Investment Portfolio Optimization Techniques are not just for Wall Street wizards; they’re the secret sauce to making your money work smarter, not harder. This exploration delves into the fascinating world of maximizing returns while minimizing risk, from the classic Modern Portfolio Theory (MPT) to the more nuanced approaches of Post-Modern Portfolio Theory (PMPT) and factor-based investing. We’ll unravel the complexities of risk management, portfolio rebalancing, and even the role of investor psychology in shaping optimal investment strategies. Get ready to ditch the guesswork and embrace the power of data-driven decision-making in your investment journey!
We’ll cover a range of techniques, including mean-variance optimization, the Black-Litterman model, and risk parity, providing practical examples and clear explanations to demystify these often-intimidating concepts. Whether you’re a seasoned investor or just starting out, this guide offers a comprehensive overview of the tools and strategies needed to build a portfolio that aligns with your financial goals and risk tolerance. Prepare to become a more informed and confident investor.
Introduction to Investment Portfolio Optimization
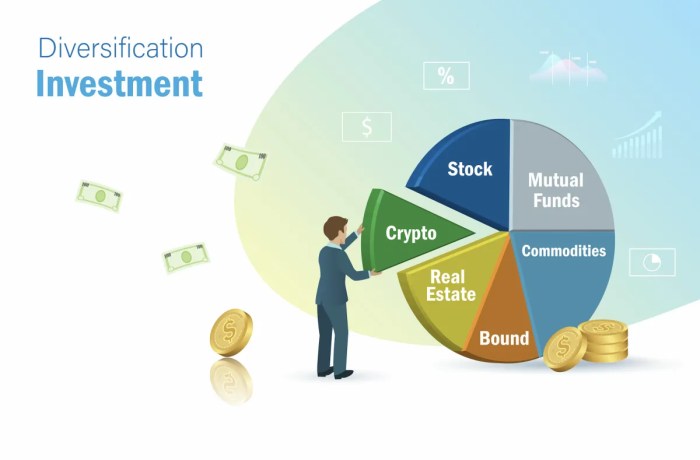
Investing wisely isn’t just about picking winning stocks; it’s about crafting a portfolio that serenades your financial goals while simultaneously performing a dazzling dance of risk management. Portfolio optimization is the conductor of this financial orchestra, aiming for the sweetest possible melody of returns with the least jarring dissonance of risk. Think of it as the art of strategic asset allocation, maximizing potential gains while minimizing the chances of a catastrophic market meltdown leaving you singing the blues.
Portfolio optimization hinges on several core principles. Firstly, it acknowledges that the world of investment is inherently uncertain; we can’t predict the future with the precision of a Swiss watchmaker. Therefore, the goal isn’t to guarantee riches (unless you’ve discovered a time machine, in which case, please share!), but to strategically manage risk and maximize expected returns given the inherent uncertainty. Secondly, the process involves a deep understanding of your risk tolerance and investment horizon. Are you a thrill-seeking daredevil or a cautious tortoise? Are you saving for retirement in 30 years or a down payment next year? These factors significantly influence the optimal asset allocation. Finally, portfolio optimization utilizes mathematical models and statistical analysis to make informed decisions, transforming the somewhat subjective world of investment into a more data-driven and objective arena.
Diversification: The Unsung Hero of Portfolio Construction
Diversification, the cornerstone of any successful investment strategy, is the practice of spreading your investments across various asset classes (stocks, bonds, real estate, etc.) and sectors (technology, healthcare, energy, etc.). It’s the financial equivalent of not putting all your eggs in one basket – a lesson even your grandma likely taught you. The rationale behind diversification is simple yet profound: by spreading your risk across different assets, you reduce the impact of any single investment performing poorly. If one sector takes a dive, the others might be soaring, cushioning the blow and preventing a complete portfolio collapse. Imagine a portfolio entirely invested in a single company; if that company fails, your entire investment evaporates. Diversification, however, acts as a safety net, reducing volatility and improving the overall risk-adjusted return of the portfolio. A diversified portfolio isn’t guaranteed to outperform a concentrated one, but it significantly reduces the chance of a catastrophic loss. This is why even seasoned investors embrace diversification as a crucial component of their investment strategy.
Historical Overview of Portfolio Optimization Techniques
The quest for the perfect portfolio optimization technique has been a fascinating journey through history. Early approaches, often rooted in intuition and basic rules of thumb, gradually gave way to more sophisticated mathematical models. The seminal work of Harry Markowitz in the 1950s, culminating in his Modern Portfolio Theory (MPT), revolutionized the field. MPT introduced the concept of efficient frontiers – a graphical representation showing the optimal balance between risk and return. It demonstrated that it’s possible to achieve higher returns for a given level of risk, or lower risk for a given level of return, by carefully diversifying across assets. Subsequent developments, such as the Capital Asset Pricing Model (CAPM) and Black-Litterman model, further refined these techniques, incorporating factors like market risk and investor views. While MPT and its descendants have been instrumental in shaping modern portfolio management, it’s crucial to remember that these models rely on assumptions that may not always hold true in the real world, particularly during periods of market turmoil. The ongoing evolution of portfolio optimization techniques reflects the constant search for better ways to navigate the complex and ever-changing landscape of financial markets. It’s a continuous process of refinement, driven by the eternal quest for higher returns with lower risk – a quest as old as investing itself.
