Navigating the complexities of the financial markets requires a strategic approach to wealth management. Investment portfolio optimization is the cornerstone of this strategy, enabling investors to maximize returns while mitigating risk. This exploration delves into various techniques, from established Modern Portfolio Theory (MPT) to more contemporary approaches like Post-Modern Portfolio Theory (PMPT) and factor-based investing, equipping readers with a comprehensive understanding of portfolio construction and management.
Understanding your risk tolerance, defining your investment goals, and selecting appropriate asset classes are crucial first steps. We’ll examine how different investor profiles necessitate tailored strategies and how sophisticated mathematical models and risk management techniques can refine portfolio construction. Furthermore, we will consider the impact of behavioral biases and the growing importance of ESG (Environmental, Social, and Governance) considerations in shaping modern investment portfolios.
Introduction to Investment Portfolio Optimization
Investment portfolio optimization is the process of constructing a portfolio of assets that maximizes returns for a given level of risk, or minimizes risk for a given level of return. It’s a strategic approach to investing that aims to achieve the best possible outcome based on an investor’s specific circumstances and objectives. This involves careful consideration of various factors, including asset allocation, diversification, and risk tolerance.
Portfolio optimization aims to achieve several key goals. Primarily, it seeks to maximize the expected return on investment while simultaneously minimizing the associated risk. This balance is crucial, as higher returns often come with higher risk. Other goals might include meeting specific financial targets, such as retirement savings or funding a child’s education, or adhering to specific regulatory constraints. Ultimately, the goal is to create a portfolio tailored to the investor’s individual needs and risk profile.
Diversification plays a crucial role in portfolio optimization. By spreading investments across different asset classes (stocks, bonds, real estate, etc.) and sectors, diversification helps to reduce the overall risk of the portfolio. If one asset class performs poorly, the losses can be offset by gains in other areas, leading to a more stable and predictable portfolio performance. This doesn’t eliminate risk entirely, but it significantly mitigates the impact of any single investment’s underperformance. Effective diversification considers not only asset classes but also geographic locations and individual company characteristics.
Investor Profiles and Portfolio Optimization Strategies
The optimal portfolio strategy varies significantly depending on the investor’s profile. The table below illustrates how different risk tolerances and investment horizons influence portfolio allocation:
Investor Profile | Risk Tolerance | Investment Horizon | Portfolio Optimization Strategy |
---|---|---|---|
Conservative Investor | Low | Short-term (e.g., less than 5 years) | Focus on low-risk, liquid assets such as high-quality bonds, money market funds, and short-term government securities. Limited exposure to equities. |
Moderate Investor | Moderate | Medium-term (e.g., 5-10 years) | Balanced portfolio with a mix of stocks and bonds. May include some alternative investments, depending on risk appetite. Asset allocation shifts towards equities as the investment horizon lengthens. |
Aggressive Investor | High | Long-term (e.g., more than 10 years) | Higher allocation to equities, including growth stocks and emerging markets. May incorporate more alternative investments such as private equity or hedge funds. Higher tolerance for short-term volatility in pursuit of higher long-term returns. |
Retirement Investor (near retirement) | Low to Moderate | Short-term | Focus on preserving capital and generating income. Higher allocation to fixed-income securities and dividend-paying stocks. Lower risk tolerance to protect accumulated savings. |
Modern Portfolio Theory (MPT)
Modern Portfolio Theory (MPT), developed by Harry Markowitz in the 1950s, revolutionized investment thinking by emphasizing diversification and the importance of considering the relationship between assets within a portfolio. Unlike earlier approaches that focused solely on individual asset returns, MPT provides a framework for constructing portfolios that optimize risk and return based on the statistical properties of asset returns.
Key Assumptions of MPT
MPT rests on several key assumptions. Investors are rational and risk-averse, meaning they prefer higher returns for a given level of risk and lower risk for a given level of return. They base investment decisions on expected returns and variances (a measure of risk). Returns are normally distributed, implying that past performance can be used to predict future performance, although with inherent uncertainty. Finally, the market is efficient, meaning all relevant information is reflected in asset prices, and no investor can consistently outperform the market through superior information. It’s crucial to acknowledge that these assumptions are simplifications of reality, yet they provide a useful framework for portfolio construction.
Risk and Return in MPT
In MPT, risk is quantified by the standard deviation of returns, representing the volatility or fluctuation of an asset’s price. Return is the expected profit from an investment, typically expressed as an annual percentage. The theory demonstrates that diversification, the inclusion of assets with low or negative correlations, can reduce portfolio risk without necessarily sacrificing return. By combining assets that don’t move in perfect unison, investors can “smooth out” the overall portfolio performance, lessening the impact of negative returns in any single asset. This principle is at the heart of MPT’s effectiveness.
