changing one variable at a time, while holding others constant, we can determine which assumptions have the greatest influence on the final results. For example, a sensitivity analysis on a DCF model might reveal that changes in the discount rate have a far greater impact on valuation than changes in revenue growth. This allows for focused attention on the most critical assumptions, improving the model’s reliability.
Incorporating Monte Carlo Simulations
Monte Carlo simulation is a powerful technique for quantifying risk and uncertainty. Unlike scenario analysis, which considers a limited number of pre-defined scenarios, Monte Carlo simulation generates thousands or even millions of random simulations based on probability distributions assigned to the model’s input variables. Each simulation produces a different outcome, allowing for a statistical distribution of possible results. For example, in a project valuation, instead of assuming a fixed revenue growth rate, a Monte Carlo simulation might use a triangular distribution with minimum, most likely, and maximum values. The simulation would then randomly sample from this distribution for each iteration, generating a range of possible net present values (NPVs) and associated probabilities. The resulting distribution provides a much richer understanding of the project’s risk profile than a single point estimate. This allows for a more informed decision-making process.
Building a Discounted Cash Flow (DCF) Model: A Step-by-Step Guide
A DCF model is a fundamental valuation technique that estimates the present value of future cash flows. Building a robust DCF model requires careful consideration of several steps:
- Project Free Cash Flows (FCF): This involves forecasting the company’s future cash flows, typically for 5-10 years. This projection should be based on realistic assumptions about revenue growth, operating margins, capital expenditures, and working capital requirements. Consider using historical data, industry benchmarks, and management forecasts to inform these projections.
- Determine the Terminal Value: Since it’s impractical to forecast cash flows indefinitely, a terminal value is calculated to represent the value of all cash flows beyond the explicit forecast period. Common methods include the perpetuity growth method or the exit multiple method. The perpetuity growth method assumes a constant growth rate of cash flows beyond the forecast period. The exit multiple method uses a multiple of a terminal year’s cash flow or earnings.
- Select a Discount Rate: The discount rate reflects the risk associated with the investment. The weighted average cost of capital (WACC) is commonly used as the discount rate. This requires determining the company’s cost of equity and cost of debt, as well as the proportion of debt and equity in its capital structure. The formula for WACC is:
WACC = (E/V) * Re + (D/V) * Rd * (1 – Tc)
where E is market value of equity, D is market value of debt, V = E + D, Re is cost of equity, Rd is cost of debt, and Tc is corporate tax rate.
- Discount the Cash Flows: The projected free cash flows and the terminal value are discounted back to their present values using the chosen discount rate. The sum of these present values represents the enterprise value of the company.
- Calculate Equity Value: Subtract net debt (total debt less cash and cash equivalents) from the enterprise value to arrive at the equity value.
Comparison of Valuation Methods
Several valuation methods exist beyond DCF, each with its strengths and weaknesses. These include relative valuation (comparing a company’s valuation multiples to those of its peers), precedent transactions (analyzing the prices paid for similar companies in previous acquisitions), and asset-based valuation (estimating the value of a company’s assets). Relative valuation is quick and easy but susceptible to market sentiment. Precedent transactions are relevant but may lack perfect comparables. Asset-based valuation is suitable for companies with primarily tangible assets but may undervalue intangible assets like brand reputation or intellectual property. The choice of valuation method depends on the specific circumstances of the company being valued and the availability of relevant data. A comprehensive valuation often involves employing multiple methods and comparing the results.