Modern Portfolio Theory (MPT)
Modern Portfolio Theory, or MPT, is like the Swiss Army knife of investment strategies – deceptively simple on the surface, yet surprisingly powerful (and occasionally prone to unexpected blade closures). It offers a framework for constructing investment portfolios that maximize returns for a given level of risk, or minimize risk for a given level of return. Sounds fantastic, right? Well, buckle up, because the reality is slightly less utopian.
Assumptions and Limitations of MPT
MPT rests on several key assumptions, some of which are, shall we say, optimistic in their portrayal of the real world. For example, it assumes investors are rational, risk-averse utility maximizers. This conveniently ignores the human element – our tendency towards panic selling, herd behavior, and the occasional bout of irrational exuberance. Further, it assumes that asset returns follow a normal distribution. While a handy simplification, real-world returns often exhibit “fat tails,” meaning extreme events occur more frequently than the normal distribution predicts. This is why MPT sometimes struggles to capture market crashes and black swan events accurately. Finally, MPT assumes that investors can borrow and lend at the risk-free rate, a rate that, in reality, can be elusive and may vary considerably depending on creditworthiness.
The Efficient Frontier: A Graphical Representation of Optimal Portfolios
The efficient frontier is the heart of MPT. It’s a graphical representation of the set of optimal portfolios that offer the highest expected return for a defined level of risk. Imagine a curve plotted on a graph with risk (usually measured by standard deviation) on the x-axis and return (usually measured by expected return) on the y-axis. The efficient frontier is the upper portion of this curve – any portfolio lying below the curve is considered inefficient because it offers either lower returns for the same level of risk or higher risk for the same level of return. Portfolios on the efficient frontier represent the sweet spot – the optimal balance between risk and reward. Finding the optimal portfolio on this frontier is the ultimate goal of MPT.
Calculating Portfolio Variance and Covariance
Calculating portfolio variance and covariance is crucial for constructing the efficient frontier. Portfolio variance measures the overall risk of the portfolio, while covariance measures how the returns of different assets move together.
Portfolio Variance: σp2 = w12σ12 + w22σ22 + 2w1w2Cov(1,2)
Where:
* σp2 is the portfolio variance
* w1 and w2 are the weights of assets 1 and 2 in the portfolio
* σ12 and σ22 are the variances of assets 1 and 2
* Cov(1,2) is the covariance between assets 1 and 2
Covariance: Cov(1,2) = ρ1,2σ1σ2
Where:
* ρ1,2 is the correlation coefficient between assets 1 and 2
* σ1 and σ2 are the standard deviations of assets 1 and 2
Essentially, you need to know the risk of each individual asset, how much of each asset is in your portfolio, and how the returns of those assets move together. It’s a bit like a complex dance, but with numbers.
Risk-Return Profiles Using MPT
The following table demonstrates how different risk-return profiles can be achieved using MPT. Note that these are illustrative examples and actual results may vary considerably.
Portfolio | Expected Return (%) | Standard Deviation (%) | Sharpe Ratio |
---|---|---|---|
Conservative | 5 | 5 | 0.5 |
Moderate | 10 | 10 | 0.8 |
Aggressive | 15 | 15 | 0.9 |
Highly Aggressive | 20 | 25 | 0.7 |
The Sharpe Ratio (a measure of risk-adjusted return) helps compare portfolios with different levels of risk. A higher Sharpe ratio indicates a better risk-adjusted return. As you can see, while higher returns are associated with higher risk, optimizing the portfolio using MPT allows for a more efficient risk-return profile.
Mean-Variance Optimization
Mean-variance optimization, darling, is the sophisticated art of building investment portfolios that maximize expected returns while minimizing the volatility – the stomach-churning ups and downs – that comes with the territory. It’s like a high-stakes game of balancing act, where the goal is to achieve the highest possible return without throwing up your breakfast. Think of it as the Goldilocks of portfolio management: not too risky, not too boring, just right.
The core of this method lies in a simple (ish) equation that considers the expected return of each asset, their individual volatilities (risks), and the correlations between them. This allows us to craft a portfolio that optimally balances risk and reward. It’s not magic, but it’s close.
The Mean-Variance Optimization Process, Investment Portfolio Optimization Techniques
Mean-variance optimization involves calculating the optimal weights for each asset in a portfolio to achieve a desired level of risk and return. This typically involves using matrix algebra and optimization algorithms, which, let’s be honest, sounds scarier than it is. In essence, the process systematically searches for the best combination of assets to reach your financial goals, accounting for the inherent trade-off between risk and return. The process involves defining the investment universe, estimating expected returns and variances, and then using optimization techniques to determine the ideal asset allocation.