The Efficient Frontier
The efficient frontier is a graphical representation of the optimal portfolios that offer the highest expected return for a given level of risk or the lowest risk for a given level of expected return. Portfolios lying on the efficient frontier are considered “efficient” because they offer the best possible risk-return trade-off. Portfolios below the efficient frontier are suboptimal, offering lower returns for the same level of risk or higher risk for the same level of return. The efficient frontier is crucial because it helps investors identify the optimal portfolio that aligns with their risk tolerance and return objectives.
Example Portfolio using MPT Principles
Let’s consider a simple example portfolio using three asset classes: Stocks (represented by a broad market index fund), Bonds (represented by a long-term government bond fund), and Real Estate (represented by a Real Estate Investment Trust (REIT) fund). Assume the following historical data (simplified for illustrative purposes):
Asset Class | Expected Return | Standard Deviation | Correlation with Stocks | Correlation with Bonds |
---|---|---|---|---|
Stocks | 10% | 15% | 1.0 | -0.2 |
Bonds | 5% | 5% | -0.2 | 1.0 |
Real Estate | 8% | 10% | 0.6 | 0.1 |
A moderately conservative portfolio might allocate 50% to Stocks, 30% to Bonds, and 20% to Real Estate. This allocation aims to balance higher potential returns from stocks with the stability of bonds, while incorporating real estate for diversification and potentially higher returns compared to bonds. The rationale is to achieve a balance between risk and return, taking advantage of the diversification benefits offered by these assets with varying correlations. The actual allocation would need to be refined using sophisticated optimization techniques and updated regularly based on market conditions and risk tolerance. Note: This is a simplified example and does not constitute investment advice. Actual portfolio construction requires professional financial guidance.
Post-Modern Portfolio Theory (PMPT)
Post-Modern Portfolio Theory (PMPT) offers a compelling alternative to the traditional Modern Portfolio Theory (MPT), addressing some of its inherent limitations. While MPT relies heavily on assumptions of normality in asset returns and focuses primarily on minimizing risk as measured by variance, PMPT adopts a more nuanced approach, acknowledging the complexities of real-world market behavior. This approach allows for a more robust and potentially more profitable portfolio construction strategy.
PMPT acknowledges and incorporates the non-normality of asset returns, a key departure from MPT. This means that PMPT doesn’t solely rely on the mean and variance of returns to characterize the risk profile of an investment. Instead, it utilizes a broader range of statistical measures to capture the full spectrum of potential outcomes, including extreme events (like market crashes) that can significantly impact portfolio performance. The focus shifts from minimizing variance to managing downside risk more effectively.
Comparison of MPT and PMPT
MPT and PMPT differ significantly in their underlying assumptions and methodologies. MPT assumes that asset returns are normally distributed, meaning they follow a bell curve. This assumption simplifies risk calculation to focusing solely on variance (standard deviation). PMPT, however, acknowledges that asset returns often exhibit fat tails and skewness, implying a higher probability of extreme events than predicted by a normal distribution. This difference leads to contrasting approaches to portfolio optimization. MPT aims to optimize the Sharpe ratio, maximizing risk-adjusted return, while PMPT often prioritizes downside risk mitigation and focuses on measures like Sortino ratio or downside deviation.
Limitations of MPT Addressed by PMPT
MPT’s reliance on the assumption of normally distributed returns is its most significant limitation. Real-world asset returns frequently deviate from this assumption, particularly during periods of market stress. The infrequent but impactful occurrence of extreme events, which MPT underestimates, can lead to substantial portfolio losses. Furthermore, MPT’s focus on variance as the sole measure of risk overlooks the asymmetry of risk; investors are often more concerned with downside risk than upside risk. PMPT directly addresses these issues by incorporating measures that capture fat tails, skewness, and specifically quantify downside risk.
Examples of PMPT Techniques
Several techniques are employed within the framework of PMPT. One common approach involves using non-parametric methods that don’t rely on assumptions about the distribution of returns. These techniques often employ historical data to simulate potential future scenarios and assess the portfolio’s performance under various conditions. Another approach involves utilizing downside risk measures such as Sortino ratio, which focuses on the downside deviation below a target return, instead of the overall variance. Furthermore, techniques like Monte Carlo simulations can be used to model different scenarios, incorporating the possibility of extreme events and generating a more comprehensive risk profile.
Practical Applications of PMPT in Real-World Scenarios
PMPT finds application in various real-world investment scenarios. For instance, in managing hedge fund portfolios, where downside protection is paramount, PMPT techniques can be instrumental in constructing strategies that aim to limit potential losses during market downturns while still capturing upside potential. Similarly, in retirement planning, where preserving capital is crucial, PMPT can help construct portfolios that balance growth with downside risk mitigation. Another practical application is in portfolio insurance strategies, where PMPT models can help determine optimal hedging strategies to protect against significant market declines. For example, a portfolio manager might use PMPT to determine the optimal allocation between stocks and bonds to achieve a desired level of downside risk protection while still maintaining sufficient growth potential. The specific techniques used will vary depending on the investor’s risk tolerance, investment horizon, and investment goals.