Software and Tools for Financial Modeling
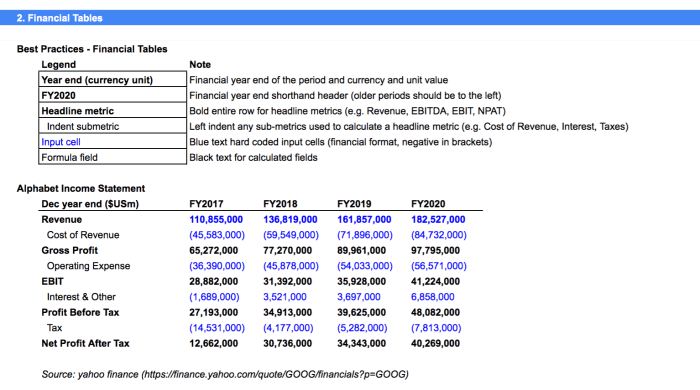
Selecting the right software is crucial for building efficient and accurate financial models. The choice depends on factors such as model complexity, data volume, team collaboration needs, and budget. A range of options exists, from widely available spreadsheet programs to specialized financial modeling platforms. Understanding the strengths and weaknesses of each is essential for making an informed decision.
The software landscape for financial modeling offers a variety of tools catering to different needs and skill levels. Each option presents a unique set of capabilities and limitations that modellers should carefully consider before selecting a platform for their project.
Popular Financial Modeling Software Packages
Several software packages dominate the financial modeling space, each offering distinct features and capabilities. The selection often hinges on the specific requirements of the modeling task and the user’s familiarity with the software.
- Microsoft Excel: The industry standard, offering extensive functionalities, including formulas, macros, charting, and data analysis tools. Its widespread use ensures ease of collaboration and accessibility. However, it can be prone to errors if not used carefully and lacks advanced features found in dedicated platforms.
- Google Sheets: A cloud-based alternative to Excel, offering real-time collaboration and accessibility from any device. It shares many of Excel’s functionalities but may have limitations in handling extremely large datasets or complex calculations. Its collaborative features are particularly useful for team projects.
- Bloomberg Terminal: A comprehensive financial data and analytics platform providing access to real-time market data, news, and analytical tools. It’s highly powerful but expensive and requires specialized training. It is primarily used by professionals in the financial industry.
- Capital IQ: Another data and analytics platform offering company financials, industry benchmarks, and valuation tools. It is used for in-depth company research and financial analysis, often integrated into larger modeling projects.
- Dedicated Financial Modeling Platforms (e.g., Alteryx, Tableau): These platforms offer advanced features such as automated reporting, scenario planning, and risk analysis. They are generally more expensive than spreadsheet software but can significantly improve efficiency and accuracy for complex models. These platforms often integrate with other data sources and offer more robust data visualization capabilities.
Spreadsheet Software vs. Dedicated Financial Modeling Platforms
A key decision for financial modellers is choosing between general-purpose spreadsheet software and dedicated financial modeling platforms. This decision significantly impacts the model’s efficiency, scalability, and overall quality.
Feature | Spreadsheet Software (e.g., Excel, Google Sheets) | Dedicated Financial Modeling Platforms |
---|---|---|
Cost | Relatively low | Significantly higher |
Ease of Use | Generally easy to learn | Steeper learning curve |
Functionality | Basic to intermediate modeling capabilities | Advanced features, automation, and collaboration tools |
Scalability | Can be challenging for large models | Designed for large, complex models |
Error Management | Prone to errors if not used carefully | Often includes built-in error checking and validation |
Collaboration | Limited collaboration features | Robust collaboration features |
Advantages and Disadvantages of Different Software Tools
The choice of software depends heavily on the specific modeling task. For simple models, spreadsheets may suffice; for complex projects, dedicated platforms are often necessary. Understanding the strengths and weaknesses of each tool is crucial for effective modeling.
For instance, using Excel for a simple budgeting model is efficient and straightforward. However, attempting to build a complex discounted cash flow model with many interconnected variables in Excel can quickly become unwieldy and error-prone. A dedicated platform would be better suited for such a task due to its advanced features and error-checking capabilities.
Tips and Tricks for Efficient Financial Modeling Software Use
Maximizing the efficiency and accuracy of financial models requires leveraging the full potential of the chosen software. Several best practices contribute to streamlined modeling workflows.
- Use clear and consistent naming conventions: This improves readability and reduces errors.