The Role of Risk Aversion in Portfolio Construction
Risk aversion is the crucial spice that flavors our portfolio recipe. It measures how much an investor dislikes risk. A highly risk-averse investor will prioritize capital preservation over high returns, opting for a portfolio heavily weighted towards low-risk assets like government bonds. Conversely, a risk-seeking investor might happily gamble a larger portion of their portfolio on high-growth, high-risk assets like penny stocks (proceed with caution!). Mean-variance optimization allows us to tailor the portfolio’s risk profile to match the investor’s unique risk tolerance, ensuring a comfortable and potentially profitable journey. This is often incorporated through a parameter called the risk aversion coefficient, which directly influences the optimal portfolio weights.
Example Portfolio Using Mean-Variance Optimization
Let’s concoct a hypothetical portfolio using mean-variance optimization. Imagine we have three assets: a low-risk government bond (GB), a moderately risky stock index (SI), and a high-risk tech stock (TS). We’ll use some made-up (but plausible) data for simplicity:
Asset | Expected Return | Standard Deviation | Correlation with GB | Correlation with SI |
---|---|---|---|---|
GB | 3% | 2% | 1.0 | 0.2 |
SI | 8% | 10% | 0.2 | 1.0 |
TS | 15% | 20% | 0.1 | 0.5 |
Using optimization software (or even a sophisticated spreadsheet), we can input this data and specify a desired level of risk aversion. The output would be the optimal weights for each asset in the portfolio that maximizes expected return given the specified risk tolerance. For example, a risk-averse investor might receive a portfolio allocation of 60% GB, 30% SI, and 10% TS, while a less risk-averse investor might get something like 20% GB, 40% SI, and 40% TS. The exact weights will depend on the chosen risk aversion level and the correlations between the assets.
Implementing Mean-Variance Optimization in a Spreadsheet
Implementing mean-variance optimization in a spreadsheet requires a decent level of comfort with matrix algebra and solver functions. However, the general steps are as follows:
First, you’ll need to input the expected returns and standard deviations of each asset. Then, calculate the covariance matrix, which reflects the relationships between asset returns. This is where the correlations come into play. Finally, use the spreadsheet’s solver function (often found under “Data” -> “Solver”) to find the optimal portfolio weights that maximize the Sharpe Ratio (a measure of risk-adjusted return) or minimize portfolio variance for a given expected return, based on the chosen risk aversion. Remember, this process requires accurate data and a thorough understanding of the underlying principles; otherwise, you might end up with a portfolio that’s less than optimal – or even disastrous.
Post-Modern Portfolio Theory (PMPT)
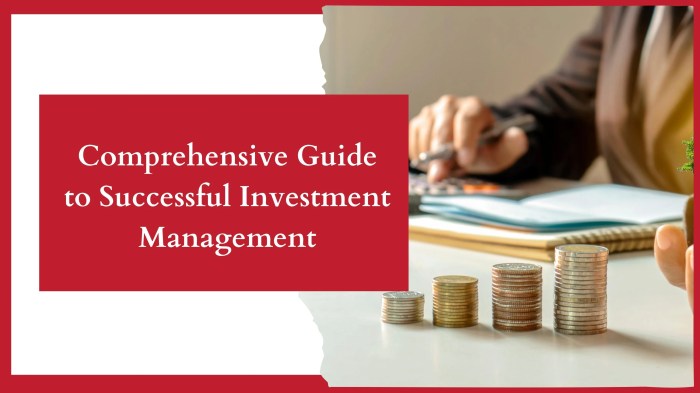
Ah, Post-Modern Portfolio Theory (PMPT)! If Modern Portfolio Theory (MPT) was the disco era of investment strategies, then PMPT is the sophisticated, slightly cynical, but ultimately more resilient house music scene. While MPT confidently struts its stuff with its elegant (if somewhat naive) assumptions, PMPT acknowledges the messy realities of the dance floor – namely, that returns aren’t always normally distributed and that focusing solely on the upside ignores the potential for a spectacular face-plant.
PMPT offers a more nuanced approach to portfolio construction, acknowledging the limitations of MPT’s reliance on solely mean and variance. It’s like moving from a simple two-step to a complex, multi-layered dance routine that accounts for unexpected dips and sudden changes in rhythm. Instead of solely focusing on maximizing returns while minimizing variance (standard deviation), PMPT prioritizes downside risk management and tail risk mitigation. This approach recognizes that investors are often more concerned about the potential for significant losses than they are about missing out on slightly higher gains.