Factor-Based Investing
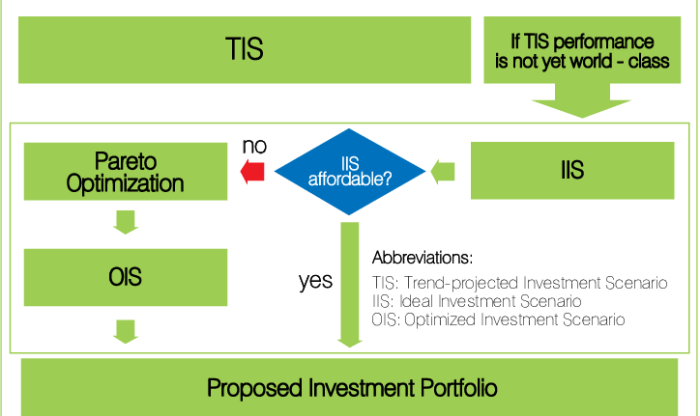
Factor-based investing represents a significant shift from traditional portfolio optimization strategies. Instead of solely focusing on individual stock selection or market capitalization, it leverages identified market factors to construct portfolios. This approach aims to generate superior risk-adjusted returns by systematically exploiting predictable patterns in asset prices linked to these factors. The core principle lies in the belief that certain characteristics (factors) consistently predict future returns, allowing investors to build portfolios tilted towards those characteristics.
Factor investing integrates seamlessly with portfolio optimization techniques. Instead of optimizing solely based on historical correlations (as in Modern Portfolio Theory), factor-based optimization considers the expected returns associated with various factors. This allows for the construction of portfolios that are not only diversified but also strategically positioned to capitalize on anticipated factor premiums. The optimization process then seeks to find the optimal allocation across different factor exposures to achieve the desired risk-return profile, potentially improving Sharpe ratios and other performance metrics.
Common Factors in Factor-Based Investing
Several factors have demonstrated a consistent ability to predict future returns. Understanding these factors is crucial for constructing effective factor-based portfolios. The selection and weighting of these factors depend on the specific investment strategy and risk tolerance.
- Value: Value investing focuses on companies that appear undervalued relative to their fundamental metrics, such as book value, earnings, or cash flow. Value stocks often exhibit lower price-to-earnings (P/E) ratios, price-to-book (P/B) ratios, and price-to-sales (P/S) ratios compared to growth stocks. Historically, value stocks have demonstrated a tendency to outperform growth stocks over the long term, although this relationship can vary over time.
- Growth: Growth investing centers on companies exhibiting high earnings growth and expansion potential. These companies often have high P/E ratios and high price-to-sales ratios, reflecting investor expectations of future growth. Growth stocks can provide significant returns during periods of economic expansion but may be more vulnerable during economic downturns.
- Momentum: Momentum investing identifies stocks that have performed well recently and bets on their continued upward trajectory. This strategy capitalizes on the tendency of past winners to continue outperforming for a certain period. However, momentum strategies can be susceptible to reversals, particularly in market corrections.
- Size: Size, often measured by market capitalization, has shown a historical tendency for smaller-cap stocks to outperform larger-cap stocks. This is often attributed to higher growth potential and increased risk premiums associated with smaller companies.
- Quality: Quality investing focuses on companies with strong balance sheets, high profitability, and consistent earnings growth. These firms are generally considered less risky and offer a more stable return profile.
Comparison of Factor Models
Various factor models exist, each with its unique set of factors and weighting methodologies. The Fama-French three-factor model, for instance, incorporates market risk, size, and value factors. More sophisticated models, such as the Carhart four-factor model, add momentum as an additional factor. The choice of model depends on the investor’s objectives and beliefs regarding the predictive power of different factors. Some models incorporate dozens of factors, attempting to capture a wider range of market dynamics. The effectiveness of each model is subject to ongoing debate and empirical testing. The choice often depends on the specific investment goals and risk tolerance.
Constructing an Optimized Factor-Based Portfolio
Constructing an optimized factor-based portfolio involves several steps. First, the investor selects a factor model that aligns with their investment philosophy and risk tolerance. Then, they determine the desired exposure to each factor, considering the expected returns and risk associated with each. This might involve using optimization techniques such as mean-variance optimization or more advanced approaches that account for factor correlations and higher moments of returns. Finally, the portfolio is constructed by selecting individual securities that have high loadings on the desired factors, while also considering diversification across sectors and other relevant risk factors. The portfolio’s composition is then regularly rebalanced to maintain the desired factor exposures and risk profile. This process may involve sophisticated quantitative methods, such as linear programming or quadratic programming, to find the optimal allocation of assets across the selected factors while minimizing risk and maximizing expected returns. For example, an investor might target a portfolio with high exposure to value and quality factors, potentially reducing overall portfolio volatility while seeking superior returns compared to a market-cap weighted benchmark.