- Employ robust error checking and validation: Regularly review formulas and data for accuracy.
- Leverage built-in features: Utilize functions and tools to automate calculations and reduce manual effort.
- Employ data validation techniques: Restrict input values to ensure data accuracy.
- Regularly back up your work: This safeguards against data loss.
- Document your model thoroughly: Include clear explanations of formulas, assumptions, and data sources.
- Use version control: Track changes to the model and revert to previous versions if needed.
Best Practices for Presentation and Communication
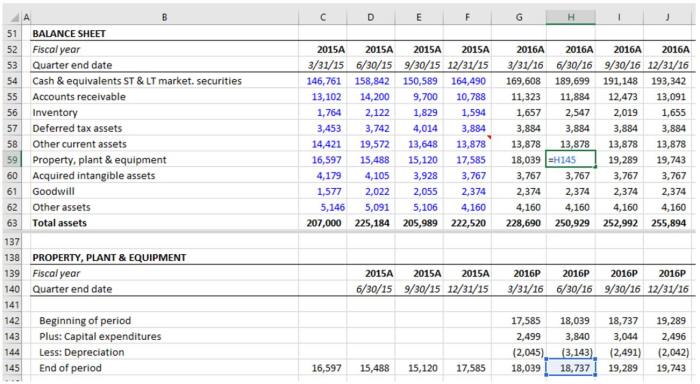
Effective communication of financial model outputs is crucial for their successful application. A well-designed presentation, coupled with clear and concise documentation, ensures that stakeholders, regardless of their financial expertise, can understand and utilize the model’s insights. This section Artikels best practices for achieving this.
Template for Presenting Financial Model Outputs
A standardized template ensures consistency and clarity in presenting model outputs. The following table illustrates a basic framework, adaptable to specific needs. Note that visual aids, such as charts and graphs, should supplement tabular data whenever appropriate.
Metric | Base Case | Best Case | Worst Case | Year 1 | Year 2 | Year 3 | Year 4 | Year 5 |
---|---|---|---|---|---|---|---|---|
Revenue | $1,000,000 | $1,200,000 | $800,000 | $200,000 | $250,000 | $300,000 | $350,000 | $400,000 |
Cost of Goods Sold | $600,000 | $720,000 | $480,000 | $120,000 | $150,000 | $180,000 | $210,000 | $240,000 |
Gross Profit | $400,000 | $480,000 | $320,000 | $80,000 | $100,000 | $120,000 | $140,000 | $160,000 |
Operating Expenses | $200,000 | $240,000 | $160,000 | $40,000 | $50,000 | $60,000 | $70,000 | $80,000 |
Operating Income | $200,000 | $240,000 | $160,000 | $40,000 | $50,000 | $60,000 | $70,000 | $80,000 |
Communicating Complex Financial Information to Diverse Audiences
Tailoring the communication style to the audience is paramount. For example, a presentation to senior management might focus on high-level summaries and key performance indicators (KPIs), while a presentation to a technical team might delve into the model’s underlying assumptions and methodologies. Using clear, concise language and avoiding technical jargon where possible is crucial for all audiences. Visual aids such as charts and graphs are especially helpful in conveying complex data in an easily digestible format. For instance, a bar chart comparing projected revenue against different scenarios would be far more effective than a table of numbers alone.
Importance of Clear and Concise Model Documentation
Comprehensive documentation is essential for model transparency, maintainability, and auditability. It should include a detailed description of the model’s purpose, assumptions, data sources, calculations, and limitations. Well-structured documentation facilitates collaboration, allows for easier troubleshooting, and ensures that the model remains useful and relevant over time. For instance, clearly documenting the source of each input variable prevents confusion and errors in the future. A well-documented model can be easily understood and maintained by others, even if the original creator is no longer involved.
Checklist for Reviewing and Validating Financial Models
A rigorous review process is crucial to ensure model accuracy and reliability. The following checklist provides a framework:
- Data Validation: Verify the accuracy and completeness of all input data.