Comparison of MPT and PMPT Approaches to Risk Management
MPT’s risk management strategy centers around minimizing variance, which is a measure of overall volatility, both upside and downside. This assumes a symmetrical distribution of returns, a simplification that often doesn’t hold true in the real world. Imagine a perfectly symmetrical bell curve – MPT loves this. PMPT, however, sees the real world as a much more lopsided affair. It recognizes that investors are typically far more concerned with the downside (the left tail of the distribution) than the upside. PMPT shifts the focus to managing downside risk, specifically focusing on the probability and magnitude of losses.
PMPT’s Address of MPT Limitations
One of MPT’s major limitations is its reliance on the assumption of normally distributed returns. Real-world asset returns often exhibit fat tails – meaning there’s a higher probability of extreme events (both positive and negative) than a normal distribution predicts. Think of the 2008 financial crisis – a clear example of a “fat tail” event that MPT’s models struggled to anticipate. PMPT addresses this by using alternative distributions that better capture the leptokurtic (fat-tailed) nature of asset returns. It’s like recognizing that sometimes, the dance floor gets unexpectedly crowded, leading to unexpected stumbles.
Another weakness of MPT is its sensitivity to input parameters (like expected returns and covariances), which are often estimated with significant uncertainty. Small changes in these inputs can lead to dramatically different portfolio allocations. PMPT tackles this by incorporating robust optimization techniques that are less sensitive to estimation errors. It’s like having a backup dancer ready to step in if the lead dancer trips.
Downside Risk Measures in PMPT
PMPT employs various measures to quantify and manage downside risk. Instead of the all-encompassing variance, it focuses on metrics that specifically capture the potential for losses.
Semivariance: This measures the dispersion of returns below the mean, focusing solely on downside deviations. It ignores positive deviations, reflecting the investor’s greater concern about losses.
Value at Risk (VaR): This statistical measure quantifies the potential loss in value of an asset or portfolio over a specific time period and confidence level. For example, a 95% VaR of $1 million means there’s a 5% chance of losing at least $1 million.
Conditional Value at Risk (CVaR), also known as Expected Shortfall (ES): This goes beyond VaR by calculating the expected loss in the worst cases. Instead of just identifying the threshold loss, it calculates the average loss beyond that threshold. So, it tells you not just what the worst-case scenario *might* be, but also how bad it *could* be on average.
These measures provide a more comprehensive picture of downside risk than the simple variance used in MPT. They are like having a detailed floor plan of the dance floor, highlighting potential hazards and allowing for a more strategic dance.
Factor-Based Investing
Factor-based investing, darling of the quantitative finance world, moves beyond simply picking stocks based on gut feeling or a whispered tip from your uncle. Instead, it leverages the power of statistical models to identify and exploit systematic patterns in market returns. Think of it as the sophisticated, data-driven cousin of traditional stock picking – far less reliant on caffeine-fueled hunches.
Factor models provide a framework for understanding these patterns, suggesting that certain characteristics – or “factors” – are associated with higher returns over the long run. These factors aren’t just random; they often reflect fundamental economic principles or market inefficiencies. The beauty? By strategically constructing portfolios based on these factors, investors can potentially achieve superior risk-adjusted returns. It’s like having a secret decoder ring for the market’s hidden messages, except the ring is made of algorithms and spreadsheets.
Factor Model Roles in Portfolio Optimization
Factor models serve as the backbone of factor-based portfolio optimization. They provide a structured way to identify and quantify the relationships between various factors and asset returns. This allows investors to build portfolios that are explicitly exposed to desired factors, potentially enhancing returns and controlling risk. Essentially, it’s like building with LEGOs, where each brick represents a factor and you get to choose which ones to use and how to arrange them to create the portfolio of your dreams (or at least, a portfolio with a decent Sharpe ratio). This structured approach differs significantly from traditional methods that may focus solely on individual asset selection, often ignoring the broader market context and factor exposures.
Identifying and Selecting Relevant Factors
The selection of relevant factors is crucial, and not just because choosing the wrong ones could lead to a portfolio that performs worse than a dart-throwing chimpanzee. A variety of factors exist, each with its own characteristics and potential benefits. Some common factors include value (low price-to-book ratio), size (market capitalization), momentum (recent price performance), quality (profitability and financial strength), and volatility (price fluctuations). The selection process often involves a combination of academic research, empirical analysis, and consideration of the investor’s specific objectives and risk tolerance. It’s a bit like choosing your ingredients for a gourmet dish; each factor adds a unique flavor, and the right combination can create something truly exceptional (or a culinary disaster, if you’re not careful).
Constructing a Factor-Based Portfolio
Let’s imagine a simplified scenario with three factors: Value (V), Size (S), and Momentum (M). We’ll use hypothetical factor returns for a given period: V = 10%, S = 8%, M = 5%. Suppose we want a portfolio with a 60% allocation to Value, 30% to Size, and 10% to Momentum. The portfolio return would be calculated as: (0.6 * 10%) + (0.3 * 8%) + (0.1 * 5%) = 8.3%. This is a highly simplified example, of course. In reality, constructing a factor-based portfolio involves sophisticated optimization techniques to manage risk, diversification, and transaction costs. It’s less “mix and match” and more “high-frequency trading algorithm meticulously calculating optimal factor weights.”