Risk Management Techniques
Effective risk management is crucial for successful portfolio optimization. Understanding and mitigating various risks is paramount to achieving investment goals while preserving capital. This section will explore different types of investment risks, strategies for managing them, and illustrate risk mitigation techniques within portfolio construction.
Types of Investment Risks
Investment risk encompasses a wide range of potential negative impacts on an investment’s value. These risks can be broadly categorized, though they often overlap and interact. Systematic risks, also known as market risks, affect the entire market and cannot be diversified away. Unsystematic risks, on the other hand, are specific to individual assets and can be reduced through diversification.
Risk Management Strategies
Several strategies can be employed to manage investment risks effectively. Diversification, arguably the most fundamental, spreads investments across different asset classes, sectors, and geographies to reduce the impact of any single asset’s poor performance. Hedging involves using financial instruments to offset potential losses from adverse price movements. For example, purchasing put options on a stock you own provides insurance against a price drop. Another strategy is active management, where portfolio managers actively adjust the portfolio based on market conditions and risk assessments. This approach aims to outperform a passive strategy, such as simply tracking a market index. Finally, risk budgeting explicitly allocates a certain amount of risk to different parts of a portfolio, helping to maintain an overall acceptable risk level.
Risk Mitigation Techniques in Portfolio Construction
Risk mitigation is integrated into portfolio construction through various techniques. Diversification, as mentioned, is key. This can involve allocating funds to different asset classes (stocks, bonds, real estate), sectors (technology, healthcare, energy), and geographies (developed markets, emerging markets). Another technique is strategic asset allocation, where the long-term allocation to different asset classes is determined based on risk tolerance and investment goals. Tactical asset allocation, on the other hand, involves making short-term adjustments to asset allocation based on market conditions. Stress testing, which simulates the portfolio’s performance under various adverse market scenarios, allows for identification of potential weaknesses and informs adjustments to reduce vulnerability. Finally, using derivatives such as options and futures can be used for hedging specific risks. For example, a portfolio heavily invested in emerging market equities could use currency futures to hedge against adverse currency movements.
Risk Measures and Interpretations
Understanding different risk measures is essential for effective portfolio management. The following table illustrates some key measures and their interpretations:
Risk Measure | Description | Interpretation | Example |
---|---|---|---|
Standard Deviation | Measures the dispersion of returns around the average return. | Higher standard deviation indicates higher volatility and risk. | A stock with a standard deviation of 20% is more volatile than one with 10%. |
Beta | Measures the sensitivity of an asset’s returns to market returns. | A beta greater than 1 indicates higher volatility than the market; less than 1 indicates lower volatility. | A beta of 1.5 suggests the asset’s price will move 1.5 times as much as the market index. |
Sharpe Ratio | Measures risk-adjusted return, considering the excess return relative to the risk-free rate per unit of volatility. | Higher Sharpe ratio indicates better risk-adjusted performance. | A Sharpe ratio of 1.0 is generally considered good. |
Value at Risk (VaR) | Estimates the potential loss in value of an asset or portfolio over a specific time horizon with a given confidence level. | Indicates the maximum potential loss under normal market conditions. | A VaR of $10,000 at a 95% confidence level means there is a 5% chance of losing at least $10,000. |
Portfolio Rebalancing Strategies
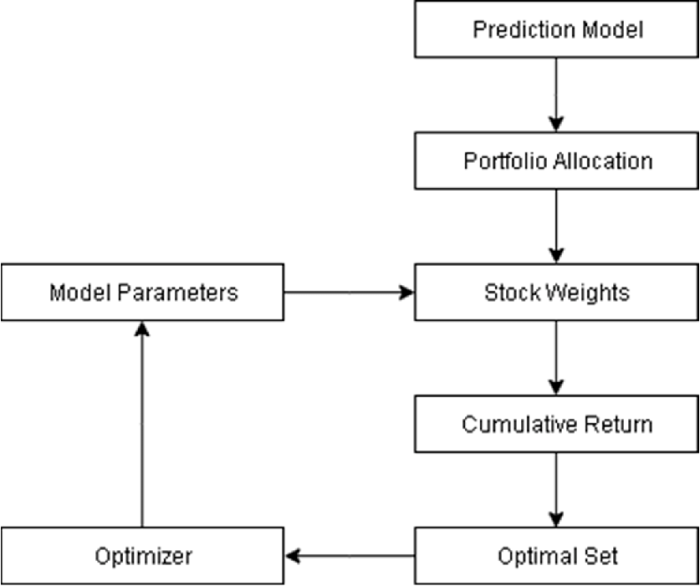
Maintaining a well-diversified investment portfolio requires more than just initial asset allocation. Over time, market fluctuations will cause the proportions of your assets to drift from your target allocation. This is where portfolio rebalancing comes in, a crucial strategy to help manage risk and potentially enhance returns. Rebalancing involves adjusting your portfolio to restore its original asset allocation percentages.