- Formula Verification: Check the accuracy of all formulas and calculations.
- Scenario Analysis: Test the model’s sensitivity to changes in key assumptions.
- Logical Consistency: Ensure that the model’s outputs are logically consistent with its inputs and assumptions.
- Documentation Review: Review the model’s documentation for completeness and clarity.
- Peer Review: Have another experienced modeler review the model.
Ethical Considerations in Financial Modeling
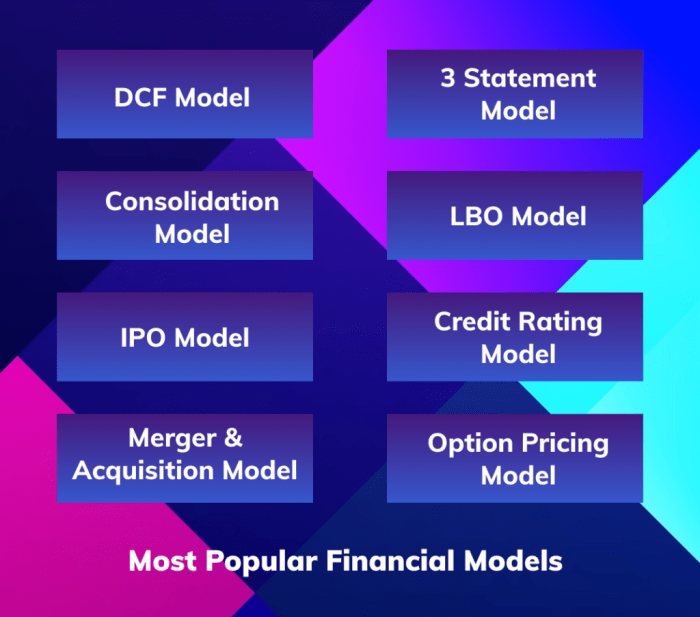
Financial modeling, while a powerful tool for decision-making, carries significant ethical responsibilities. The potential for misuse and the impact on stakeholders necessitate a strong ethical framework guiding the creation and use of these models. Ignoring ethical considerations can lead to inaccurate predictions, flawed investment strategies, and ultimately, financial losses or even legal repercussions.
Building and using financial models ethically involves a commitment to transparency, accuracy, and objectivity. Modelers have a responsibility to ensure their work is free from bias, avoids manipulation, and is used responsibly by clients and stakeholders.
Potential Ethical Dilemmas and Solutions
Ethical dilemmas in financial modeling often arise from pressures to produce desired results, conflicts of interest, or a lack of transparency. For example, a modeler might be pressured by a client to manipulate assumptions to achieve a specific outcome, such as inflating projected revenues to secure funding. Solutions include establishing clear guidelines on acceptable modeling practices, fostering a culture of ethical behavior within organizations, and utilizing independent review processes to verify model integrity. Another example is the use of overly optimistic or unrealistic assumptions to present a more favorable financial picture, which can mislead investors. To mitigate this, modelers should clearly document all assumptions, sensitivity analysis, and limitations of the model, promoting transparency and reducing the likelihood of misinterpretation.
Transparency and Accuracy in Financial Modeling
Transparency and accuracy are cornerstones of ethical financial modeling. Transparency involves clearly documenting all data sources, assumptions, methodologies, and limitations of the model. This allows stakeholders to understand the model’s strengths and weaknesses, assess its validity, and identify potential biases. Accuracy demands rigorous attention to detail, meticulous data validation, and the use of appropriate modeling techniques. Inaccurate models can lead to flawed investment decisions, misallocation of resources, and significant financial losses. For example, a model used to value a company that fails to account for crucial industry trends or competitive pressures will produce inaccurate results, potentially leading to an overvaluation of the company.