Advantages and Disadvantages of Factor-Based Investing
Before diving in headfirst, it’s wise to consider the pros and cons.
- Advantages:
- Potentially higher risk-adjusted returns compared to traditional market-cap weighted strategies.
- Improved diversification by exploiting different sources of return.
- More transparent and systematic approach to portfolio construction.
- Potential for enhanced risk management through factor exposure control.
- Disadvantages:
- Factor premiums can be unpredictable and may not always persist.
- Requires sophisticated data and analytical capabilities.
- Potential for higher transaction costs due to frequent rebalancing.
- Exposure to factor risks, such as a sudden shift in market preferences.
Black-Litterman Model
The Black-Litterman model, a darling of sophisticated investors, elegantly addresses a common frustration in portfolio optimization: the inherent uncertainty of market expectations. Unlike simpler models that rely solely on historical data, Black-Litterman allows investors to inject their own informed opinions – their “views” – into the optimization process, resulting in a portfolio more aligned with their individual risk tolerance and market outlook. It’s like adding a dash of personal flair to a perfectly good recipe, resulting in a culinary masterpiece (or at least, a better-performing portfolio).
The Black-Litterman model cleverly combines the equilibrium returns from the Capital Asset Pricing Model (CAPM) or a similar market model with the investor’s subjective views. It does this using Bayesian statistics, a fancy way of saying it updates prior beliefs (the market equilibrium returns) with new information (the investor’s views). This results in posterior estimates of expected returns, which are then used in a standard mean-variance optimization framework to generate a portfolio allocation. The brilliance lies in its ability to gracefully handle conflicting information, blending objective market data with subjective investor opinions.
Incorporating Investor Views
Investor views are expressed as specific statements about the relative performance of assets. These views aren’t just gut feelings; they should be based on fundamental analysis, macroeconomic forecasts, or other credible sources. For example, an investor might believe that the technology sector will outperform the energy sector over the next year. This view is quantified using a matrix that specifies the relative expected return differences between assets, along with a measure of confidence in the view. The higher the confidence, the stronger the influence of the investor’s view on the final portfolio allocation. The model cleverly weighs these views against the market equilibrium returns, preventing overly aggressive or unrealistic portfolio adjustments based on potentially flawed predictions.
Example of Investor View Influence
Let’s say a portfolio manager believes that Company A will outperform Company B by 5% over the next year. Without incorporating this view, a standard mean-variance optimization might allocate a similar weight to both companies based solely on their historical risk and return characteristics. However, by inputting this view into the Black-Litterman model (along with a confidence level reflecting the strength of the belief), the model will adjust the portfolio allocation to favor Company A, potentially shifting a larger portion of the portfolio to Company A’s stock compared to Company B’s. The magnitude of this shift is directly proportional to the confidence level assigned to the view; a highly confident view will lead to a more significant reallocation.
Implementing the Black-Litterman Model: A Step-by-Step Guide
Implementing the Black-Litterman model requires a structured approach.
- Estimate Market Equilibrium Returns: This is typically done using a model like the CAPM, generating expected returns for each asset based on market factors.
- Construct the Covariance Matrix: This matrix quantifies the relationships between the returns of different assets, reflecting their historical volatility and correlations. This is crucial for risk management within the model.
- Specify Investor Views: Articulate specific views on relative asset performance, quantifying them with numerical values and confidence levels. This is the heart of the Black-Litterman process, injecting investor-specific insights.
- Combine Market Equilibrium and Investor Views: Using Bayesian techniques, the model blends the market equilibrium returns with the investor’s views, adjusting the expected returns based on the confidence assigned to each view. This step is mathematically complex but is readily implemented using specialized software.
- Perform Mean-Variance Optimization: Using the adjusted expected returns and the covariance matrix, a mean-variance optimization is performed to determine the optimal portfolio weights. This final step ensures the portfolio is well-diversified and aligned with the investor’s risk profile and views.
The Black-Litterman model is not a magic bullet; its success hinges on the quality of both the market equilibrium estimates and, crucially, the investor’s views. Garbage in, garbage out, as they say. However, when used thoughtfully and with well-researched views, it can provide a powerful tool for tailoring portfolios to individual investor needs and beliefs.
Risk Parity
Risk parity, in the whimsical world of finance, isn’t about balancing your checkbook (though that’s important too!). Instead, it’s a portfolio construction strategy that aims for a more even distribution of risk across different asset classes, rather than focusing solely on expected returns. Think of it as giving each asset a fair shot at contributing to the overall portfolio’s risk, rather than letting one asset hog the spotlight (and potentially the losses).