Portfolio rebalancing is important because it helps investors maintain their desired level of risk and potentially capitalize on market fluctuations. Without rebalancing, a portfolio that initially had a balanced risk profile could become overly concentrated in certain asset classes after periods of strong performance, increasing its risk exposure. Conversely, periods of underperformance can lead to a portfolio that is overly cautious, potentially missing out on future growth opportunities. Regular rebalancing helps mitigate these issues by systematically bringing the portfolio back to its target allocation.
Time-Based Rebalancing
Time-based rebalancing involves adjusting your portfolio at predetermined intervals, regardless of market performance. This approach offers simplicity and discipline. For example, an investor might rebalance their portfolio annually, semi-annually, or even quarterly. The frequency depends on the investor’s risk tolerance and investment goals. A more frequent rebalancing schedule generally results in smaller adjustments and a greater degree of risk management. However, it also means incurring more transaction costs. Conversely, less frequent rebalancing involves larger adjustments and potentially greater risk exposure but reduces transaction costs.
Value-Based Rebalancing
Value-based rebalancing, also known as percentage-based rebalancing, triggers rebalancing when the deviation from the target allocation reaches a specified threshold. For instance, an investor might rebalance when the allocation of a particular asset class deviates by 5% or 10% from its target. This method is more reactive than time-based rebalancing, adjusting only when necessary, thus minimizing transaction costs. However, it might require more active monitoring of the portfolio.
Comparison of Rebalancing Strategies
Time-based and value-based rebalancing strategies each have their own advantages and disadvantages. Time-based rebalancing offers simplicity and consistency, while value-based rebalancing is more efficient in terms of transaction costs and focuses on maintaining the target allocation more precisely. The optimal strategy depends on individual circumstances, including risk tolerance, investment goals, and the cost of transactions. Generally, investors with higher risk tolerance and lower transaction costs might prefer more frequent time-based or value-based rebalancing, while those with lower risk tolerance and higher transaction costs might opt for less frequent rebalancing. Empirical evidence on the superior performance of one strategy over another is inconclusive and depends heavily on market conditions and specific portfolio composition.
Sample Rebalancing Schedule
Consider a hypothetical portfolio with a target allocation of 60% stocks and 40% bonds. A time-based rebalancing schedule might involve rebalancing annually. At the end of each year, the investor would review the portfolio’s performance. If the stock allocation has drifted to 70%, for example, the investor would sell 10% of stocks and buy 10% of bonds to restore the 60/40 allocation. A value-based approach might trigger a rebalance when either the stock or bond allocation deviates by 5% from the target. Therefore, a rebalance would be initiated if the stock allocation falls below 55% or rises above 65%, or if the bond allocation falls below 35% or rises above 45%. The choice between these approaches depends on the investor’s preference for simplicity versus cost efficiency and the degree of active management they’re willing to undertake.
Tax-Efficient Investing Strategies
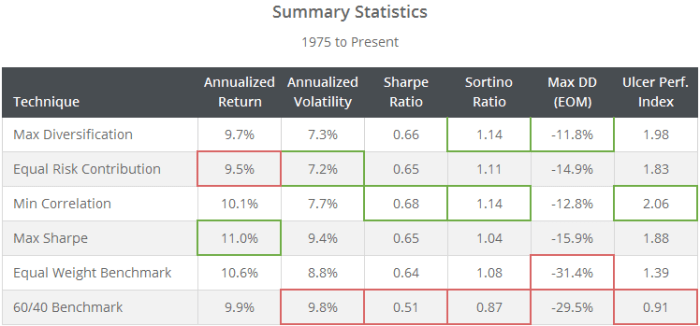
Taxes significantly impact investment returns, reducing the overall profit generated. Understanding and employing tax-efficient strategies is crucial for maximizing long-term growth. Failing to account for taxes can lead to a substantial erosion of investment gains, leaving investors with less than they might have otherwise achieved.
Tax-efficient investing aims to minimize the tax burden on investment income and capital gains, allowing more of your investment returns to compound over time. This involves strategic choices regarding asset allocation, timing of transactions, and utilizing tax-advantaged accounts. The goal is not to avoid taxes entirely, but to legally reduce your tax liability to its lowest possible level, thus increasing your after-tax returns.
Impact of Taxes on Investment Returns
Taxes affect investment returns in several ways. Capital gains taxes are levied on profits from the sale of assets like stocks or bonds. Dividend taxes apply to income received from dividend-paying stocks. Interest income from bonds is also subject to taxation. The specific tax rates vary depending on the investor’s income bracket and the holding period of the asset (short-term vs. long-term capital gains). High tax brackets can significantly diminish investment growth, especially in volatile markets where frequent trading might be necessary. For example, an investor in a high tax bracket might see a substantial portion of their gains eaten away by taxes, leaving them with less to reinvest and compound. This emphasizes the need for careful tax planning.