Responsibilities of Financial Modelers in Ensuring Model Integrity
Financial modelers bear a significant responsibility for ensuring the integrity of their models. This includes maintaining objectivity, avoiding conflicts of interest, and adhering to professional standards. They must ensure that the model is fit for its intended purpose, that the data used is reliable and accurate, and that the model’s limitations are clearly communicated. Modelers should also be prepared to defend their work and explain their methodologies to stakeholders. Failure to meet these responsibilities can damage professional reputation and lead to legal or regulatory consequences. A modeler who knowingly uses inaccurate data or manipulates assumptions to achieve a desired outcome is acting unethically and potentially illegally.
Best Practices for Maintaining Ethical Standards
Maintaining ethical standards requires a proactive approach. Best practices include: regularly reviewing and updating models to reflect changes in market conditions and data; using robust validation techniques to ensure data accuracy; conducting sensitivity analysis to assess the impact of changes in assumptions; clearly documenting all model inputs, assumptions, and outputs; obtaining independent reviews of complex models; and adhering to relevant professional codes of conduct. Furthermore, fostering a culture of open communication and ethical awareness within the organization is crucial for promoting responsible modeling practices. For instance, implementing a formal process for reviewing and approving models before they are used for decision-making can help to prevent unethical practices.
Illustrative Example
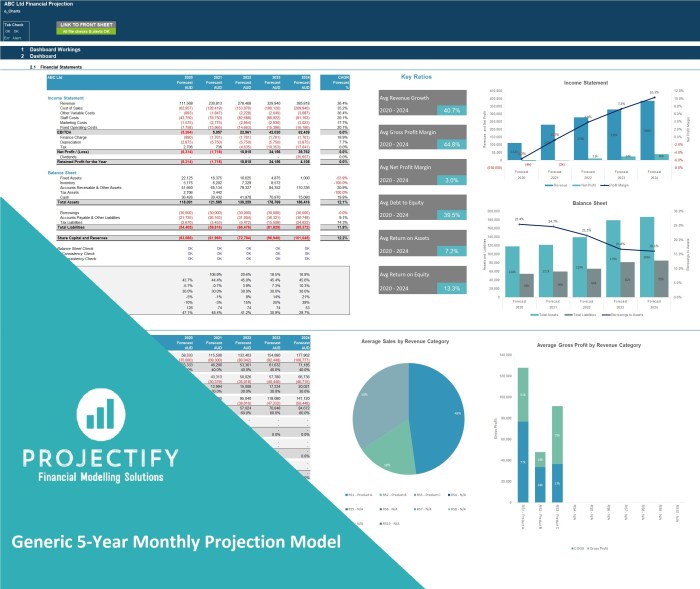
This section presents a detailed walkthrough of a hypothetical financial model for a small bakery, “Sweet Success,” illustrating key modeling concepts discussed previously. We’ll examine its assumptions, calculations, and outputs, including a sensitivity analysis to assess the model’s robustness.
Model Assumptions
The model assumes Sweet Success sells three primary products: cupcakes, cookies, and brownies. We’ve estimated unit sales based on historical data and projected growth, considering seasonal variations. Pricing is based on current market rates and anticipated adjustments. Cost of goods sold (COGS) includes ingredients, packaging, and direct labor. Operating expenses encompass rent, utilities, salaries, marketing, and administrative costs. These are projected based on current expenses and anticipated changes. The model assumes a constant tax rate throughout the projection period. A 10% annual growth rate in sales is assumed for the first three years, followed by a more conservative 5% growth rate thereafter.
Key Calculations
The model calculates revenue by multiplying unit sales by the selling price for each product. COGS is calculated by multiplying unit sales by the cost per unit. Gross profit is the difference between revenue and COGS. Operating expenses are subtracted from gross profit to arrive at operating income. Interest expense, if any, is then deducted to determine earnings before taxes (EBT). Taxes are calculated at the assumed tax rate, and finally, net income is determined. The model also projects cash flow, considering changes in working capital (accounts receivable, inventory, accounts payable) and capital expenditures. The projected cash flow is used to evaluate the bakery’s liquidity and funding needs. A simple discounted cash flow (DCF) analysis is performed to estimate the business’s present value.