Risk parity portfolios allocate capital to different assets not based on their expected returns, but rather on their contribution to the overall portfolio’s risk. This is achieved by targeting equal risk contributions from each asset, which typically involves adjusting the weights based on each asset’s volatility. In simpler terms, it’s about ensuring that your exposure to risk is evenly spread, rather than concentrated in a few high-risk investments. It’s like spreading your bets across multiple tables at a casino, instead of putting all your chips on one number – a far more sensible strategy, wouldn’t you agree?
Principles of Risk Parity Portfolio Construction
Risk parity’s core principle is to allocate assets such that each contributes equally to the overall portfolio risk, usually measured by volatility. This differs significantly from traditional mean-variance optimization, which prioritizes maximizing returns for a given level of risk. The process typically involves several steps: estimating the covariance matrix of asset returns (a fancy way of saying how assets move together), determining the target risk contribution for each asset (usually equal), and then calculating the portfolio weights that achieve this target. This often involves iterative optimization techniques to find the optimal weights that balance risk contributions.
Advantages and Disadvantages of Risk Parity
Let’s face it, nothing in finance is perfect. Risk parity, while offering some alluring advantages, also comes with its own set of drawbacks.
Advantages:
- Reduced Portfolio Volatility: By diversifying risk across assets, risk parity portfolios can exhibit lower overall volatility compared to traditional strategies heavily weighted towards high-return, high-risk assets.
- Improved Risk-Adjusted Returns: In many historical scenarios, risk parity has demonstrated competitive risk-adjusted returns, particularly during periods of market stress.
- More Robust Diversification: The focus on risk contribution rather than return expectation leads to a more robust and potentially less correlated portfolio.
Disadvantages:
- Lower Expected Returns (Potentially): Because it prioritizes risk balance, risk parity may not always maximize expected returns, especially in bull markets where high-risk assets outperform.
- Sensitivity to Risk Measures: The effectiveness of risk parity depends heavily on the accuracy of the chosen risk measure (typically volatility). Inaccurate risk estimates can lead to suboptimal portfolio performance.
- Complexity: Implementing and managing a risk parity portfolio requires sophisticated techniques and may be more complex than traditional portfolio approaches.
Example of a Risk Parity Portfolio Allocation
Imagine a portfolio consisting of three asset classes: U.S. Equities, U.S. Government Bonds, and Emerging Market Equities. Let’s assume, for simplicity, that their annualized volatilities are 15%, 5%, and 20%, respectively. A risk parity approach would aim for equal risk contributions from each asset. This wouldn’t mean a 33.33% allocation to each; instead, the weights would be adjusted to account for the differing volatilities. The higher volatility of emerging market equities would lead to a lower allocation compared to U.S. Equities, which has moderate volatility. A possible (and simplified) risk parity allocation could be 40% U.S. Equities, 60% U.S. Government Bonds, and 20% Emerging Market Equities. The exact weights would be determined by more sophisticated optimization techniques. Note: This is a simplified illustration and actual risk parity allocations are more complex.
Visual Representation of a Risk Parity Portfolio
Imagine a pie chart. This pie represents the total portfolio risk. Instead of slices representing asset allocation percentages, each slice represents the percentage contribution to the total portfolio risk. In a risk parity portfolio, each slice would be roughly equal in size, demonstrating the equal risk contribution from each asset class. For instance, if we have three asset classes (A, B, C), each would ideally occupy approximately one-third of the pie, even if their weights in terms of capital allocation are significantly different. This visual clearly demonstrates the core principle of risk parity: equal risk contribution, not necessarily equal capital allocation. The size of each slice reflects the risk contribution, not the percentage of the investment.
Tactical Asset Allocation
Tactical asset allocation (TAA) is the exciting, rollercoaster-like cousin of strategic asset allocation. While strategic allocation sets a long-term course, TAA is all about making short-term adjustments based on market forecasts – a bit like trying to time the perfect wave for a lucrative surfing session. It involves actively shifting asset weights in response to perceived market opportunities and risks, aiming to outperform a static portfolio. Think of it as a dynamic dance with the market, full of potential for both profit and peril.
TAA strategies employ a variety of techniques to identify potentially favorable market conditions and capitalize on them. This isn’t about crystal balls and tea leaves, though; it’s about analyzing market data and indicators to make informed decisions. The core idea is to adjust portfolio weights to take advantage of short-term market trends, with the expectation that these adjustments will generate higher returns than a passively managed portfolio over time. Of course, it’s not always a smooth ride.
Market Timing in Tactical Asset Allocation
Market timing, a key component of TAA, involves attempting to predict future market movements. Successful market timing relies on identifying shifts in economic trends, investor sentiment, and other factors that might foreshadow market direction. This is where the art meets the science of investing – it’s about interpreting data and making bold (but hopefully well-informed) calls. It’s worth noting that consistently successful market timing is notoriously difficult, even for seasoned professionals. Many attempts fail, emphasizing the need for rigorous analysis and risk management. Imagine trying to consistently predict the price of Bitcoin – it’s a volatile beast!