Tax-Efficient Investment Strategies to Minimize Tax Liabilities
Minimizing tax liabilities requires a proactive approach to investment management. This includes strategically choosing investments with lower tax implications, such as municipal bonds (which are often tax-exempt at the federal level and sometimes at the state level, depending on where the bond is issued), or focusing on investments that generate long-term capital gains (which are often taxed at a lower rate than ordinary income). Tax-loss harvesting, a strategy that involves selling losing investments to offset capital gains, can also be effective in reducing overall tax burdens. Careful timing of trades can help minimize tax exposure. For example, delaying the sale of an appreciated asset until a lower tax bracket is reached can result in substantial savings.
Tax-Advantaged Accounts
Several tax-advantaged accounts offer significant benefits for long-term investors. These accounts allow investments to grow tax-deferred or tax-free, depending on the specific type of account.
- 401(k) plans: Employer-sponsored retirement savings plans offering tax-deferred growth. Contributions may be tax-deductible, and taxes are only paid upon withdrawal in retirement.
- Individual Retirement Accounts (IRAs): Retirement savings plans offering tax advantages, including Traditional IRAs (tax-deductible contributions, taxed withdrawals in retirement) and Roth IRAs (after-tax contributions, tax-free withdrawals in retirement).
- Health Savings Accounts (HSAs): Accounts for individuals enrolled in high-deductible health plans. Contributions are tax-deductible, grow tax-free, and can be withdrawn tax-free for qualified medical expenses.
- 529 Plans: Education savings plans offering tax-advantaged growth for qualified education expenses. Earnings grow tax-free, and withdrawals are tax-free when used for eligible education costs.
Tax Optimization Techniques
A well-rounded tax optimization strategy often involves a combination of techniques. Careful consideration should be given to the investor’s individual circumstances and financial goals.
- Tax-Loss Harvesting: Offsetting capital gains with capital losses to reduce overall tax liability.
- Asset Location: Strategically placing assets in different accounts (taxable, tax-deferred, tax-free) to optimize tax efficiency.
- Tax-Efficient Mutual Funds and ETFs: Investing in funds that minimize capital gains distributions.
- Bunching Deductions: Concentrating deductions in a single year to maximize tax savings.
- Gifting and Estate Planning: Utilizing gifting strategies and estate planning techniques to minimize estate taxes.
Quantitative Techniques for Portfolio Optimization
Quantitative portfolio optimization leverages the power of mathematical models and computational algorithms to construct investment portfolios that efficiently balance risk and return. This approach moves beyond intuitive or rule-of-thumb methods, offering a more rigorous and data-driven framework for investment decision-making. The core principle lies in defining an objective function (often maximizing return for a given level of risk or minimizing risk for a given level of return) and subject to constraints (such as budget limitations or asset allocation targets). This framework allows for the systematic exploration of a vast number of potential portfolio combinations to identify the optimal solution.
Mathematical models are fundamental to quantitative portfolio optimization. These models formally represent the investor’s objectives and constraints, translating them into a mathematical problem that can be solved using computational techniques. The models incorporate factors such as expected returns, variances, and covariances of assets, allowing for a precise quantification of risk and return trade-offs. Different models cater to varying levels of complexity and incorporate diverse assumptions about market behavior. For instance, a simple model might assume normally distributed returns, while a more sophisticated model might account for non-normality and other stylized facts of financial markets.
Mathematical Models in Portfolio Optimization
Mathematical models used in portfolio optimization typically involve the formulation of an objective function and a set of constraints. The objective function represents the investor’s goal, such as maximizing expected return or minimizing portfolio variance (risk). Constraints represent limitations or restrictions on the portfolio, such as budget constraints (the total investment amount), short-selling restrictions (prohibiting borrowing assets to sell), or asset allocation targets (minimum or maximum weights for specific asset classes). The solution to the optimization problem is a set of asset weights that optimizes the objective function while satisfying all constraints. A common example is the Markowitz mean-variance optimization model, which aims to minimize portfolio variance for a given level of expected return. This is often represented mathematically as a quadratic programming problem.
Optimization Algorithms
Several optimization algorithms are employed to solve the mathematical problems arising from portfolio optimization models. The choice of algorithm depends on the complexity of the model and the size of the problem.
Linear programming is suitable for optimization problems where both the objective function and the constraints are linear. This is simpler to solve computationally but may not accurately reflect the complexities of real-world financial markets, where returns are often not linearly related.