Model Outputs
The model outputs include projected income statements, balance sheets, and cash flow statements for a five-year period. Key performance indicators (KPIs) such as gross profit margin, net profit margin, return on assets (ROA), and return on equity (ROE) are calculated and displayed. The DCF analysis provides an estimated business valuation based on projected future cash flows and a chosen discount rate (e.g., 10%). A break-even analysis is also included, showing the sales volume needed to cover all costs. These outputs provide a comprehensive overview of the bakery’s financial health and future prospects.
Sensitivity Analysis
A sensitivity analysis is performed to assess the impact of changes in key assumptions on the model’s outputs. For instance, we vary the sales growth rate (from 5% to 15%) and the cost of goods sold (by ±10%) to observe their effects on net income and business valuation. This helps understand the uncertainty associated with the projections and identify the most critical assumptions. For example, a 15% increase in COGS reduces the projected net income significantly, highlighting the importance of managing costs.
Interpretations and Insights
The model’s results suggest that Sweet Success is projected to be profitable within the five-year forecast period. However, the sensitivity analysis reveals that the business is particularly sensitive to changes in COGS. Therefore, efficient inventory management and cost control are crucial for achieving the projected profitability. The DCF analysis provides a valuation range, depending on the chosen discount rate and sales growth assumptions. This valuation can be used for various purposes, such as securing financing or making investment decisions. The break-even analysis indicates the sales volume required to achieve profitability, providing a benchmark for performance monitoring. The model highlights the importance of careful planning and management to achieve financial success.
Summary
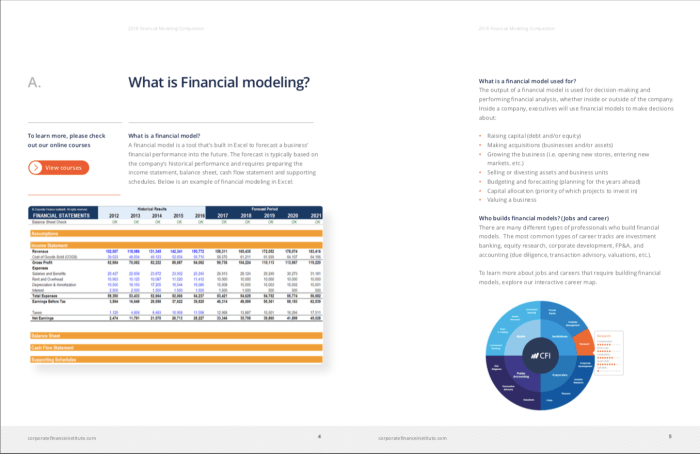
Building effective financial models requires a blend of technical expertise and sound judgment. By adhering to best practices, employing appropriate software, and prioritizing ethical considerations, you can create models that are not only accurate and reliable but also contribute to informed and responsible decision-making. This guide provides a strong foundation for building your skills and confidence in the world of financial modeling. Remember that continuous learning and adaptation are key to staying ahead in this ever-evolving field.
Query Resolution
What is the difference between a deterministic and a stochastic model?
A deterministic model uses fixed inputs to produce a single, predictable outcome. A stochastic model incorporates random variables, resulting in a range of possible outcomes.
How can I improve the accuracy of my financial projections?
Use reliable data sources, incorporate sensitivity analysis, regularly review and update assumptions, and validate your model against historical data.
What are some common mistakes to avoid in financial modeling?
Circular references, incorrect formulas, inconsistent data, neglecting risk factors, and poor documentation are common pitfalls.
What is the best software for financial modeling?
The best software depends on your needs and budget. Popular choices include Excel, Google Sheets, and dedicated financial modeling platforms like Alteryx or Anaplan. Consider factors like scalability, functionality, and ease of use.