Risks Associated with Tactical Asset Allocation
TAA, while potentially lucrative, comes with its fair share of risks. The most significant is the risk of *inaccurate market forecasts*. If your predictions are wrong, you could find yourself underwater, having made asset allocation shifts that hurt your portfolio rather than helping it. Another significant risk is the *transaction costs* associated with frequent trading. Buying and selling assets frequently can eat into your profits, especially if your timing isn’t perfect. Finally, there’s the ever-present risk of *missed opportunities* – sometimes, sitting tight and letting the market do its thing can yield better results than attempting to time the market perfectly.
Market Indicators Used in Tactical Asset Allocation
Several market indicators help inform TAA decisions. These aren’t foolproof, but they provide valuable data points for analysis. For example, the *price-to-earnings ratio (P/E)* can signal whether the market is overvalued or undervalued. A high P/E ratio might suggest it’s time to reduce equity exposure. Similarly, the *yield curve* – the difference in yields between short-term and long-term government bonds – can provide insights into future economic growth. An inverted yield curve (short-term yields higher than long-term yields) is often seen as a recessionary predictor. Other indicators include *economic growth rates*, *inflation rates*, *consumer confidence indices*, and *volatility indices* like the VIX. These indicators, when analyzed in conjunction with each other, can offer a more comprehensive view of market conditions and inform tactical allocation decisions.
Portfolio Rebalancing Strategies
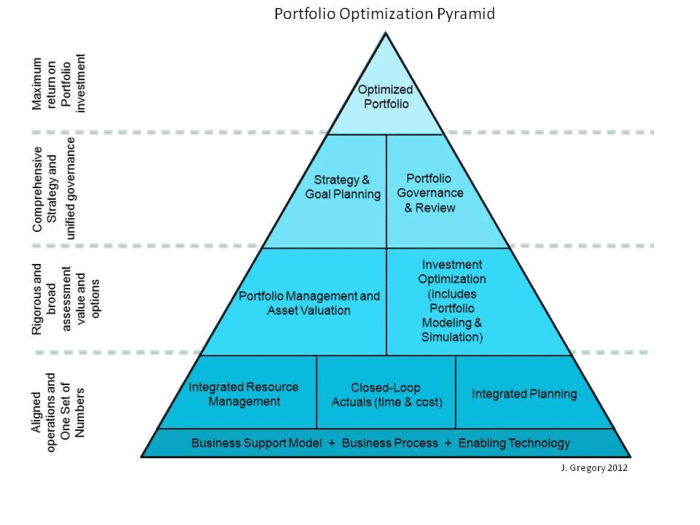
Rebalancing your investment portfolio might sound like a chore, akin to alphabetizing your spice rack, but it’s actually a crucial strategy to maintain your desired asset allocation and potentially boost returns. Think of it as a financial spring cleaning – getting rid of the dust (underperforming assets) and making room for new growth. Ignoring it is like letting your spice rack descend into utter chaos – eventually, you won’t even know what you have!
Rebalancing involves adjusting your portfolio’s asset allocation back to your target percentages after market fluctuations. This systematic approach helps you capitalize on market dips and avoid overexposure to any single asset class that’s experienced a surge. Different approaches exist, each with its own set of advantages and disadvantages, and the best choice depends on your investment goals, risk tolerance, and the dreaded transaction costs.
Time-Based Rebalancing
Time-based rebalancing involves adjusting your portfolio at predetermined intervals, regardless of market performance. This could be annually, semi-annually, or even quarterly. The simplicity of this approach is its main appeal. It’s like setting a calendar reminder for your financial health check-up. However, it can lead to more frequent trading, potentially increasing transaction costs, especially if market movements are minimal. For example, a portfolio rebalanced annually might involve selling some winners and buying some losers even if their price changes are small.
Level-Based Rebalancing
Level-based rebalancing, on the other hand, triggers a rebalance only when the deviation from your target allocation exceeds a predefined threshold. This strategy is more reactive than time-based rebalancing, and it minimizes transaction costs by only rebalancing when necessary. Imagine your target allocation is 60% stocks and 40% bonds. A level-based strategy might only trigger a rebalance if the stock allocation drifts beyond, say, 65% or below 55%. This approach reduces trading frequency and thus minimizes expenses. However, it might mean missing opportunities to capitalize on significant market swings if the deviation threshold is set too high.
Impact of Transaction Costs on Rebalancing Frequency
Transaction costs, including brokerage commissions and bid-ask spreads, are a significant factor in determining the optimal rebalancing frequency. High transaction costs can erode returns, especially for frequent rebalancing. The sweet spot lies in finding a balance between maintaining the desired asset allocation and minimizing trading expenses. A high-frequency rebalancing strategy might be suitable for a high-net-worth investor with low transaction costs, but for someone with a smaller portfolio, a less frequent approach might be more appropriate. It’s a delicate balancing act, much like juggling chainsaws (not recommended).