Quadratic programming handles problems with a quadratic objective function and linear constraints. This is particularly relevant for mean-variance optimization, as the portfolio variance is a quadratic function of asset weights. Quadratic programming algorithms are more computationally intensive than linear programming but offer a more realistic representation of risk and return relationships. Other algorithms, such as stochastic programming and simulated annealing, are used for more complex scenarios involving uncertainty or non-convex optimization problems.
Software for Quantitative Portfolio Optimization
Several software packages facilitate quantitative portfolio optimization. These tools offer functionalities for data analysis, model building, optimization, and portfolio reporting.
Examples include:
- R: A powerful open-source statistical computing language with extensive packages for financial modeling and optimization.
- Python (with libraries like NumPy, SciPy, and CVXOPT): A versatile programming language with libraries providing tools for numerical computation and optimization.
- MATLAB: A commercial software widely used in engineering and finance, offering advanced optimization algorithms and tools for data analysis.
- Commercial portfolio management software: Many commercial platforms incorporate quantitative optimization capabilities, often integrating with data providers and trading systems.
These tools provide functionalities ranging from basic mean-variance optimization to more sophisticated techniques like robust optimization and factor-based modeling.
Implementing a Quantitative Portfolio Optimization Model
The process of implementing a quantitative portfolio optimization model involves several key steps.
First, data acquisition and preprocessing are crucial. This involves gathering historical return data for the assets under consideration, cleaning the data to handle missing values or outliers, and calculating relevant statistics such as means, variances, and covariances. Then, a suitable optimization model is selected based on the investor’s objectives and risk tolerance. The model parameters are estimated using the historical data, and the optimization problem is formulated. Next, an appropriate optimization algorithm is chosen and applied to solve the problem, yielding the optimal portfolio weights. Finally, the results are analyzed, interpreted, and used to guide investment decisions. Regular monitoring and rebalancing are essential to maintain the portfolio’s alignment with the optimization model and changing market conditions. The process often involves iterative refinement, where the model is adjusted and recalibrated based on new data and performance evaluation.
Behavioral Finance and Portfolio Optimization
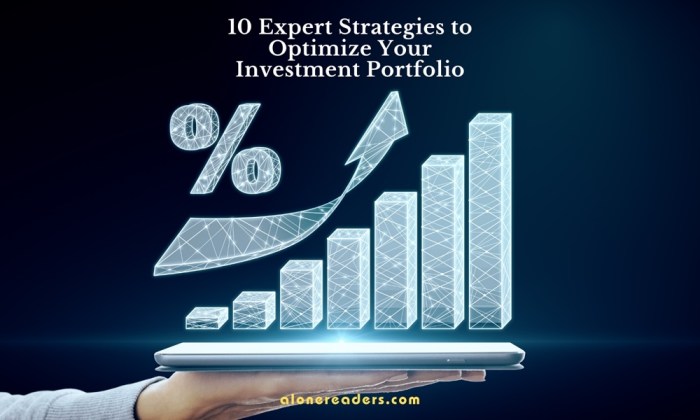
Behavioral finance recognizes that human emotions and cognitive biases significantly influence investment decisions, often leading to suboptimal portfolio performance. Unlike traditional finance which assumes perfect rationality, behavioral finance acknowledges the impact of psychological factors on investor behavior, providing a more realistic framework for portfolio optimization. This understanding allows for the development of strategies that account for these biases and improve investment outcomes.
Impact of Behavioral Biases on Investment Decisions
Numerous cognitive biases affect investment choices. For example, overconfidence can lead investors to overestimate their abilities and take on excessive risk. Loss aversion, the tendency to feel the pain of a loss more strongly than the pleasure of an equivalent gain, can cause investors to hold onto losing investments for too long, hoping for a recovery, while selling winning investments too early to lock in profits. Herding behavior, the tendency to mimic the actions of others, can lead to buying high and selling low. Confirmation bias, the tendency to seek out information that confirms pre-existing beliefs and ignore contradictory evidence, reinforces poor investment decisions. These biases, among others, can lead to poor portfolio construction and ultimately reduced returns.
Strategies to Mitigate the Effects of Behavioral Biases
Several strategies can help mitigate the negative impact of behavioral biases. One effective approach is to develop a well-defined investment plan and stick to it. This reduces the likelihood of making impulsive decisions based on emotions or short-term market fluctuations. Regular portfolio reviews, conducted without emotional involvement, can help identify and correct deviations from the plan. Diversification across asset classes is crucial to reduce the impact of individual investment losses. Seeking professional financial advice can provide an objective perspective and help investors avoid emotional decision-making. Furthermore, understanding the various cognitive biases and their potential impact on investment decisions can help investors become more aware of their own vulnerabilities and make more rational choices.
Examples of Behavioral Finance Improving Portfolio Optimization
Consider an investor exhibiting loss aversion. A behavioral finance approach would recognize this bias and recommend strategies to mitigate its impact. Instead of holding onto losing investments indefinitely, the investor might be advised to rebalance their portfolio, selling some underperforming assets and reinvesting in better-performing ones. This disciplined approach helps avoid the emotional attachment that often prevents rational decision-making. Similarly, an investor prone to overconfidence might benefit from strategies that encourage diversification and risk management, limiting their exposure to individual securities or overly concentrated positions. By acknowledging and addressing these behavioral tendencies, portfolio optimization becomes more effective and aligned with the investor’s long-term goals.
Comparison of Rational and Irrational Investment Behaviors
Characteristic | Rational Investor | Irrational Investor |
---|---|---|
Decision-Making | Based on objective analysis and data; minimizes emotional influence. | Influenced by emotions (fear, greed), biases (overconfidence, herd mentality), and heuristics (mental shortcuts). |
Risk Management | Diversifies portfolio; understands and accepts appropriate levels of risk. | Underestimates or overestimates risk; takes excessive or insufficient risk; avoids diversification. |
Investment Strategy | Develops a long-term investment plan and adheres to it; rebalances regularly. | Makes frequent trades based on short-term market fluctuations; chases performance; reacts emotionally to market news. |
Information Processing | Seeks diverse information sources; critically evaluates information; avoids confirmation bias. | Selectively seeks information confirming existing beliefs; ignores contradictory evidence; susceptible to misinformation. |
ESG Investing and Portfolio Optimization
ESG investing, which stands for Environmental, Social, and Governance, is an investment approach that considers environmental, social, and governance factors alongside traditional financial metrics when making investment decisions. This approach aims to generate positive social and environmental impact while also pursuing financial returns. By integrating ESG factors, investors can potentially mitigate risks, enhance long-term value creation, and align their portfolios with their values.
ESG factors can be integrated into portfolio optimization in several ways. One common method involves incorporating ESG scores or ratings into existing portfolio optimization models. These scores, provided by various ESG rating agencies, quantify a company’s performance across environmental, social, and governance dimensions. These scores can then be used as constraints or objectives within optimization models, allowing investors to tailor their portfolios to specific ESG preferences while still aiming for optimal risk-adjusted returns. Another method involves using ESG data to refine risk assessments, identifying potential risks and opportunities related to environmental and social issues. For example, a company with a poor environmental record might be perceived as having higher risk due to potential regulatory changes or reputational damage.
ESG Investment Strategies
Several strategies exist for integrating ESG considerations into investment portfolios. These strategies range from negative screening, where companies with poor ESG performance are excluded, to positive screening, where companies with strong ESG profiles are selected. Some investors engage in active ownership, using their shareholder influence to promote positive ESG change within companies. Impact investing focuses on investments that explicitly aim to generate measurable social and environmental impact alongside financial returns. Finally, thematic investing targets specific ESG themes, such as renewable energy or sustainable agriculture.
Constructing an ESG-Optimized Portfolio
Constructing an ESG-optimized portfolio involves a multi-step process. First, investors must define their ESG objectives and constraints. This might involve setting minimum ESG scores for companies to be included in the portfolio or specifying targets for carbon emissions reduction. Next, a suitable portfolio optimization model is selected. This model will incorporate ESG data alongside traditional financial data such as expected returns and correlations. The model is then used to optimize the portfolio, balancing risk and return objectives with ESG considerations. Finally, the portfolio is implemented and monitored, with regular rebalancing to maintain alignment with the investor’s ESG objectives and market conditions. For example, an investor aiming to reduce their carbon footprint might use a portfolio optimization model to minimize the carbon intensity of their holdings, potentially leading to a portfolio heavily weighted toward renewable energy companies and those with strong energy efficiency initiatives. Another investor might focus on social impact, leading to a portfolio emphasizing companies with strong labor practices and community engagement programs. The specific composition of the portfolio will depend on the investor’s chosen ESG objectives and risk tolerance.
Conclusion
Ultimately, effective investment portfolio optimization is an iterative process, requiring continuous monitoring, rebalancing, and adaptation to changing market conditions and personal circumstances. By understanding the principles of MPT, PMPT, factor investing, and incorporating risk management strategies, alongside awareness of behavioral biases and ESG factors, investors can build robust and resilient portfolios aligned with their individual financial objectives. The techniques explored here provide a framework for informed decision-making, empowering individuals to take control of their financial futures.
FAQs
What is the difference between active and passive portfolio management?
Active management involves actively trading securities to outperform a benchmark, while passive management aims to match a benchmark’s performance with minimal trading.
How often should I rebalance my portfolio?
Rebalancing frequency depends on your investment strategy and risk tolerance; common approaches include annual, semi-annual, or quarterly rebalancing.
What are some common software tools for portfolio optimization?
Popular options include specialized financial software packages and programming languages like Python with relevant libraries (e.g., NumPy, SciPy).
How can I incorporate ESG factors into my portfolio?
You can invest in ESG-focused funds, screen companies based on ESG criteria, or engage with companies on ESG issues.