Hypothetical Scenario: The Benefits of Rebalancing
Let’s imagine a portfolio initially allocated 50% to stocks and 50% to bonds. Over a year, stocks outperform bonds significantly, resulting in a portfolio composition of 65% stocks and 35% bonds. A rebalancing strategy would involve selling 15% of the stock holdings and buying 15% of bonds, bringing the allocation back to the 50/50 target. Without rebalancing, the investor would have become significantly more exposed to stocks, increasing risk. Had the market taken a downturn in the following year, the unrebalanced portfolio would have suffered greater losses. Rebalancing, therefore, acted as a built-in risk management tool, ensuring the portfolio remained aligned with the investor’s risk tolerance. The disciplined investor, in this scenario, enjoys a more serene night’s sleep.
Software and Tools for Portfolio Optimization
The world of portfolio optimization isn’t just spreadsheets and slide rules anymore (though, let’s be honest, some of us still fondly remember those days). Today, a plethora of sophisticated software and tools exist, each promising to unlock the secrets to financial nirvana. Choosing the right one, however, requires a discerning eye and a healthy dose of skepticism – after all, even the most advanced algorithm can’t predict the next market hiccup caused by a rogue squirrel shorting the almond market.
Software for portfolio optimization ranges from simple spreadsheet add-ins to powerful, enterprise-grade platforms. The best choice depends heavily on your specific needs, technical skills, and, of course, budget. Think of it like choosing a car – a bicycle might suffice for short trips, but a Formula 1 racecar is overkill unless you’re aiming for the podium (and have a pit crew).
Popular Software and Tools
Several software packages cater to different levels of expertise and portfolio complexity. These range from user-friendly tools ideal for individual investors to complex platforms utilized by institutional investors managing billions. Understanding the capabilities and limitations of each is crucial for making an informed decision.
Capabilities and Limitations of Different Software Options
The capabilities of portfolio optimization software vary widely. Some offer basic mean-variance optimization, while others incorporate advanced techniques like Black-Litterman or risk parity. Limitations often revolve around data quality, model assumptions, and the inherent uncertainties of future market performance. No software can perfectly predict the future, no matter how many fancy algorithms it uses. Even the most sophisticated software is only as good as the data it’s fed. Garbage in, garbage out, as the saying goes (and trust us, we’ve seen some truly garbage data).
Comparison of Software Packages
The following table compares several popular portfolio optimization software packages. Note that pricing can vary based on features and the number of users. Also, bear in mind that features and pricing are subject to change, so always check the vendor’s website for the most up-to-date information.
Software | Features | Pricing | Limitations |
---|---|---|---|
Portfolio Visualizer | Backtesting, portfolio optimization, risk analysis, various asset classes | Subscription-based, various tiers | Limited advanced optimization techniques; data limitations for some international markets |
Riskalyze | Risk tolerance assessment, portfolio construction, performance reporting | Subscription-based, tiered pricing | Primarily focused on risk management; limited advanced optimization features |
Bloomberg Terminal | Comprehensive data, analytics, and trading platform; advanced portfolio optimization tools | Expensive subscription, typically used by institutions | High cost; steep learning curve |
Axioma Portfolio Optimizer | Advanced optimization algorithms, factor models, stress testing | Enterprise-level pricing, typically for large institutions | Extremely complex; requires specialized expertise |
Final Summary: Investment Portfolio Optimization Techniques
Mastering investment portfolio optimization is a journey, not a destination. While there’s no magic bullet for guaranteed riches, understanding the principles Artikeld here empowers you to make more informed, strategic decisions about your investments. By combining theoretical frameworks with practical applications, you can craft a portfolio tailored to your unique circumstances, maximizing potential returns while mitigating unnecessary risks. Remember, consistent learning and adaptation are key to long-term success in the ever-evolving world of finance. So, go forth and optimize!
Key Questions Answered
What’s the difference between active and passive portfolio management?
Active management involves actively trying to beat the market through stock picking and market timing. Passive management aims to match the market’s return by investing in index funds or ETFs, requiring less effort and typically lower fees.
How often should I rebalance my portfolio?
Rebalancing frequency depends on your investment strategy and risk tolerance. Common approaches include annual, semi-annual, or quarterly rebalancing. More frequent rebalancing can be costly due to transaction fees.
What is the role of emotional bias in portfolio management?
Emotional biases, like fear and greed, can significantly impact investment decisions. Rational, data-driven approaches help mitigate these biases and promote long-term success.
Are there any free tools available for portfolio optimization?
While many sophisticated tools are paid, several free spreadsheet templates and online calculators offer basic portfolio optimization functionalities. However, their capabilities are often limited compared to professional software.